- Academic Editor
†These authors contributed equally.
Background: To explore the feasibility of radiomic models using
different magnetic resonance imaging (MRI) sequences combined with clinical
information in evaluating the status of lymphovascular space invasion (LVSI) in
cervical cancer. Methods: One hundred one cervical cancer patients were
included from January 2018 to December 2020. All patients underwent 3.0T MRI
examination including T2 weighted imaging (T2WI), diffusion weighted imaging
(DWI) and contrast-enhanced T1 weighted imaging (T1WI + C) enhanced sequences.
Age, preoperative squamous cell carcinoma (SCC) associated antigen value and the
depth of muscular invasion were collected. The 101 patients were divided into
training set and validation set. Three different models were developed using
T2WI, DWI and T1WI + C parameters respectively. One model was developed combining
the three different sequences. The diagnostic performance of each model was
compared via receiver operating characteristic curve analysis. Results:
Forty-eight cases were pathologically confirmed with lymphovascular space
invasion. The average SCC value of the LVSI positive group (10.82
Cervical cancer is the fourth leading cause of morbidity and mortality in women worldwide [1]. Several treatment strategies are available for cervical cancer including surgery, radiotherapy and concurrent chemoradiotherapy, depending on different clinical stages. Despite significant improvement in the development of diagnostic techniques, the long-term survival of patients with cervical cancer has not remarkably increased. Recent studies have reported that there are still overall 11%–22% and 28%–64% recurrence rate in IB–IIA and IIIB–IVA patients respectively [2, 3, 4]. This suggests that studies on prognostic factors of cervical cancer are of great importance.
Lymph node metastasis has been regarded as an independent prognostic factor in the progress of cervical cancer, while lymphovascular space invasion (LVSI) is a medium risk factor for the prognosis of cervical cancer. LVSI is a common pathological feature of malignant tumors; that is, tumor cells can be observed in the space surrounded by endothelial cells (including lymphatic space and vascular space) around the tumor tissue. Relevant studies have shown that LVSI is an independent risk factor of lymph node metastasis in cervical cancer [5, 6]. In the process of tumor metastasis, tumor cells break off from the primary tumor entering into the vascular system and form tumor thrombi, which further spreads to invade other organs. Although the effect of LVSI on the prognosis of cervical cancer is still inconclusive, many studies have confirmed that positive LVSI indicates poor prognosis for cervical cancer [7, 8, 9]. The National Comprehensive Cancer Network (NCCN) clinical practice guidelines for cervical cancer have also demonstrated that the treatment decision of early-stage cervical cancer varies on LVSI assessment. Stage IA1 patients with negative LVSI can be treated with simple hysterectomy, while those with positive LVSI require treatment similar to stage IA2 with modified radical hysterectomy with bilateral pelvic lymphadenectomy or sentinel lymph node visualization. Therefore, accurate assessment of LVSI is of great value for the formulation of individualized treatment plans for cervical cancer patients.
At present, the clinical evaluation of LVSI mainly depends on pathologic assessment. Accuracy of preoperative evaluation of LVSI is greatly restricted by the limited biopsy specimens. Therefore, it is extremely valuable to explore an accurate non-invasive method for preoperative evaluation of LVSI. Magnetic resonance imaging (MRI) has been widely used in the diagnosis and treatment of gynecological malignancies, including cervical cancer and endometrial cancer due to its excellent soft tissue resolution [10, 11], as well as for the evaluation of LVSI [12]. Compared with traditional imaging, radiomics allows data mining into images through high-throughput extraction of quantitative features to support clinical decisions [13]. Some investigators have evaluated the role of radiomics in gynecological malignancies, including ovarian, cervical and endometrial cancer, mostly evaluating association with tumor prognostic factors, response to therapy and prediction of recurrence and metastasis. Other studies have correlated radiomic features with molecular/genomic profiling to classify prognosis [14, 15].
The aim of this study was to establish radiomic models using three different MRI sequences (T2 weighted imaging (T2WI), diffusion weighted imaging (DWI), and contrast-enhanced T1 weighted imaging (T1WI + C)) combined with clinical information, in order to explore the application value of radiomic models in the preoperative evaluation of LVSI in cervical cancer.
This study retrospectively collected 157 patients who underwent gynecological pelvic MRI in our hospital from January 2018 to December 2020. Relevant clinical data including age, squamous cell carcinoma antigen (SCC) value (1–2 days before surgery) and postoperative pathological results were recorded.
The inclusion criteria for patients were as follows: ① patients
underwent gynecological pelvic MRI examination before operation which included
T2WI, DWI (b value was 800 s/mm
The exclusion criteria for patients were as follows: ① any form of treatment before MRI examination, such as medication, surgery, radiotherapy and neoadjuvant chemotherapy; ② lesions too small to have been accurately identified on MRI; ③ poor quality MRI, such as images with significant artifacts; ④ incomplete imaging or clinical information.
As a result, 101 patients were included in this study and the patient enrollment process is shown in Fig. 1. We assigned 60% (n = 61) of the patients to the train set and 40% (n = 40) to the test set by stratified randomized sampling.
