- Academic Editor
Background: Transcranial random noise stimulation (tRNS) is a form of noninvasive transcranial electrical stimulation that applies alternating current in various randomized frequencies to the cortex, thereby improving cognitive functioning in multiple domains. However, the precise mechanism of tRNS, as well as its impact on human electroencephalography (EEG), remains unclear. This is partly because most studies have used tRNS in conjunction with a cognitive task, making it difficult to tease apart whether the observed changes in EEG are a result of tRNS, the cognitive task, or their interaction. Methods: Forty-nine healthy individuals participated in this study and were randomly assigned to active tRNS (n = 24) and sham (n = 25) groups. tRNS was delivered for 20 minutes over Fp1/Fp2 and Oz. Resting-state EEG data were collected before and after either tRNS or sham stimulation. Results: Cluster-based permutation tests using FieldTrip revealed no frequency-specific effect of tRNS on resting-state EEG data across four frequency bands (theta, alpha, beta, gamma). Conclusions: These observations suggest that tRNS itself does not target or alter specific EEG frequencies. Rather, tRNS most likely interacts with the cognitive task/activity at hand to produce an observable difference in post-tRNS EEG. Positive tRNS-EEG findings from previous studies are also likely to have resulted from the interactive and cognitive activity-dependent nature of tRNS.
Transcranial electrical stimulation (tES) comprises several noninvasive techniques that can modulate neural activity. This is useful, as brain stimulation can go one step beyond neuroimaging to provide causal evidence of a brain region or a network of brain regions behind different cognitive functions. Three types of tES are currently used in cognitive research: transcranial direct current stimulation (tDCS), transcranial alternating current stimulation (tACS), and transcranial random noise stimulation (tRNS). Of these, tDCS is the most popular and has been demonstrated to improve cognitive functioning in many domains by increasing neural activity [1, 2, 3, 4, 5, 6, 7]. tACS, conversely, entrains neural activity in a frequency-specific manner and is often used in conjunction with a cognitive task to delineate the oscillatory mechanisms behind different cognitive functions [8, 9, 10, 11, 12, 13].
Among the three types of tES, tRNS is the least understood technique in cognitive neuroscientific research. Unlike tDCS or tACS, tRNS uses random frequency alternating current ranging from 0.1 to 640 Hz. With its biphasic characteristic but without a fixed frequency, tRNS can be viewed as a randomized variant of tACS. tRNS does not have a clear directionality for neural activity, unlike anodal and cathodal tDCS may have for neural excitation and inhibition, respectively. Because of its fast alternating frequency in the high-frequency range (e.g., 200 Hz), tRNS has also been hypothesized to operate via different mechanisms to tDCS and tACS [14, 15] that are normally assumed to be acting on neuronal oscillations and neural entrainment. Behaviorally, studies have shown that tRNS can produce facilitatory effects in a wide range of perceptual and cognitive tasks, such as emotion perception [16, 17], perception of facial identity [18], and perceptual learning [19, 20]. This is also true physiologically, as studies have demonstrated tRNS to be equal to or better than anodal tDCS in inducing large changes in excitability in motor evoked potential (MEP) [21, 22, 23, 24]. Furthermore, tRNS has been shown to induce less irritation on the scalp compared with tDCS, which makes it better-suited for single- or double-blind design [25]. However, the mechanisms of tRNS behind these promising behavioral effects remain elusive.
