- Academic Editor
Background: Alzheimer’s disease (AD) is a brain disorder characterized
by atrophy of cerebral cortex and neurofibrillary tangles. Accurate
identification of individuals at high risk of developing AD is key to early
intervention. Combining neuroimaging markers derived from diffusion tensor images
with machine learning techniques, unique anatomical patterns can be identified
and further distinguished between AD and healthy control (HC). Methods:
In this study, 37 AD patients (ADs) and 36 healthy controls (HCs) from the
Alzheimer’s Disease Neuroimaging Initiative were applied to tract-based spatial
statistics (TBSS) analysis and multi-metric classification research.
Results: The TBSS results showed that the corona radiata, corpus
callosum and superior longitudinal fasciculus were the white matter fiber tracts
which mainly suffered the severe damage in ADs. Using support vector machine
recursive feature elimination (SVM-RFE) method, the classification performance
received a decent improvement. In addition, the integration of fractional
anisotropy (FA) + mean diffusivity (MD) + radial diffusivity (RD) into
multi-metric could effectively separate ADs from HCs. The rank of significance of
diffusion metrics was FA
Alzheimer’s disease (AD) is associated with abnormal functioning of the nervous system and usually appears in people over the age of 60. Patients are often accompanied by memory loss and cognitive decline and other problems, which not only seriously harms the physical and mental health of patients, but also brings a heavy burden on families and society [1]. It is estimated that in 30 years there will be 134.6 million cases of AD worldwide [2]. However, the exact cause of AD is still unknown and existing targeted drugs can only reduce symptoms or delay its progression. Therefore, revealing the brain changes caused by the disease is crucial to explore the underlying cause of AD [3].
Precise diagnosis of AD helps patients improve future quality of life, including early prevention and optimal treatment [4]. With the fast development of artificial intelligence and medical imaging technology, computer-aided diagnosis provides sufficient evidence of accuracy to distinguish AD from healthy control (HC) [5, 6, 7, 8, 9, 10]. Traditionally, AD has been thought of as a disease of gray matter (GM) damage, while the effects of white matter (WM) have generally been thought of as damage secondary to GM [11]. Although there is growing concern about WM damage in AD, our knowledge is still limited when compared to GM atrophy and other biomarkers. In particular, a review illustrates different main research point of penetration for how WM injury leads to AD [12]. One piece of evidence is to study WM degeneration and demyelination as the important pathophysiological features of AD at the microstructural level [13, 14]. Another piece of evidence comes from neuroimaging, which have the remarkable advantage of being able to noninvasively observe morphologic changes in patients’ brains. Despite the study is focused on WM microstructures, it’s important to highlight that diffusion tensor imaging (DTI) can be used to investigate even microstructural changes in GM (not only WM) [15, 16]. With the development of DTI technology, it can be used to show the direction of fasciculus in the WM of the brain, which is the only non-invasive imaging method that can show the fasciculus in vivo [17].
There are some common metrics that can reflect the brain microstructure in DTI, such as fractional anisotropy (FA), mean diffusivity (MD), axial diffusivity (DA) and radial diffusivity (RD). FA represents the directivity of water molecular dispersion and can reflect the maximum possible arrangement direction of WM tracts. The FA value is higher in WM, close to 1, while it is close to 0 in cerebrospinal fluid. MD represents the overall dispersion of water molecules. DA represents the degree of dispersion of water molecules along the main direction. RD represents the dispersion of water molecules in the other two directions [18, 19]. For further understand the pathological mechanisms of WM tracts’ change, tract-based spatial statistics (TBSS) method has been applied to research the microstructural of WM [20]. In recent years, machine learning-based neuroimaging technology for AD diagnosis and disease development has become a research hotspot [21, 22, 23, 24]. As a widely used supervised learning method, support vector machine (SVM) shows good advantages in solving small sample, nonlinear and high-dimensional pattern recognition problems [25, 26, 27, 28].
Here, we aimed to analyze research microstructural difference of WM and predict the accuracy between ADs and HCs. Therefore, the TBSS and SVM method will be used in our study. Specifically, we combined with different kind of DTI metric together for improving classification performance and rank the importance of the WM fiber tract.
A total of 73 subjects from Alzheimer’s Disease Neuroimaging Initiative Grand Opportunities/phase 2 (ADNI-GO/2) database (http://adni.loni.usc.edu/) [29] were collected for this study. As everyone knows, the ADNI dataset has the multi-site nature which characterized by different scanners and acquisition protocols, can have an impact on DTI data such as noise and bias. However, harmonization methods already applied on ADNI DTI data in other studies [30, 31] could fix this issue in the future. Before scanning, the subjects undergo cognitive and behavioral assessments. Statistical analysis of basic information in Table 1 is completed in SPSS 22.0 (IBM Corp., Armonk, NY, USA). The Table 1 display p-values and t-value for two sample t-tests for each sample characteristic except for gender, which displays p-values and chi square value from a Chi square test.