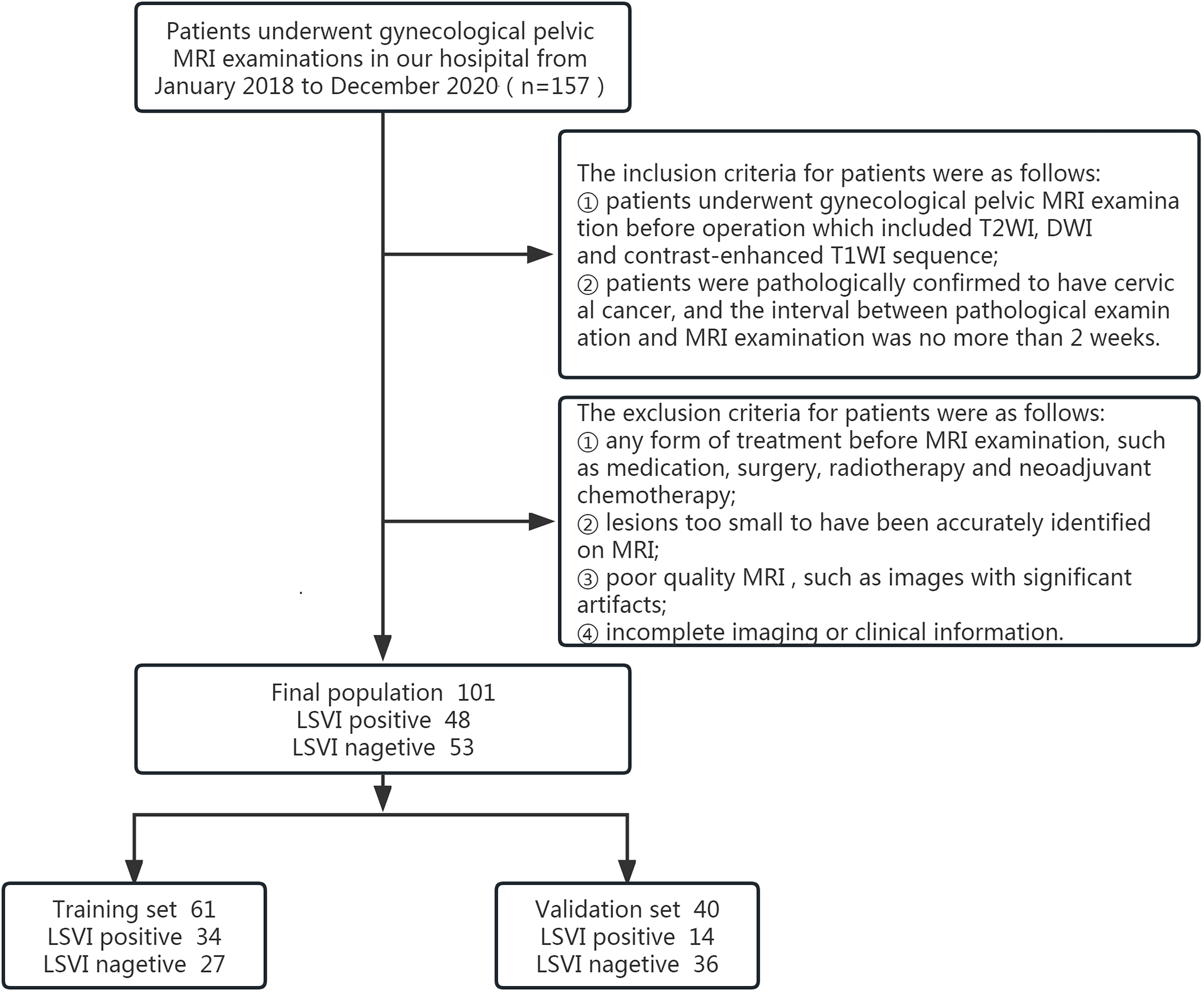
Flowchart of inclusion. LVSI, lymphovascular space invasion; MRI, magnetic resonance imaging; T2WI, T2 weighted imaging; DWI, diffusion weighted imaging; T1WI, T1 weighted imaging.
All MRI examinations were performed on a 3.0T magnetic resonance scanner
(Ingenia3.0T; Philips Healthcare, Best, the Netherlands). Magnetic resonance
imaging sequences included the following: axial and sagittal T2-weighted turbo
spin-echo (T2W-TSE) sequence; axial T1 high resolution isotropic volume
examination (THRIVE) sequence; axial and sagittal T2 spectral presaturation
attenuated inversion recovery (SPAIR). The contrast medium was Omniscan (GE
Healthcare Ireland, Shanghai, China), which was injected intravenously at a rate
of 3.0 mL/s with the dose of 0.2 mL/kg body weight, followed by 15 mL saline
flush. The high pressure injector used was Medrad Spectris Solaris EP MR Injector
System (One Medrad Drive, Indianola, PA, USA). B values of 0 and 800 s/mm
T2WI, DWI (b value = 800 s/mm
MRI images of all patients (including axial T2WI, DWI and T1WI + C sequences) were imported into ITK-SNAP 3.0 software (Cognitica, Philadelphia, PA, USA; http://www.itksnap.org) in digital imaging and communications in medicine (DICOM) format. The identified lesions were manually segmented layer by layer by a radiologist with 4 years’ experience in gynecological imaging and rechecked by a second radiologist with 15 years’ experience. One week later, T2WI, DWI and T1WI + C images of 25 patients were randomly selected and outlined again for intraclass correlation coefficient (ICC) feature screening.