Why would a randomized, non-specific stimulation tool such as tRNS be able to
enhance human cognition? One idea that has been proposed to explain the positive
effects of tRNS is stochastic resonance [26, 27]. By adding random frequency
noises to near-threshold neural activities, subthreshold potentials could be
boosted to surpass the threshold and therefore increase the activity of the
neural network. Animal and in vitro studies have demonstrated that the
application of external electrical random noise stimulation can induce the
facilitation of Na
One potential explanation for the mixed finding in tRNS literature is that tRNS-induced enhancement might be task- or stimulation protocol-dependent. To test this, recent studies have begun combining electroencephalography (EEG) with tRNS to shed light on the mechanism behind the facilitatory effects of tRNS. For instance, tRNS on the auditory cortex has shown increased power in 40 Hz auditory steady-state responses (ASSR) [36]. One recent study has also demonstrated an improvement in acoustic perception with a decreased peak latency of the P50 and N1 components in event-related potentials (ERP) [37]. Stimulation of different cortical areas has also revealed modulation of cortical excitability. One study applying tRNS over the frontal cortex in the context of auditory selective attention was able to demonstrate reduced peak latency in P3 [38]. In addition, Harty and Cohen Kadosh [39] examined the effect of tRNS on sustained attention and found a reduction in the theta-to-beta ratio (TBR) when 1-mA tRNS was applied over the frontal cortex. Sheffield et al. [40] found improvement in arithmetic performance with increased N1 and P2 amplitude. Furthermore, participants with higher resting TBR showed better reaction time when tRNS was administered over the frontal cortex. Ghin et al. [41] demonstrated increased power spectral density (PSD) in the alpha and beta band when tRNS was administered to the visual cortex. Collectively, these EEG signatures reveal that, by modulating neural oscillatory activities, tRNS can effectively improve cognitive functioning. However, not all studies have observed an improvement [35, 42]. One specific example comes from the Dondé et al. [43] study, which utilized a randomized double-blind control design to investigate the correlation between the Stroop effect and resting state after bifrontal tRNS. The authors reported no significant change in behavioral performance and beta-to-alpha ratio. Furthermore, even the use of the same or similar stimulation parameters over the auditory cortex can still lead to inconsistent results [36, 44]. These results therefore suggest that the underlying mechanism of tRNS is unlikely to be simple frequency entrainment and needs to be further investigated.
Another complex aspect of tRNS findings comes from the fact that no two studies have used exactly the same task. As a result, it is difficult to disentangle whether a positive vs null result can be fully attributed only to the tRNS parameters, other experimental aspects such as task design, or the interaction between the two. For example, an increase in 40 Hz oscillation (such as those from Van Doren et al. [36]) in the active stimulation condition can either be a result of heightened intrinsic oscillations that is purely tRNS-induced (without task), or the oscillatory effect of heightened interaction between tRNS and task demand. To better elucidate this complex interaction, there is a need for a baseline condition, where task-free but tRNS-induced changes in EEG are clearly documented. This would in turn shed light on previous findings by clarifying which frequency bands can be boosted via tRNS alone, or via the interaction of tRNS and task.
To this end, in this study we compared resting-state EEG data before and after full spectrum tRNS. Our pre- and post-tRNS EEG data would shed light on how tRNS affects neural activities, without the confounding factors of simultaneous cognitive task/engagement. Once we have fully grasped the influence of tRNS over EEG frequencies in this relatively neutral context, future studies can compare their EEG data (with task) against our task-free EEG data to clarify the complex interactions between the different factors.
Forty-nine healthy participants were recruited in this study (15 males and 34 females; aged 20 to 30 years, mean age = 21.96 years). Before being recruited into this study, a safety screening was performed to identify and exclude any participants with contraindications to tRNS. None of the participants had neurological or psychiatric issues and none had taken psychoactive drugs in recent months. Participants were randomly assigned to the tRNS group (n = 24) or sham group (n = 25). All experimental procedures were approved by the Joint Institutional Review Board of Taipei Medical University, and all participants gave informed consent prior to their participation.
The experiment took approximately 2 hours to complete. Resting-state EEG data were collected before and after the stimulation on the same day. Participants were told to relax while EEG recording was in progress. EEG data were collected with eyes open for 3 minutes (fixating on a fixation cross in front) and closed for 3 minutes, for 3 cycles (18 minutes total). Participants then received 20 minutes of full spectrum tRNS or sham stimulation. Following that, another 18-minute post-stimulation resting-state EEG session, identical to the pre-stimulation session, was performed.