ADs (n = 37) | HCs (n = 36) | p-value | Chi2/t-value | |
Gender (male:female) | 25:12 | 19:17 | 0.197 | 1.667 |
Age (years) | 74.81 |
73.28 |
0.398 | 0.85 |
MMSE | 23.38 |
28.81 |
–12.984 | |
CDR | 4.55 |
0.03 |
19.153 |
Data is mean
All subjects are scanned through a 3T GE MEDICAL SYSTEMS scanner (General Electric company, Boston, MA, USA). A whole brain
diffusion MRI (dMRI) SE-EPI (spin-echo echo-planar imaging) is acquired with the
following parameters: echo time (TE): 68.3 ms, repetition time (TR): 13,000 ms, Slice Thickness: 2.7 mm, Field
Strength: 3.0, Flip Angle: 90 degree, 128 mm
Currently, PANDA (A Pipeline for Analysing Brain Diffusion Images) [32] is commonly used to process DTI data. PANDA software package is based on Linux operating system (Ubuntu 21.04, Canonical, London, UK) and Matlab software (MATLAB 9.7, MathWorks, Natick, MA, USA). In addition, PANDA’s underlying commands invoke Functional magnetic resonance imaging of the brain (FMRIB) Software Library (FSL) tools [33], Diffusion Toolkit [34], Pipeline System for Octave and Matlab (PSOM) [35], and MRIcron tools (https://people.cas.sc.edu/rorden/mricron/install.html). The preprocessing steps mainly include: (1) converting Digital Imaging and Communications in Medicine (DICOM) data to Neuroimaging Informatics Technology Initiative (NIFTI) format; (2) head movement and eddy current correction; (3) brain tissue was removed by Brain Extraction Tool (BET); (4) DTI metrics (i.e., FA, MD, DA, RD) calculation with non-linear fitting algorithm.
The tract-based spatial statistics (TBSS) can fully reflect the microstructural
changes of the whole brain WM and the skeletonized data processing method can
obtain high accuracy without smoothing [20]. All subjects’ FA maps were
nonlinearly aligned to a 1
rICBM-DTI-81 WMPM FMRIB58 atlas [36] | |||
Middle cerebellar peduncle | MCP | Pontine crossing tract | PCT |
Splenium of corpus callosum | SCC | Column and body of fornix | CBF |
Genu of corpus callosum | GCC | Body of corpus callosum | BCC |
Medial lemniscus.R | ML.R | Medial lemniscus.L | ML.L |
Corticospinal tract.R | CT.R | Corticospinal tract.L | CT.L |
Superior cerebellar peduncle.R | SCP.R | Superior cerebellar peduncle.L | SCP.L |
Inferior cerebellar peduncle.R | ICP.R | Inferior cerebellar peduncle.L | ICP.L |
Cerebral peduncle.R | CP.R | Cerebral peduncle.L | CP.L |
Posterior limb of internal capsule.R | PLIC.R | Posterior limb of internal capsule.L | PLIC.L |
Anterior limb of internal capsule.R | ALIC.R | Anterior limb of internal capsule.L | ALIC.L |
Retrolenticular part of internal capsule.R | RPIC.R | Retrolenticular part of internal capsule.L | RPIC.L |
Posterior thalamic radiation.R | PTR.R | Posterior thalamic radiation.L | PTR.L |
Superior corona radiata.R | SCR.R | Superior corona radiata.L | SCR.L |
Anterior corona radiata.R | ACR.R | Anterior corona radiata.L | ACR.L |
Posterior corona radiata.R | PCR.R | Posterior corona radiata.L | PCR.L |
External capsule.R | EC.R | External capsule.L | EC.L |
Sagittal stratum.R | SS.R | Sagittal stratum.L | SS.L |
Hippocampus gyrus.R | HG.R | Hippocampus gyrus.L | HG.L |
Cingulate gyrus.R | CG.R | Cingulate gyrus.L | CG.L |
Stria terminalis.R | ST.R | Stria terminalis.L | ST.L |
Superior fronto-occipital fasciculus.R | SFOF.R | Superior fronto-occipital fasciculus.L | SFOF.L |
Superior longitudinal fasciculus.R | SLF.R | Superior longitudinal fasciculus.L | SLF.L |
Uncinate fasciculus.R | UF.R | Uncinate fasciculus.L | UF.L |
Inferior fronto-occipital fasciculus.R | IFOF.R | Inferior fronto-occipital fasciculus.L | IFOF.L |
Tapetum.R | TAP.R | Tapetum.L | TAP.L |
The machine learning algorithm used in this study comes from Python’s scikit-learn library [37]. The present application demonstrates that SVM was very good at mining information features [38]. Different type of SVM such as linear, non-linear with different kernel, SVM with recursive feature elimination (RFE) or regularization were applied to classify different disorders. In this paper, linear support vector machine recursive feature elimination (SVM-RFE) [39] was used to obtain the feature weight ranking that could best distinguished ADs and HCs. The SVM-RFE method could gradually minimize superfluous and irrelevant features [40]. The SVM-RFE method eliminated useless features one by one during each recursive process and had been successfully applied to feature selection in several functional neuroimaging studies [41, 42]. In addition, the leave-one-out cross validation (LOOCV) method was used for cross validation [43]. In this process, for each selected number of features, N classifications were made (where N corresponds to the number of subjects). The mean value of N classification accuracies was similar to the classification accuracy of corresponding feature numbers in the training data set.