The criteria for manual segmentation: (1) outline along the boundary of the lesion in order for the maximum volume of interest (VOI); (2) necrosis and bleeding area inside the lesion were included in order to reflect the heterogeneity of the tumor; (3) adjacent organs such as rectum and bladder were avoided.
The workflow of radiomics is shown in Fig. 2.
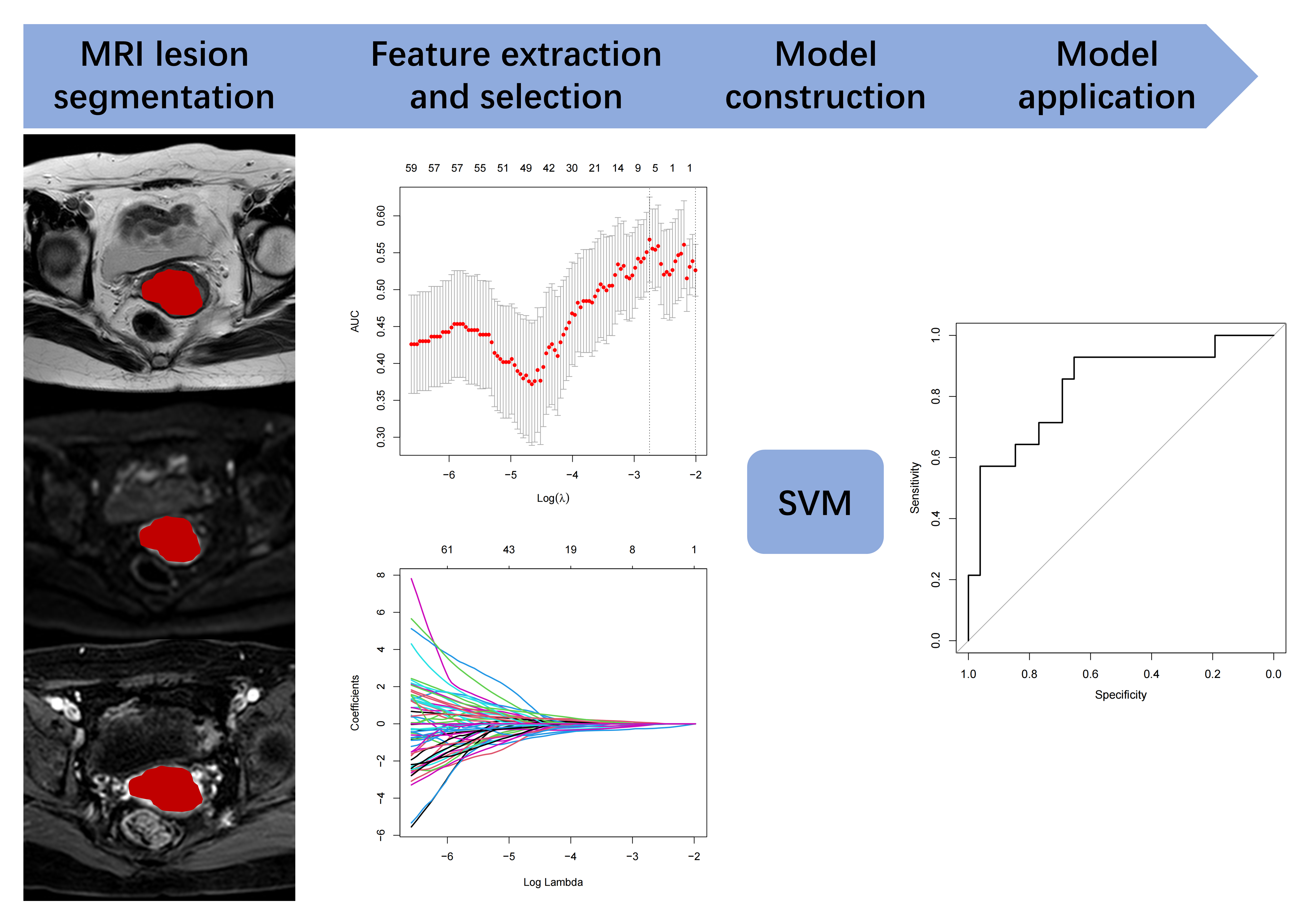
Workflow of this research. MRI, magnetic resonance imaging; SVM, support vector machine; ROC, receiver operating characteristic; AUC, area under the ROC curve.
A total of 1158 features were extracted from T2WI, DWI and T1WI + C modes,
including morphological features, first-order statistical features, and texture
features (gray level co-occurrence matrix, GLCM; gray level run-length matrix,
GLRLM; gray level region size matrix, GLSZM; gray level difference matrix, GLDM).