Electrical stimulation was applied using a StarStim stimulator (Neuroelectric,
Barcelona, Spain). Two 25 cm
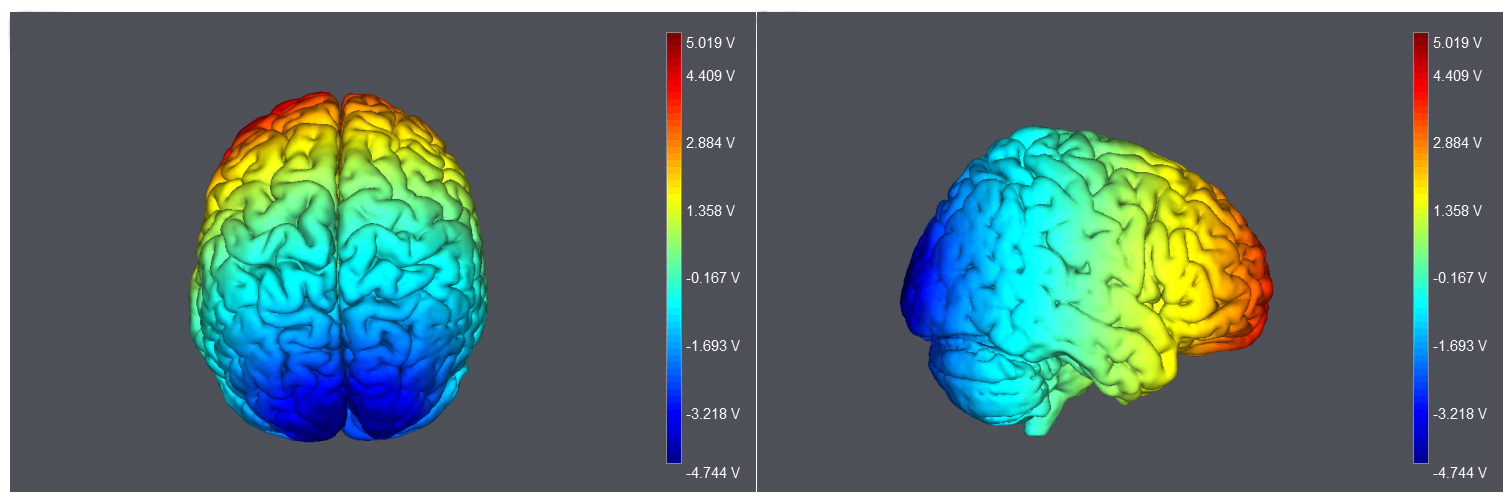
Montage and model of current flow. Electrodes were placed over the middle of Fp1/Fp2, and Oz. Modeling of current flow was produced using StarStim.
The EEG signals were continuously recorded from 32 Ag/AgCl electrodes (10/20
system) with a reference electrode between Fz and Cz. All electrodes were mounted
on a BrainCap electrode cap (Brain Products GmbH, Munich, Germany). All signals
were amplified using a BrainAmp amplifier (Brain Products GmbH, Munich, Germany)
and recorded using a BrainVision Recorder (version 1.22, Brain Products GmbH,
Munich, Germany). The signals were digitized at a sampling rate of 500 Hz. Two
sets of electrodes were placed on the upper and lower sides of the right eye, and
the canthi of both eyes, to measure participants’ vertical and horizontal eye
movements. Electrode impedance was maintained below 10 k
All preprocessing and analysis procedures were conducted using EEGLAB version
2021.1 [47] and custom MATLAB (R2020b; MathWorks Inc., Portola Valley, CA, USA)
scripts. Our preprocessing showed that data from the eyes-closed blocks were too
noisy, with ocular artifacts due to participants’ excessive eye movements, and
thus the eyes-closed blocks were excluded from further analysis. Eyes-open EEG
data were merged. An independent component analysis was performed to remove
ocular artifacts from EEG signals. The continuous ocular-corrected EEG data were
first offline and re-referenced to the average of the electrodes at the left and
right mastoids (A1 and A2). EEG data were then segmented into 2 second epochs.
Epochs with artifacts fluctuating over
A mixed analysis of variance (ANOVA) was conducted to test if power changes between pre- and post-stimulation occurred between the sham and tRNS groups in each frequency band. There was one within-subjects factor of time and one between-subjects factor of the stimulation group. Four scalp regions were chosen to perform the statistical analysis, creating the within-subjects factor of anterior-posterior electrodes: frontal (F3, Fz, and F4), central (C3, Cz, and C4), posterior (P3, Pz, and P4), and occipital regions (O1, Oz, O2). The Geisser–Greenhouse correction was applied when the assumption of sphericity was violated. Holm correction was applied for post-hoc tests. Findings from the ANOVA can be found in Supplementary Results.