The result of classification is the mean accuracy, sensitivity and specificity. Sensitivity is the percentage of samples that are actually positive that are judged to be positive. It is calculated as the ratio of true positive (TP) divided by true positive (TP) + false negative (FN) (actually positive but judged negative). Specificity refers to the proportion of samples that are actually negative that are judged to be negative. It is calculated as the ratio of true negative (TN) divided by true negative (TN) + false positive (FP) (actually negative but judged positive). Accuracy is expressed by the percentage of the total number of TP and TN in the number of subjects. For a more complete understanding of the classifier’s performance, sensitivity, specificity, and overall accuracy should be reported. Another very common method of reporting binary classifier results is to plot a receiver operating characteristic (ROC) curve [44]. ROC curve is a complete image of classifier performance provided by setting classification threshold value, in which, the horizontal coordinate represents false positive rate (FPR) (i.e., 1-specificity), and the vertical coordinate represents true positive rate (TPR), i.e., sensitivity. It is always desirable to have a numerical value to indicate whether a classifier is good or bad. The area under ROC curve (AUC) is the size of the area below the ROC curve. Typically, AUC values range from 0 to 1, with a larger AUC representing better performance. AUC is a standard used to measure the quality of a classification model [45].
There are no significant differences (p
As shown in Fig. 1 and Table 3, compared with HC group, FA values of several WM
regions in AD group decreased, and the WM fiber with the most significant
difference was ACR.L (cluster number
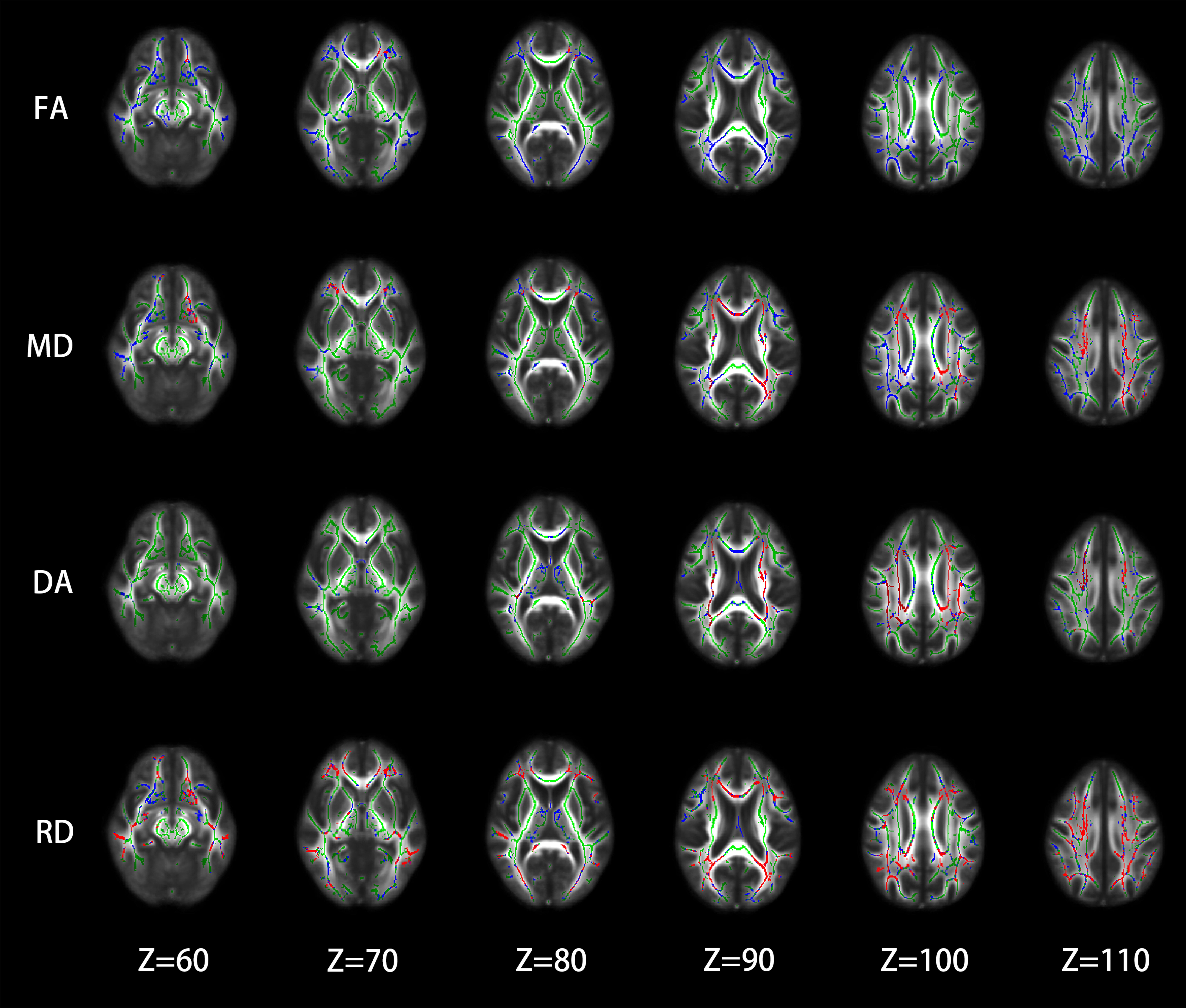
The TBSS images of the DTI metrics (i.e., FA, MD, DA, RD) in horizontal slices of brain. Each column represents the different Z-axis value from Z = 60 to Z = 110. Significantly decreased FA and significantly increased MD, DA, RD in ADs
WM tracts | ADs versus HCs cluster size | |||
FA | MD | DA | RD | |
GCC | 967 | 993* | 670 | 1072* |
BCC | 1507 | 2163* | 1367* | 2299* |
SCC | 2063 | 2155* | 1553* | 2428* |
CBF | - | - | 140 | 140 |
CP.R | 299 | - | - | 189* |
ALIC.L | - | 151 | 175 | - |
PLIC.R | 264 | 169 | 184* | 136 |