Higher-order features were extracted after Laplacian Gaussian transform and
Wavelet transform. Radiomics features were extracted using IBSI-compliant
pyradiomics software (version 3.0.1) (https://www.radiomics.io/). Image preprocessing was
performed before feature extraction, including resampling to a voxel size of 1
The 101 lesions were divided into the training set and the validation set
according to time period. For the training set, features with high stability were
first screened out according to the intra-group and inter-group consistency of
feature extraction (ICC
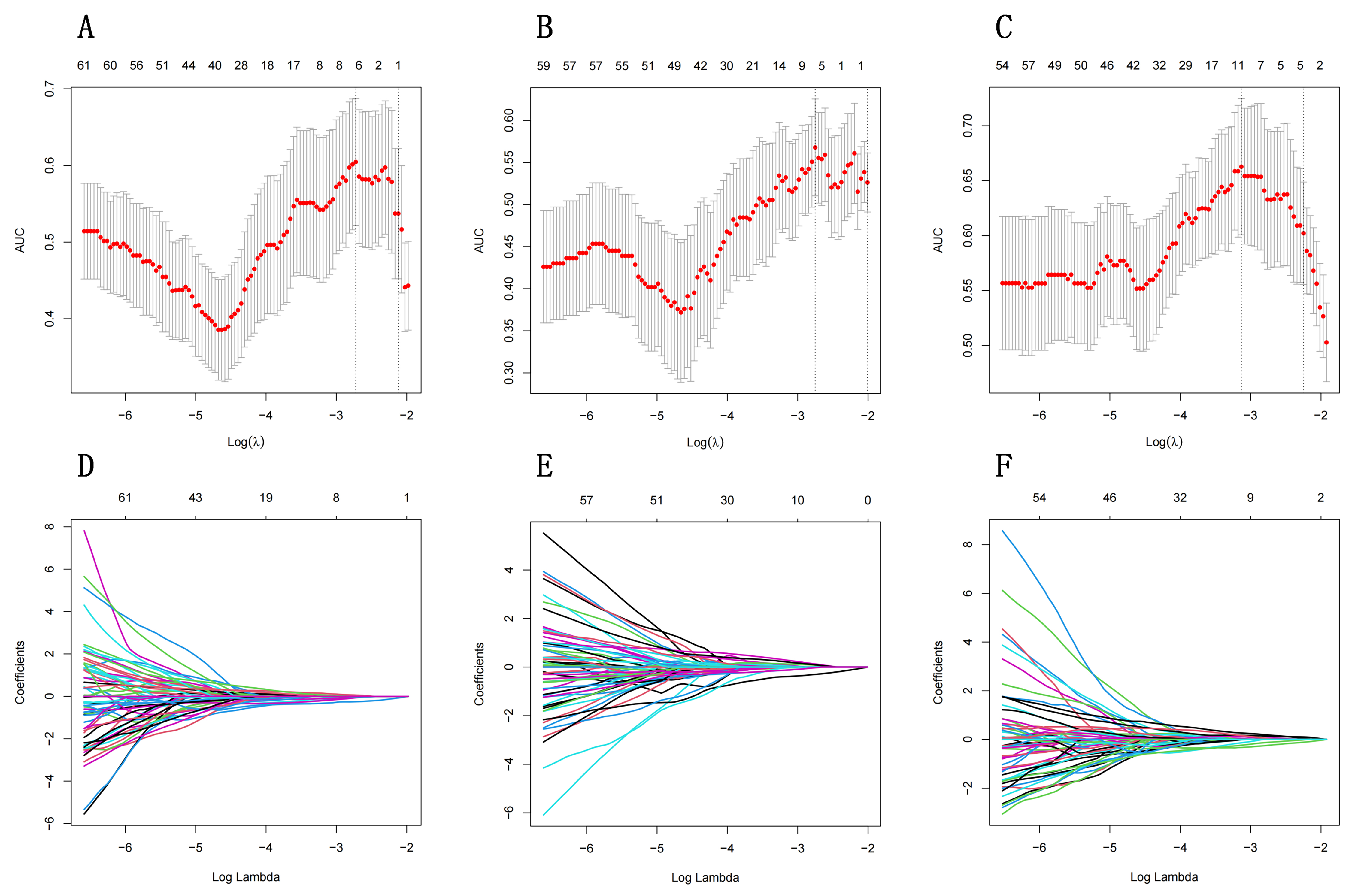
Selection of radiomics features using least absolute shrinkage
and selection operator (LASSO) regression. (A–C) show the optimal penalty
coefficient
Support vector machine (SVM) was used for the model establishment. SVM was implemented using the “e1071” package (https://CRAN.R-project.org/package=e1071) of R software (version 3.6.1, http://www.r-project.org). The type of SVM is “eps-regression”, of which the kernel function is radial basis. The total number of support vectors is 225.
All statistical analysis were performed using Rstudio (https://www.rstudio.com). Continuous quantitative
data with a normal distribution were expressed as the mean
A total of 101 patients were included in this study (mean age: 53.42
Total | LVSI (+) | LVSI (–) | p | ||
(n = 101) | (n = 48) | (n = 53) | |||
Age (years) | 53.42 |
53.25 |
53.57 |
0.853 | |
SCC (ng/mL) | 8.66 |
10.82 |
6.71 |
0.133 | |
Depth of myometrial invasion | 0.336 | ||||
80 | 40 | 40 | |||
21 | 8 | 13 | |||
Maximum diameter (cm) | 3.41 |
3.34 |
3.47 |
0.651 | |
ADC value (10 |
797.58 |
774.46 |
818.52 |
0.349 | |
Pathology n (%) | |||||
Squamous carcinoma | 84 (83.17) | 35 (34.65) | 49 (48.51) | ||
Adenocarcinoma | 16 (15.84) | 12 (11.88) | 4 (3.96) | ||
Mixed adenoendocrine carcinoma | 1 (0.99) | 1 (0.99) | 0 (0) |
Note: Age, SCC, maximum diameter of tumor, and ADC value are presented as mean
A total of 101 lesions were included in this study. Features of 1158 were extracted from the images of each mode, and 3474 features were extracted from the three modes.