In addition to spectral power, we measured phase synchronization between the two stimulation sites, Fz and Oz. The inter-site phase coherence is defined by
Where n represents the number of time points, and
We conducted a cluster-based permutation test as implemented in FieldTrip [48]
to investigate the effect of time (i.e., pre vs post) across four
frequency bands in two groups. Clusters had to extend over at least two adjacent
electrodes as a constraint. In each frequency band, a two-step statistical
analysis was performed, comparing pre- and post-stimulation effects across all
EEG channels and identifying spatial clusters with significant differences
(p
In terms of fronto-occipital synchronization, there was no main effect of time,
nor any interaction between time and group in theta, alpha, and beta (main effect
of time: theta: F(1,47) = 0.640, p = 0.428,
Previous studies including those from our own lab have shown variations in
individual receptivity to brain stimulation [7, 10, 49, 50]. Specifically, we have
repeatedly observed that low-performers (defined by the cognitive task of
interest from the sham session) tend to benefit from tDCS and tACS on their
cognitive performance, whereas high-performers do not. In the absence of a
cognitive task, this grouping procedure was not possible in the present study.
However, to examine whether there is any indication of variations in individual
receptivity to tRNS, we approximated each participant’s responsiveness to tRNS by
performing a “post – pre” contrast for all individual participants across all
frequencies. A positive difference would somewhat crudely indicate a positive
responder to tRNS. To this end, positive contrasts were observed in 16 out of 24
participants in overall power, 15 out of 24 participants in gamma band power, 16
out of 24 participants in beta band power, 14 out of 24 participants in alpha
band power, and 15 out of 24 participants in theta band power. Only 6 out of 24
participants showed full-range positive responses (i.e.,
In the present study, we found no frequency-specific effect of tRNS on human resting-state EEG data. This suggests that tRNS, although repeatedly shown to benefit human cognition in many contexts [51, 52, 53], likely derives its facilitative effect by interacting with task-specific brain states/neural dynamics. In other words, the facilitative effect of tRNS on human cognition is probably task-dependent in a specific manner, which is why many studies (that have used a range of different cognitive tasks) have reported very different frequency effects of tRNS in EEG.
If tRNS is highly interactive with cognitive tasks, one would reason that similar stimulation protocols coupled with different tasks may yield quite different results. Although there are no two studies with 100% identical parameters and task design, a review of some similar studies does support the idea of task-dependent, or task-interactive, tRNS outcome. Taking studies applying tRNS over the temporal cortex as an example, Rufener et al. [37] found that, when a near-threshold sound stimulus was used, temporal processing (using a gap detection task) could be improved with a reduced peak latency of P50 and N1, which approximates to the alpha-beta range in time frequency. In contrast, using similar stimulation parameters, Van Doren et al. [36] found increased power in 40 Hz ASSR instead. This discrepancy is likely attributable to the difference in tasks (auditory gap detection task vs ASSR) as the stimulation protocol is highly similar across both studies.
Under this framework, the interactive nature of tRNS can also be observed where tRNS reverses the oscillatory effect behind certain cognitive functions. For example, previous studies have revealed a negative correlation between task performance (e.g., attentional control and response inhibition) and TBR [54, 55]. Behzadnia, Ghoshuni and Chermahini [56] showed an increase in TBR when participants performed a visual version of the conjunctive continuous performance task compared with eyes-open resting EEG. However, one recent study applying frontal tRNS coupled with a continuous-monitoring task actually showed reduced TBR compared with sham stimulation [39]. tRNS-induced neuromodulation therefore likely depends on the task at hand and thus yields different (or opposite) results across studies.
Another potential interactant of tRNS outcome is individual receptivity. For example, Van Doren et al. [36] demonstrated 40 Hz ASSR increase after high-frequency tRNS over the temporal cortex. However, Schoisswohl et al. [44] could not replicate the same findings using both high and low-frequency tRNS. This suggests that tRNS may produce various effects between individuals. This idea is exemplified by a recent study by Rufener et al. [35], who gave participants either tRNS or white noise while participants performed an auditory detection threshold task. These authors observed considerable individual variation in their receptivity to tRNS, and the inverted U-shape function that is predicted by stochastic resonance account is only observed in some, but not all, participants. Our exploratory analyses on individual variations in different frequencies also support their conclusion.
Like tACS, one factor to consider is participant innate baseline activity (or cognitive performance). Harty and Cohen Kadosh [39] recently showed that people with higher TBR baseline could gain more benefit from tRNS over the right frontal and parietal cortex on a sustained attention task. They suggested that this might be due to the number of (relatively) more task-relevant subthreshold neurons that are excited by tRNS [39]. In contrast, people with high mathematic proficiency at baseline do not gain benefits from tRNS and may even show impaired performance in calculation tasks [57]. As such, it seems that tRNS-induced improvement may only be available to those whose neural or cognitive baseline falls within a specific range, and future studies should employ pre-stimulation baseline EEG or cognitive scores to investigate this possibility.