PLIC.L | - | 178 | 216* | - |
RPIC.R | - | - | 393* | - |
RPIC.L | - | - | 237* | - |
ACR.R | 958 | 1251* | 520* | 1328* |
ACR.L | 1095* | 1329* | 444* | 1414* |
SCR.R | 475 | 1520* | 1472* | 1014* |
SCR.L | 234 | 1390* | 1292* | 681* |
PCR.R | 388 | 650 | 671* | 539* |
PCR.L | 121 | 538* | 608* | 349* |
PTR.R | 721 | 186 | 167 | 673* |
PTR.L | 636 | 389* | 197* | 674* |
SS.R | 211 | 229 | - | 293* |
SS.L | 205 | 191 | - | 402* |
EC.L | - | 169 | 113 | - |
CG.R | 296 | 199 | - | 288 |
CG.L | - | 129 | - | - |
HG.R | 175 | - | - | 175* |
ST.R | 176 | - | - | 178* |
ST.L | 199 | - | - | 193* |
SLF.R | 773 | 1460 | 1028* | 1288* |
SLF.L | 472 | 1161* | 974* | 1012* |
IFOF.R | 117 | 213 | - | 183 |
IFOF.L | 106 | 260* | - | 255* |
Note: The numerical value showed above represent the
cluster number
Similarly, compared with HC group, MD values of several WM regions in AD group
increased, and the WM fiber with the most significant difference were GCC, BCC,
SCC, bilateral ACR, bilateral SCR, PCR.L, PTR.L, SLF.L, IFOF.L (cluster number
Similarly, compared with HC group, DA values of several WM regions in AD group
increased, and the WM fiber with the most significant difference were BCC, SCC,
bilateral PLIC, bilateral RPIC, bilateral ACR, bilateral SCR, bilateral PCR,
PTR.L, bilateral SLF (cluster number
Similarly, compared with HC group, RD values of several WM regions in AD group
increased, and the WM fiber with the most significant difference were GCC, BCC,
SCC, CP.R, bilateral ACR, bilateral SCR, bilateral PCR, bilateral PTR, bilateral
SS, HG.R, bilateral ST, bilateral SLF, IFOF.L (cluster number
The best number of features of 15 kinds of single metric or multi-metric had been drawn in the corresponding rectangular frame through the SVM-RFE and LOOCV. The X-axis value corresponding to the curve peak value was the best feature dimension.
Combined with Fig. 2 and Table 4, it could be found that FA+MD+RD received the highest accuracy which increased from 67.12% to 100% among all kinds of DTI metrics while its optimal feature dimension was not the minimum. Through SVM-RFE approach, the accuracy, sensitivity and specificity of classification received some measure of improvement. For some kinds of multi-metric, the penalty factors had been adjusted which marked with an asterisk in order to improve the classifying quality.