All features extracted from each mode were retained according to inter-observer ICC coefficients (greater than 0.75), and then assessed by using LASSO regression algorithm. Twenty-one features were kept for the model building (9 from T2WI, 6 from DWI, 6 from ADC) (Table 2). No clinical features or traditional imaging features were selected by spearman correlation analysis (Table 1).
Model | Feature | Coefficient |
T2 | T2_wavelet.LHL_gldm_LargeDependenceLowGrayLevelEmphasis | –0.0150103717082837 |
T2_wavelet.LHH_glcm_MCC | –0.333102279446099 | |
T2_wavelet.LHH_glszm_GrayLevelNonUniformityNormalized | –0.139919403617482 | |
T2_wavelet.LHH_glszm_SmallAreaEmphasis | 0.0366815585921708 | |
T2_wavelet.LHH_glszm_SmallAreaLowGrayLevelEmphasis | 0.263604565314742 | |
T2_wavelet.HLH_glszm_GrayLevelNonUniformityNormalized | 0.115882962627615 | |
T2_wavelet.HLH_glszm_LowGrayLevelZoneEmphasis | 0.170168865479784 | |
T2_wavelet.HLH_glszm_SmallAreaEmphasis | –0.239807378370699 | |
T2_wavelet.HHH_glszm_SmallAreaLowGrayLevelEmphasis | 0.156686251059209 | |
DWI | DWI_log.sigma.4.0.mm.3D_glszm_SmallAreaLowGrayLevelEmphasis | –0.0388555271048709 |
DWI_wavelet.HLH_glszm_SmallAreaLowGrayLevelEmphasis | –0.0647164659164984 | |
DWI_wavelet.HHL_glcm_Imc2 | –0.255252033880791 | |
DWI_wavelet.HHL_glcm_MCC | –0.0247464157443933 | |
DWI_wavelet.HHH_glcm_MCC | –0.0245653313656513 | |
DWI_wavelet.HHH_glszm_GrayLevelNonUniformityNormalized | 0.0571260101709459 | |
T1 + C | T1C_wavelet.HLH_firstorder_Skewness | 0.127804041567352 |
T1C_wavelet.HLH_glcm_Imc2 | –0.300103234601108 | |
T1C_wavelet.HHL_firstorder_Median | 0.0819543795798403 | |
T1C_wavelet.HHL_firstorder_Skewness | –0.0181913948467193 | |