Stochastic resonance is a phenomenon that weak signals can be detected by adding random noise. In vitro studies also support the noise-enhanced mechanism by revealing that more action potentials are generated by adding appropriate noises in the dorsal root ganglion and pyramidal neurons [28, 29]. Applying adequate levels of noise by tRNS can optimize the signal-to-noise ratio in our neural system [58]. However, our results did not show such aftereffects of neural enhancement on EEG, suggesting that a task is important to induce the modulation of brain activity. This makes sense in our study because tRNS noise without any signal (i.e., task-induced activities) would end up being unhelpful noise. Our current observations from EEG are therefore consistent with the predictions of stochastic resonance account.
A study by Shalev et al. [59] showed that attentional selectivity can be boosted by combining bi-parietal tRNS with a spatial priming task. Interestingly, in their study, tRNS was no longer helpful when the spatial priming task was eliminated. Moreover, Cappelletti et al. [60] showed that the effect of tRNS is much smaller when parietal tRNS is applied alone without the aid of cognitive training. Taken together, we can infer that tRNS-induced modulation perhaps needs a specific task to maximize its effects. However, it is important to note that many recent studies have begun to question the validity of stochastic resonance in the context of tRNS and sensory/cognitive processing [35]. Although our null result is well predicted by stochastic resonance, more research is therefore needed to test how well stochastic resonance predicts other task-related tRNS effects.
Although the present study failed to find any tRNS-induced changes in resting state EEG, it remains possible that our study lacked the sample size or statistical power needed to detect the tRNS-EEG effect, if any. Caution is therefore required when considering null results as evidence for the absence of an effect. Nonetheless, our sample size (i.e., 24 in the tRNS condition, 49 in total) is on par with the sample sizes of most task-related tRNS studies, which have achieved significant cognitive-tRNS effects with sample sizes of 8 [30], 10 [25, 60], 14 [36], 15 [23, 24], 16 [17, 19, 41, 61], 17 [16], 18 [18], 19 [34], 20 [37, 38], and 22 [22, 44]. As such, we believe that if there really is an effect that we could not detect with our sample size, such effect (if any) is likely to have a much smaller effect size than those observed from previous task-related EEG studies. This would be consistent with our current conclusion that tRNS effect is more robust when a sensory/cognitive task is present.
Alternatively, it remains possible that other parameters (e.g., montage, current intensity) may be more successful in finding a tRNS-induced resting EEG effect. Although in this study we did try to match current intensity and ramp up/down time as did most previous studies, our choice of fronto-occipital montages (for the purpose of visualizing the current flow if there was an effect) is somewhat unconventional. To our knowledge, only one study has used this setup; Battaglini et al. [62] used fronto-occipital tRNS and observed increase arousal in a discriminative reaction time task (also see [63]). Thus, the effect was also task based in a fronto-occipital design. As such, it remains possible that different results might be obtained with another set of montages or parameters.
In summary, we tested which frequency bands were targeted or altered by tRNS. No significant power change across different frequency bands was observed after tRNS compared with sham stimulation, suggesting that positive findings from previous studies may be due to the interaction between tasks and stimulation. A specific task to induce such “baseline signals” (and its subsequent aftereffects) is crucial based on our findings and is consistent with the stochastic resonance account of tRNS. In addition, our exploratory analyses suggest that neurophysiological factors such as interindividual variability and baseline performance may also need to be considered. Future research on the neuromodulation effect of tRNS, either demonstrated by behavior or by EEG, should take this into account so that the use of tRNS can be optimized.
The datasets used and/or analyzed during the current study are available from the corresponding author on reasonable request.
SCK, YHL and PT designed the study. SCK and YHL collected and analyzed the data. All authors contributed to editorial changes in the manuscript. All authors read and approved the final manuscript. All authors have participated sufficiently in the work and agreed to be accountable for all aspects of the work.
This study was approved by the Joint Institutional Review Board of Taipei Medical University, Taiwan (N201509012). All participants gave informed consent prior to their participation.
Not applicable.
This research was supported in part by National Sciences and Technology Council of Taiwan (112-2410-H-002 -252, 109-2423-H-002-004 -MY4) and the Higher Education Sprout Project awarded to Taipei Medical University from the Ministry of Education, Taiwan.
The authors declare no conflict of interest.
Publisher’s Note: IMR Press stays neutral with regard to jurisdictional claims in published maps and institutional affiliations.