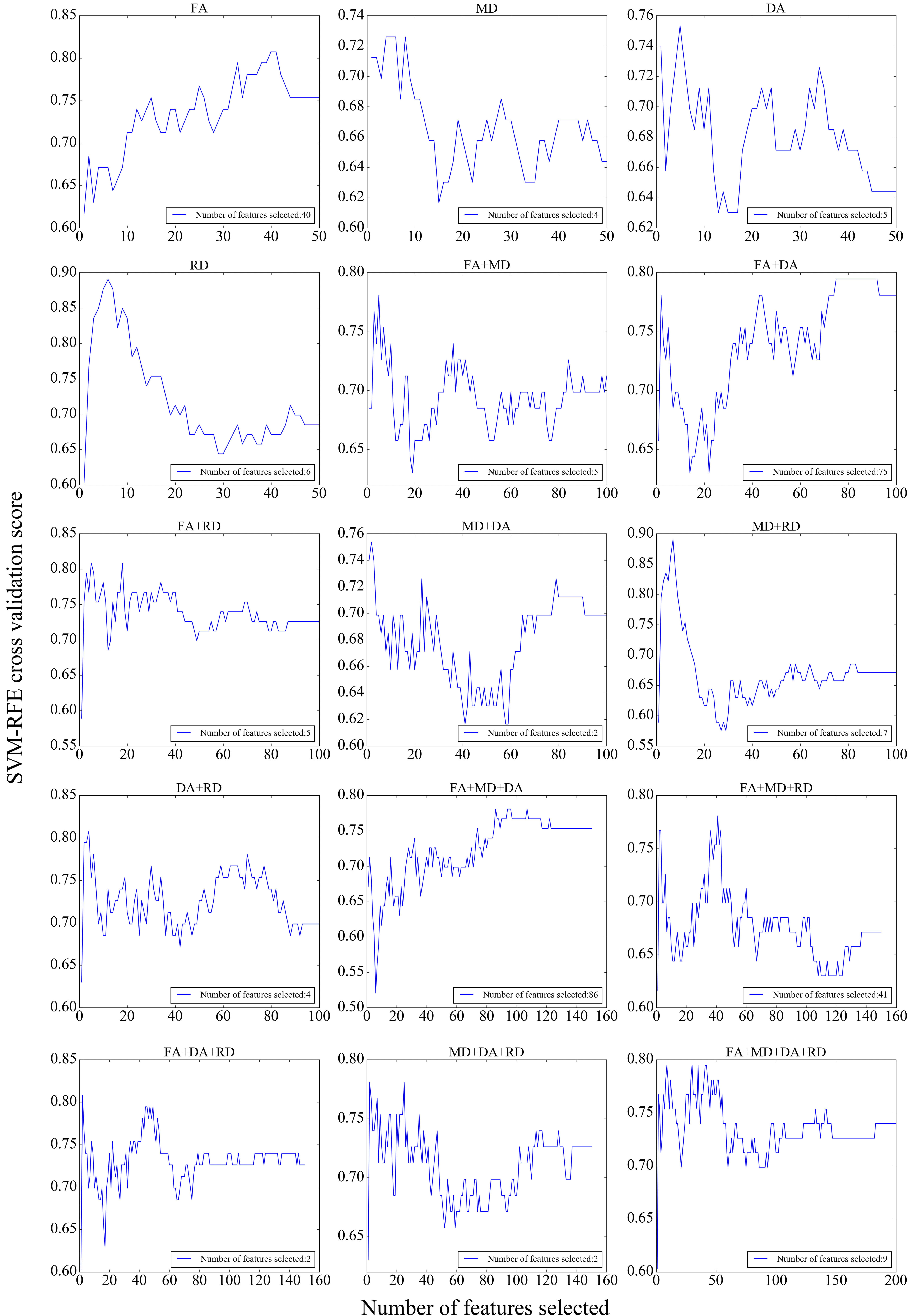
Feature ranking with SVM-RFE and LOOCV selection of the best number of features. Each subgraph represented the cross validation score corresponding to the number of features selected for 15 kinds of
Diffusion metrics | Optimal feature dimensions | Accuracy/% | Sensitivity/% | Specificity/% | |||
Before | After | Before | After | Before | After | ||
FA | 40 | 75.34 | 89.04 | 75.68 | 89.19 | 75.00 | 88.89 |
MD | 4 | 64.38 | 79.45 | 65.71 | 78.95 | 63.16 | 80.00 |
DA | 5 | 64.38 | 82.19 | 65.71 | 83.33 | 63.16 | 81.08 |
RD | 6 | 68.49 | 91.78 | 66.67 | 91.89 | 70.97 | 91.67 |
FA + MD | 5 | 71.23 | 86.30* | 72.22 | 90.91* | 70.27 | 82.5* |
FA + DA | 75 | 78.08 | 90.41 | 78.38 | 94.12 | 77.78 | 87.18 |
FA + RD | 5 | 72.60 | 89.04 | 71.79 | 89.19 | 73.53 | 88.89 |
MD + DA | 2 | 69.86 | 79.45 | 71.43 | 82.35 | 68.42 | 76.92 |
MD + RD | 7 | 67.12 | 93.15 | 66.67 | 94.44 | 67.65 | 91.89 |
DA + RD | 4 | 69.86 | 87.67 | 71.43 | 88.89 | 68.42 | 86.49 |
FA + MD + DA | 86 | 75.34 | 93.15 | 77.14 | 97.06 | 73.68 | 89.74 |
FA + MD + RD | 41 | 67.12 | 100 | 66.67 | 100 | 67.65 | 100 |
FA + DA + RD | 2 | 72.60 | 83.56* | 74.29 | 87.88* | 71.05 | 80* |
MD + DA + RD | 2 | 72.60 | 79.45 | 75.76 | 82.35 | 70.00 | 76.92 |
FA + MD + DA + RD | 9 | 73.97 | 91.78* | 76.47 | 97.06* | 71.79 | 87.5* |
The numerical value marked with an asterisk (*) represent a parameter optimization: FA + MD: C = 0.1; FA + DA + RD: C = 0.02; FA + MD + DA + RD: C = 0.1. The default C value is 1 and C represent penalty factor.