T1C_wavelet.HHH_glszm_SizeZoneNonUniformityNormalized | 0.0943811086627695 | |
T1C_wavelet.LLL_gldm_LargeDependenceLowGrayLevelEmphasis | –0.0271204202363728 |
SVM was used to establish a binary radiomics classifier to distinguish the lesion status of lymphovascular space invasion. The corresponding radiomic models were established based on different single modality and multi-modality (combined with T2WI, DWI and T1WI + C). Among them, the machine learning model based on multi-modality showed the best diagnostic efficiency in the evaluation of LVSI with AUC of 0.990 in the training set (sensitivity of 0.912 and specificity of 1.000) and AUC of 0.832 in the validation set (sensitivity of 0.929 and specificity of 0.654) (Figs. 4,5,6).
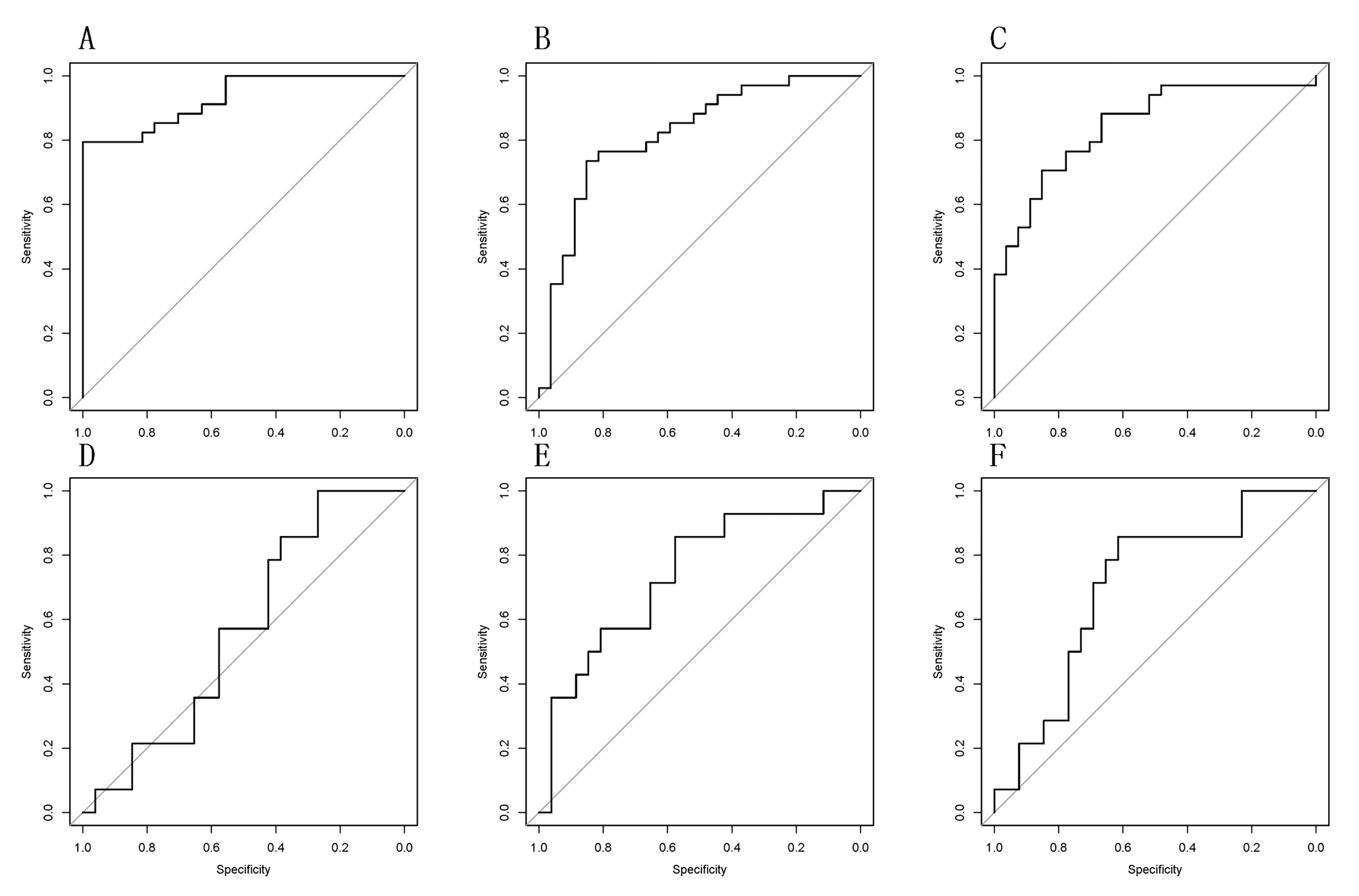
Receiver operating characteristic (ROC) curve for preoperative evaluation of lymphovascular space invasion in cervical cancer based on single-modality radiomics. (A–C) ROC curves of T2WI, DWI and T1WI + C modes in the training set (area under the ROC curve (AUC) values are 0.929, 0.845 and 0.819, respectively). (D–F) ROC curves of T2WI, DWI and T1WI + C modes in the validation set ROC curve (AUC values are 0.563, 0.703, 0.739, respectively).
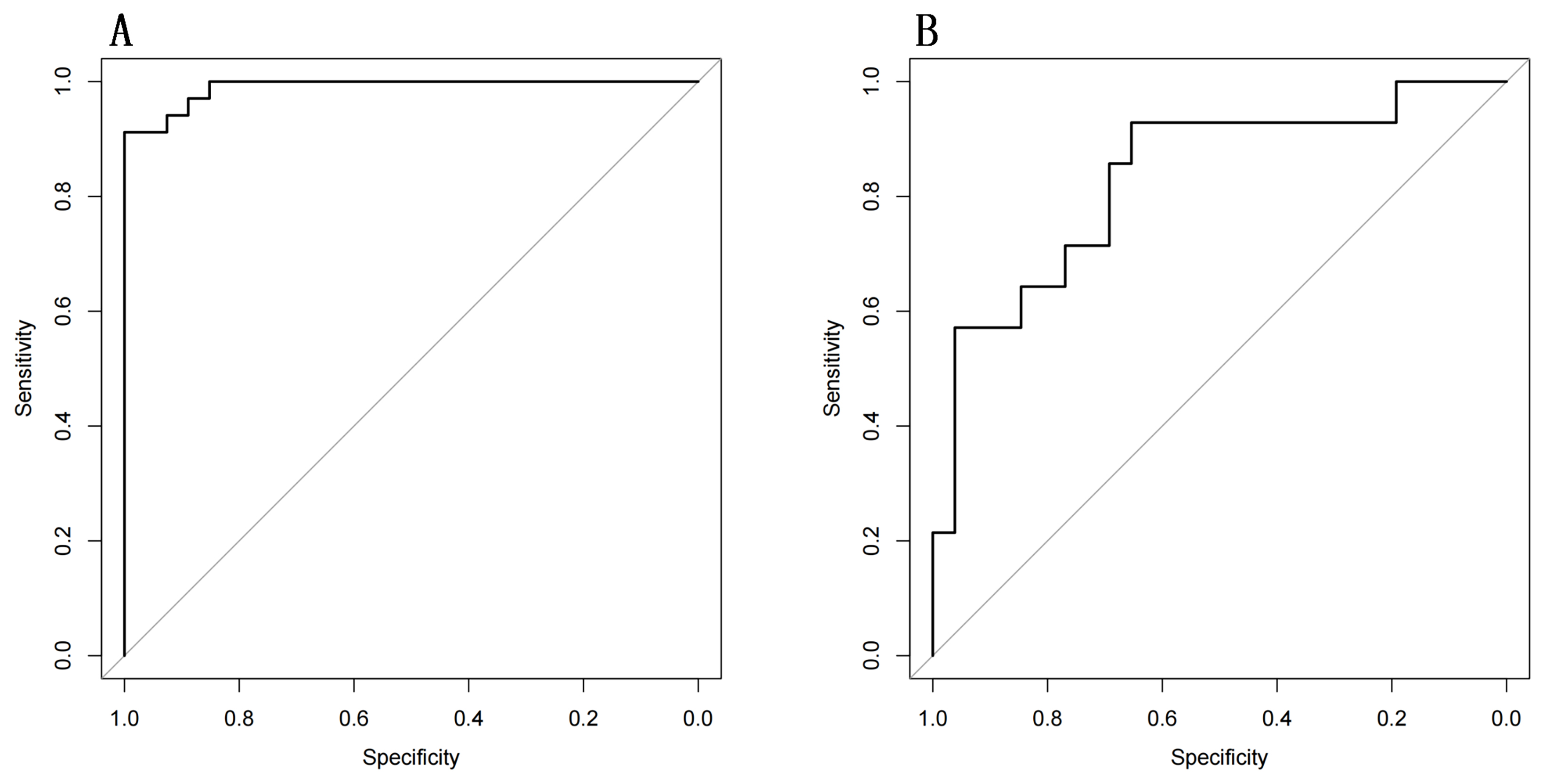
ROC curve of multimodal radiomics model for preoperative evaluation of lymphovascular space invasion in cervical cancer. (A) ROC curve of multimodal radiomics in the training set (AUC = 0.990 [95% confidence interval (95% CI): 0.975–0.999] with sensitivity of 0.912 and specificity of 1.000). (B) ROC curve of multimodal radiomics in the validation set (AUC = 0.832 [95% CI: 0.693–0.971] with sensitivity of 0.929 and specificity of 0.654).