In order to investigate the weight distribution of different WM tract and the percent of different DTI metric on each WM tract, Fig. 3 was computed to depict the WM tract for which could classify the ADs from HCs.
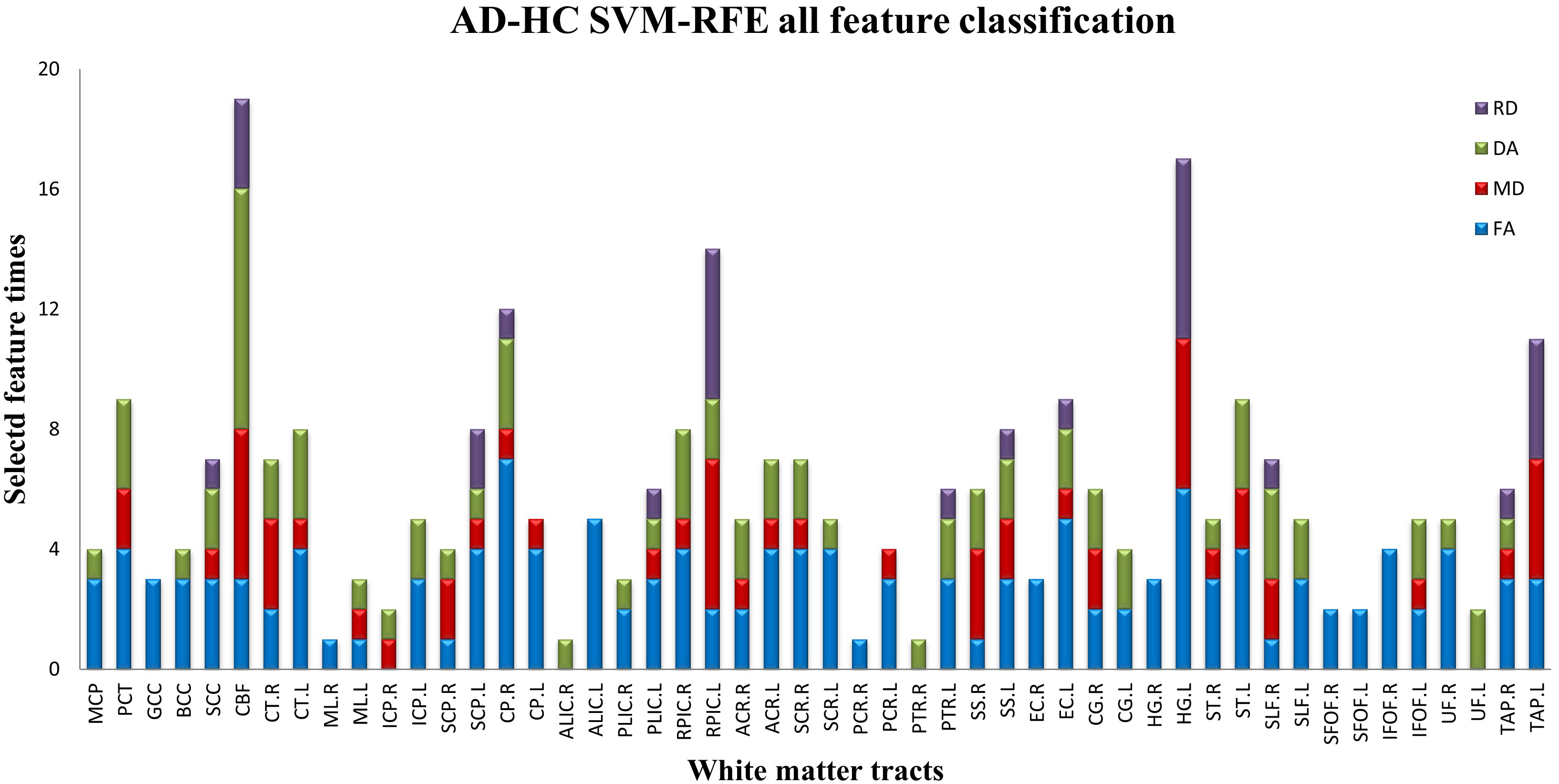
The feature weighting distribution of WM tracts received by the summation of all combined approaches. WM, white matter.
These ROC curves in Fig. 4 showed the classifier performance of different kind of DTI metric combined approach, computed from 5-fold cross-validation. Taking all of these curves, it was possible to calculate the AUC, and intuitively find the improvement of the classifier output performance. From above it could be found that the ROC curve which computed from FA+MD+RD received a high classifier output quality since it had a perfect AUC.