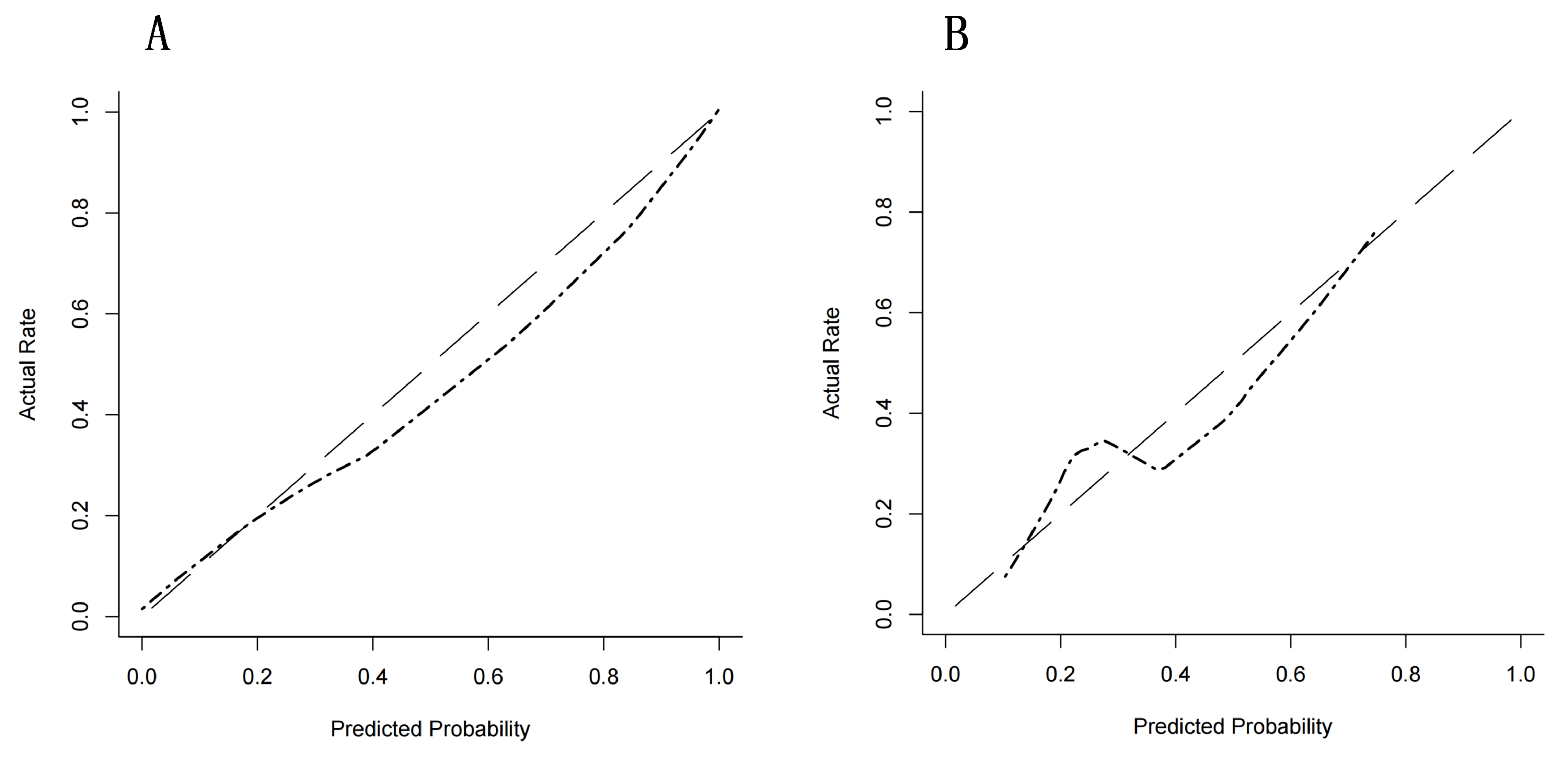
Calibration curve of prediction model for multimodal radiomics alignment. (A) Calibration curve of multimodal radiomics in the training set (Sig = 0.999). (B) Calibration curve of multimodal radiomics in validation dataset (Sig = 0.461).