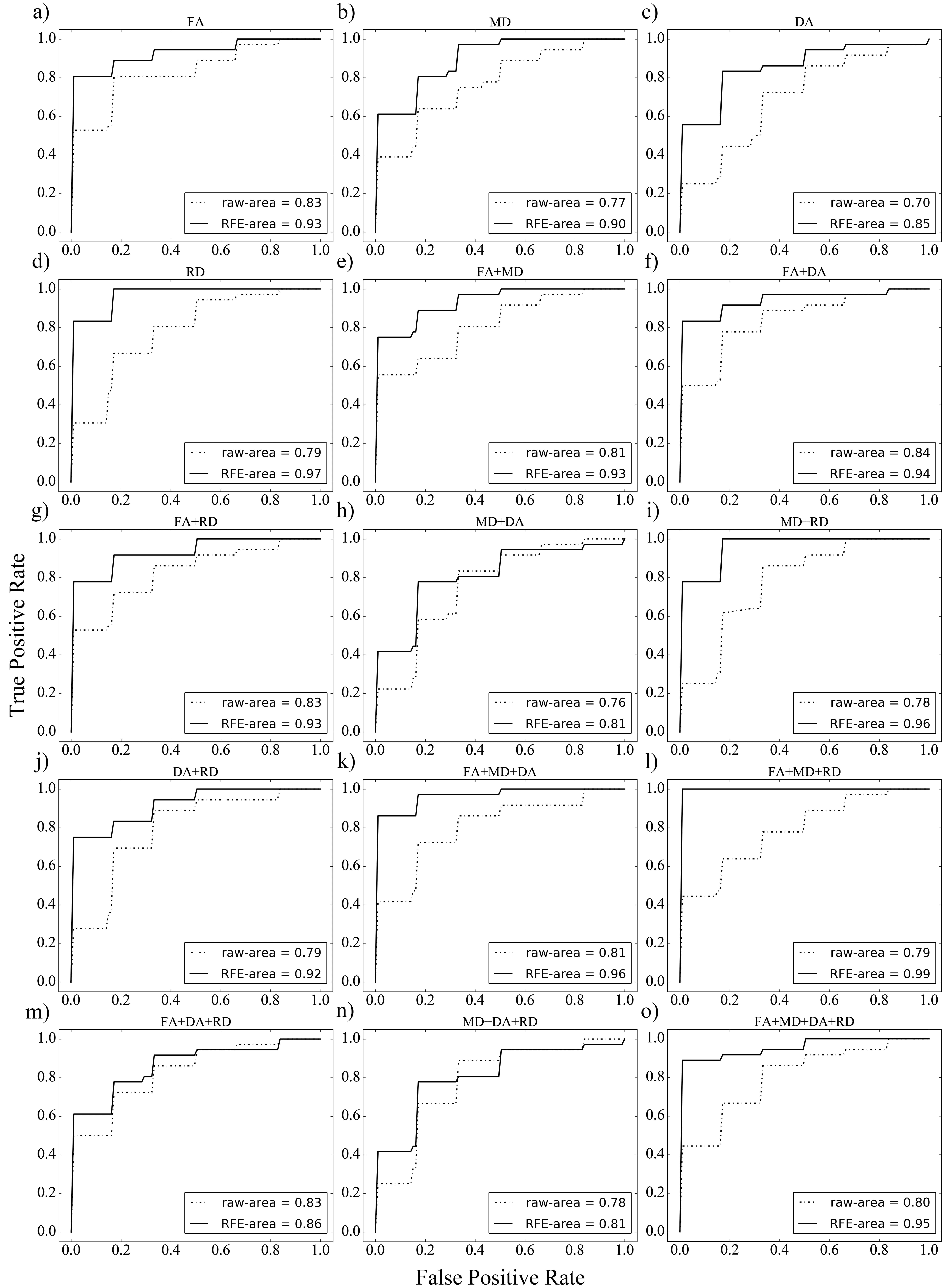
Different DTI metric of ROC curve to evaluate classifier performance by 5-fold cross-validation. The ROC curve with dotted line represented the AUC before SVM-RFE and the ROC curve with solid line represented the AUC after SVM-RFE. (a–o) represented the ROC curve and AUC for 15 kinds of DTI metric through combined approach, respectively. ROC, receiver operating characteristic; AUC, area under curve.
In our study, two aspects were mainly researched: statistical analysis of DTI data and classification. The subjects were firstly preprocessed using PANDA tool. Then the statistical analysis was operated by TBSS and classification process was conducted by SVM method.