Due to excellent soft tissue resolution, multi-parameter and multi-sequence imaging, MRI plays an important role in the clinical practice for cervical cancer. In recent years, the rise of radiomics has further expanded the application of MRI, enabling clinicians to deeply explore the hidden information in images [15]. The aim of this study was to construct radiomic models by using three conventional sequences (T2WI, DWI and T1WI + C) and to explore the new application of conventional sequences in the preoperative evaluation of LVSI in cervical cancer.
Clinical parameters including age, preoperative SCC value and the depth of muscular invasion were collected for this study, with no significant difference being found between the LVSI positive and negative group.
SCC is a serine protease inhibitor, which inhibits cell apoptosis and
participates in the metabolism, proliferation, as well as differentiation of
tumor cells. The average SCC value of patients enrolled in this study was 8.66
The maximum diameter and ADC value of the tumor were measured in this study,
with no significant difference being confirmed between the two groups (p
= 0.651 and 0.349 respectively). Malek et al. [19] showed that the mean
ADC value of the LVSI positive group (0.83
Compared with the clinical and traditional imaging parameters used in this study, radiomics parameters showed great potential in the preoperative LVSI evaluation of cervical cancer (Figs. 4,5). T2_wavelet.LHH_glcm_MC, T2_wavelet.LHH_glszm_SmallAreaLowGrayLevelEmph- asis, DWI_wavelet.HHL_glcm_Imc2 and T1C_wavelet. HLH_glcm_Imc2 showed higher weights during the selection of features. GLCM contains spatial information about the relationship of pixel pairs with similar or specific intensity within an image, while GLSZM measures groups of pixels with the same intensity regard- less of direction. Both of them can reflect the heterogeneity of the tumor. Peeken et al.’s [22] research showed similar results as the wavelet features could reflect multifrequency information of spatial heterogeneity of tumors. Among the features, wavelet.HLH_glcm_Imc2 were negatively correlated with LVSI in DWI and T1WI models, indicating lower wavelet.HLH_glcm_Imc2 and less linear structures in lesions with LVSI [23].
Numerous studies have applied radiomics in the evaluation of LVSI in cervical cancer. For example, Wu et al. [24] showed that radiomics features of T2WI, T2 with fat suppression (T2-FS), DWI and dynamic contrast enhanced (DCE) sequences can help in evaluating LVSI of cervical cancer with AUC values ranging from 0.659 to 0.814, among which, DCE sequence showed the best performance. Similar results were produced in our study, as AUC values of the single-modality radiomics parameters ranged from 0.845 to 0.929 in the training set and 0.563 to 0.739 in the validation set, of which the T1WI + C sequence showed the best performance. Similar findings may suggest that radiomics features related to tumor perfusion are better at evaluating LVSI than the diffusion-related radiomics features. Although the assessment efficiency of DCE sequence used by Wu et al. [24] is slightly superior to that of T1WI + C sequence used in this study, it requires a more rigorous operation and longer scanning time than T1WI + C sequence. Therefore, the routine sequence (T1WI + C) was eventually chosen in this study.
In addition, our study further established the radiomics model combined three different modality parameters, including T2WI, DWI and T1WI + C. The results demonstrated that performance of the comprehensive model in evaluating LVSI was significantly better than that of the single-mode radiomics model, with an AUC value of 0.990 in the training set and 0.832 in the validation set (Figs. 5,6). The research of Huang et al. [25] also showed similar results, with their AUC values of single-mode radiomics model ranged from 0.681 to 0.878, while AUC value of the comprehensive model was 0.922. This suggests that with more parameters involved, more accurate information of tumor internal characteristics can be provided, resulting in better prediction efficiency.
This study has some limitations. First, the results were established in a single center with limited sample size, and this study did not review external validation datasets. Future studies should recruit a larger cohort of patients in multi-center study to confirm our results. Second, manual segmentation of the region of interest (ROI) is a time-consuming process and more subjective, automatic segmentation is crucial and objective for promoting the feasibility of radiometric measures. Third, because of limited cases and subtypes, we did not compare the depth of myometrial invasion subgroups. Nevertheless, our results suggest that the benefits of a radiomics model to suspect the presence of LVSI of cervical cancer.
Radiomics holds great potential in the evaluation of LVSI in cervical cancer. Its diagnostic efficacy is superior to conventional MRI and clinical parameters. Radiomics has the potential to be an effective method for preoperative LVSI assessment for cervical cancer, and can provide strong support for clinical diagnosis and treatment protocols.
The datasets used and analyzed during the current study are available from the corresponding author on reasonable request.
LiZ, YJ and ZZ designed the research study. YJ performed the research. LijingZ collected data and made treatment decision. HW, JM and GD analyzed the data and wrote the manuscript. All authors contributed to editorial changes in the manuscript. All authors read and approved the final manuscript. All authors have participated sufficiently in the work and agreed to be accountable for all aspects of the work.
All subjects gave their informed consent for inclusion before they participated in the study. The study was conducted in accordance with the Declaration of Helsinki, and the protocol was approved by the Ethics Committee of Nanjing Drum Tower Hospital (approval number: 2024-095-01).
With many thanks to all our colleagues in the department of Radiology and Gynaecology and Obstetrics for their supports and assistance during this research.
This research was funded by the Key project foundation of Nanjing for the development of medical technology (ID: ZKX 20024).
The authors declare no conflict of interest.
Publisher’s Note: IMR Press stays neutral with regard to jurisdictional claims in published maps and institutional affiliations.