Relevant literatures showed that the association fiber and limbic system were the most reported abnormal regions in the WM tracts of AD [46, 47, 48]. Cingulate was the association fiber between cingulate gyrus and other brain structures. Its integrity might directly relate to the emotion and cognitive function in AD patients. In our study, FA value of the right cingulate gyrus in ADs obviously decreased compared to HCs that was agreed with previous researches [49, 50]. In addition, association fiber contacted with part cortex of the ipsilateral hemisphere, FA value of bilateral sagittal stratum, superior fronto-occipital fasciculus and superior longitudinal fasciculus in ADs decreased compared with HCs that was agreed with Teipel’s research [51]. Correlational study found superior fronto-occipital fasciculus influenced visual spatial processing and memory function [52]. The damage of superior longitudinal fasciculus might involve spatial working memory and linguistic function [53]. The decrease of these functions was reflected on ADs than related to our research results. Optic radiation was the central neurons of visual pathway so that its lesion would lead to defect of field vision. In our study, decrease of FA value of posterior thalamic radiation in ADs hinted the damage of visual performance [54]. Wang et al. [55] based on TBSS with multi-parameter found that bilateral hippocampus gyrus existed obvious abnormal in ADs and patients with mild cognitive impairment (MCIs), including the decrease of FA value and increase of RD value, especially the right hemisphere in which was most significant. While our study found the difference just exists in the right hippocampus gyrus. MD value increased when the tissue damage. Increased MD and decreased FA were found in the corpus callosum that was agreed with previous research [56]. The corpus callosum was a bundle of fibers connecting the right and left hemispheres of the brain. On the basis of previous researches, the anterior part of the corpus callosum was connected to the prefrontal cortex and was associated with the sense of motivation [57].
Therefore, our results suggested that communication disorders between brain structures might be related to apathy symptoms of ADs [58, 59]. Moreover, the particular pattern of association between severity of apathy and corpus callosum integrity might reflect slower initiation and longer response times for tasks involving hemispheric metastasis or interregional integration in apathetic ADs. Previous results had demonstrated increased MD in most lobar regions of ADs, including frontal lobes [56], temporal lobes [56], parietal lobes [60], and occipital lobe [60]. DA and RD also increased in addition to FA and MD. However, the relevant research for DA and RD was little. Our findings suggested that DA and RD might be a useful biomarker in identifying HCs and ADs.
After the SVM-RFE method, each kind of DTI metric received the optimal feature
dimensions that listed in Table 4. Obviously, the majority of diffusion metrics
received optimal effect after dimensionality reduction. In addition, the
accuracy, sensitivity and specificity of each kind of diffusion metrics were
improved via the SVM-RFE method. Several kinds of multi-metrics were further
improved by adjusting the penalty factor C value. Table 4 showed that the
FA+MD+RD metric received the best classification accuracy. For further
investigated the effect of feature weighting on classification performance, Fig. 3 had been drawn to show the feature weighting distribution of WM tracts by the
summation of all combined approaches. The feature weighting of CBF, HG.L and
RPIC.L exceed 0.6 while that of ML.R, ALIC.R and PCR.R almost zero. It was also
found that the rank of significance of diffusion metrics was FA
In this paper, we introduced multi-metrics measures to identify the difference between HCs and ADs based on TBSS method. The corona radiata, corpus callosum and superior longitudinal fasciculus were the WM fiber tracts which mainly suffered the severe damage in ADs. Inter-group classification was completed by SVM-RFE method. Multi-metrics combination would improve the classification performance compared with single diffusion metric. We also depicted the feature weighting distribution of WM tracts for each kind of DTI metric in order to research which WM fiber tract played an important role on classification. In addition, the ROC curve and AUC could evaluate classifier output quality for each kind of diffusion metric.
There were several limitations to this research. First, small sample size would affect the reliability of classification results. Although our research used SVM model to distinguish AD group from HC group, it needed to be further verified on a larger sample to reinforce the current results and ensured that it had strong generalization ability. Deep learning could be combined if necessary. Another limitation was that the MCI group was absent from this study. MCI was known as a transition stage from health status to AD. It was necessary to include MCI in future studies to understand which features develop gradually over the course of the disease evolution and to reveal the degenerative pattern of the pathological mechanism of AD.
The datasets generated and/or analyzed during the current study are available in the ADNI repository, http://adni.loni.usc.edu/.
FZ designed the research study. YZ performed the research, analyzed the data and revised the manuscript. YZ and FZ wrote the manuscript. Both authors contributed to editorial changes in the manuscript. Both authors read and approved the final manuscript. Both authors have participated sufficiently in the work and agreed to be accountable for all aspects of the work.
The dataset we used was the public dataset of ADNI, the ethical approval was not required. In addition, informed written consent was obtained from all participants at every center.
We thank two anonymous reviewers for excellent criticism of the article.
This work was supported by the National Natural Science Foundation of China (Grant No.12202208), the Basic Science (Natural Science) Research Project of Colleges and Universities of Jiangsu Province (Grant No.22KJB310019, Grant No.22KJB130009), the Scientific Research Foundation of high-level personnel of Taizhou University (Grant No.TZXY2021QDJJ001), the Research and Cultivation Project for Young Teachers of Nanjing Audit University (Grant No.2021QNPY015), 2022 Double-Innovation Doctor of Jiangsu Province, “2022 Taizhou Tuo Ju Project” for Young science and Technology Talents, the Project of Excellent Science and Technology Innovation Team of Taizhou University.
The authors declare no conflict of interest.
Publisher’s Note: IMR Press stays neutral with regard to jurisdictional claims in published maps and institutional affiliations.