†These authors contributed equally.
Academic Editors: Brian Tomlinson and Takatoshi Kasai
Background: Acute myocardial infarction (AMI) is a common
cardiovascular disease that has a high mortality. Pyroptosis is a programmed cell
death mediated by inflammasome. It remains to be clarified on the expression
pattern and risk predictive role of pyroptosis-related genes in AMI.
Methods: The gene expression data were extracted from the Gene Expression
Omnibus (GEO), and pyroptosis-related genes were obtained from published
articles. Pyroptosis-related differential expressed genes were selected between
normal and AMI samples and then we explored their immune infiltration level using
CIBERSORT. Univariate Cox and LASSO regression were applied to establish a
classifier based on pyroptosis-related genes. ROC analysis was utilized to
evaluate the classifier. Results: In this study, we obtained 20
pyroptosis-related genes which showed differential expression in AMI and normal
samples. Among the differential expressed genes, GZMB was significantly
positively associated with activated NK cells (R = 0.71, p
Acute myocardial infarction (AMI) is the most prevalent disease with high mortality in the world. Statistically, it could result in a death toll accounting for 20% of all global deaths. It has an annually increasing incidence predicted to 0.44–1.42%, and causes 4.2–13.5% in-hospital mortality [1, 2]. For the past few years, multiple treatments, such as reperfusion, percutaneous coronary intervention (PCI) and anti-thrombotic, have brought survival benefits in this patient cohort and slightly decreased the related mortality [3, 4]. However, AMI remains a great threat to human health and imparts a large economic burden in the world [5]. Serum biomarkers, such as creatine kinase-MB, cardiac troponin I, and cardiac troponin T, are proven a diagnostic indicator for AMI of clinical significance [6, 7]. Nevertheless, they are not only lack of the predictive value, but also not perfectly specific biomarkers for AMI [8, 9]. Currently, there is still a lack of specific biomarkers capable of identifying patients at a high risk of AMI in early stages.
Pyroptosis is a type of pro-inflammatory regulated cell death dependent on gasdermin family of proteins [10]. It causes cell death by cell lysis, a result of impaired cellular osmotic pressure balance because of the formation of cell membrane pores, with the involvement of NLRP3/Caspase 1/GSDMD axis [11]. It is reported that pyroptosis plays a vital role in cardiovascular diseases, notably AMI. NLRP3 inflammasome and Caspase-1 are markedly up-regulating in AMI [12, 13]. At present, several studies have found that suppressing pyroptosis of cardiomyocytes can dramatically reduce myocardial infarction [14, 15]. Several recent studies have revealed the prognostic value of a novel pyroptosis-related genes signature in various diseases [16, 17].
Based on the existing studies, pyroptosis plays an essential role in the process of occurrence and development of the AMI. However, whether pyroptosis is predictive for the risk of AMI is largely unknown and requires further exploration. Hence, we firstly screened genes associated with pyroptosis from the AMI gene expression profile obtained from Gene Expression Omnibus (GEO) and then analyzed their association with infiltrating immune cells. A risk prediction classifier for AMI was devised. Finally, we detected the prediction accuracy through an external cohort, which may help better clinical application.
GSE59867 microarray documented with AMI gene expression data was downloaded from GEO database (https://www.ncbi.nlm.nih.gov/geo/). The microarray data were from GPL6244 platform (HuGene-1_0-st Affymetrix human genome 1.0 ST), including 390 AMI samples and 46 healthy control samples. GSE62646 microarray was additionally obtained as the validation set. Platform annotation files were downloaded to annotate Gene Symbol with Probe ID. Mean probe expression was regarded as a gene expression if the Gene Symbol could be annotated by multiple Probe IDs. In that way, gene expression profiles of the AMI and normal samples were obtained. Referring to existing literature [18] and the MSIGDB database (http://www.gsea-msigdb.org/gsea/msigdb/), 41 pyroptosis-related genes were searched and 38 of them were overlapped with the genes from GSE59867.
Pyroptosis-related genes were analyzed by “limma” package in AMI and normal
samples. Genes that met
The association between pyroptosis-related genes in AMI and all samples was analyzed by Pearson correlation analysis according to a Bubble Chart by R package “corrplot” and Scatter, Density Plots by package “ggplot”.
CIBERSORT is used to provide the absolute proportions of 22 infiltrating immune
cells in the immune microenvironment based on gene expression data. It is a type
of deconvolution algorithm with the support vector regression analysis in a set
of reference genes (n = 547) [20]. By this algorithm, each sample can be
conferred a p value (p
Univariate Cox analysis was devised to filter redundant genes from the DEGs.
LASSO regression model was established to further screen the remaining genes
[21], and the genes of significant predictive value for AMI were taken to analyse
in a multi-variate analysis. A riskscore was thus obtained and formulated
as:
With the sextile as the threshold, patients were respectively assigned to high and low risk groups. An external validation set was used to test the model, and receiver operating characteristic (ROC) curve was plotted to assess the predictive performance. To further illuminate the linkage mechanisms between AMI onset and pyroptosis-related genes, a figure was presented.
R v4.0.2(R Foundation for Statistical Computing, Vienna, Austria,
http://www.r-project.org/) and RStudio v1.2.1335 (Integrated Development for R.
RStudio, Inc., Boston, MA, USA, http://www.rstudio.com/) were operated to perform data
analysis. Significance evaluation was fulfilled by t-test or
Mann-Whitney test. Spearman correlation coefficient was calculated to identify
the associations between the pyroptosis-related genes and 22 infiltrating immune
cells. Cox proportional hazard regression model was established to estimate
hazards ratio (HR) along with 95% confidence interval (CI). ROC curve was made
to estimate the accuracy of the model in predicting the risk of AMI. All
differences on p
Gene expression profiles from the whole blood samples in 390 AMI cases and 46 healthy controls were obtained from the GSE59867 microarray. Following differential analysis and combining the 41 reported pyroptosis-related genes, finally 20 pyroptosis-related DEGs were identified, including 13 up-regulated genes (NLRP9, GSDMC, NLRC4, GSDMD, CASP9, ELANE, GPX4, NLRP3, NLRP6, NOD1, NOD2, PYCARD, GSDME) and 7 down-regulated genes (DHX9, GZMA, GZMB, GSDMB, CASP8, AIM2, SCAF11) (Fig. 1A). Fig. 1B shows the differential expression of the 20 DEGs in AMI and normal samples. Fig. 1C presents the heatmap of the 20 DEGs.
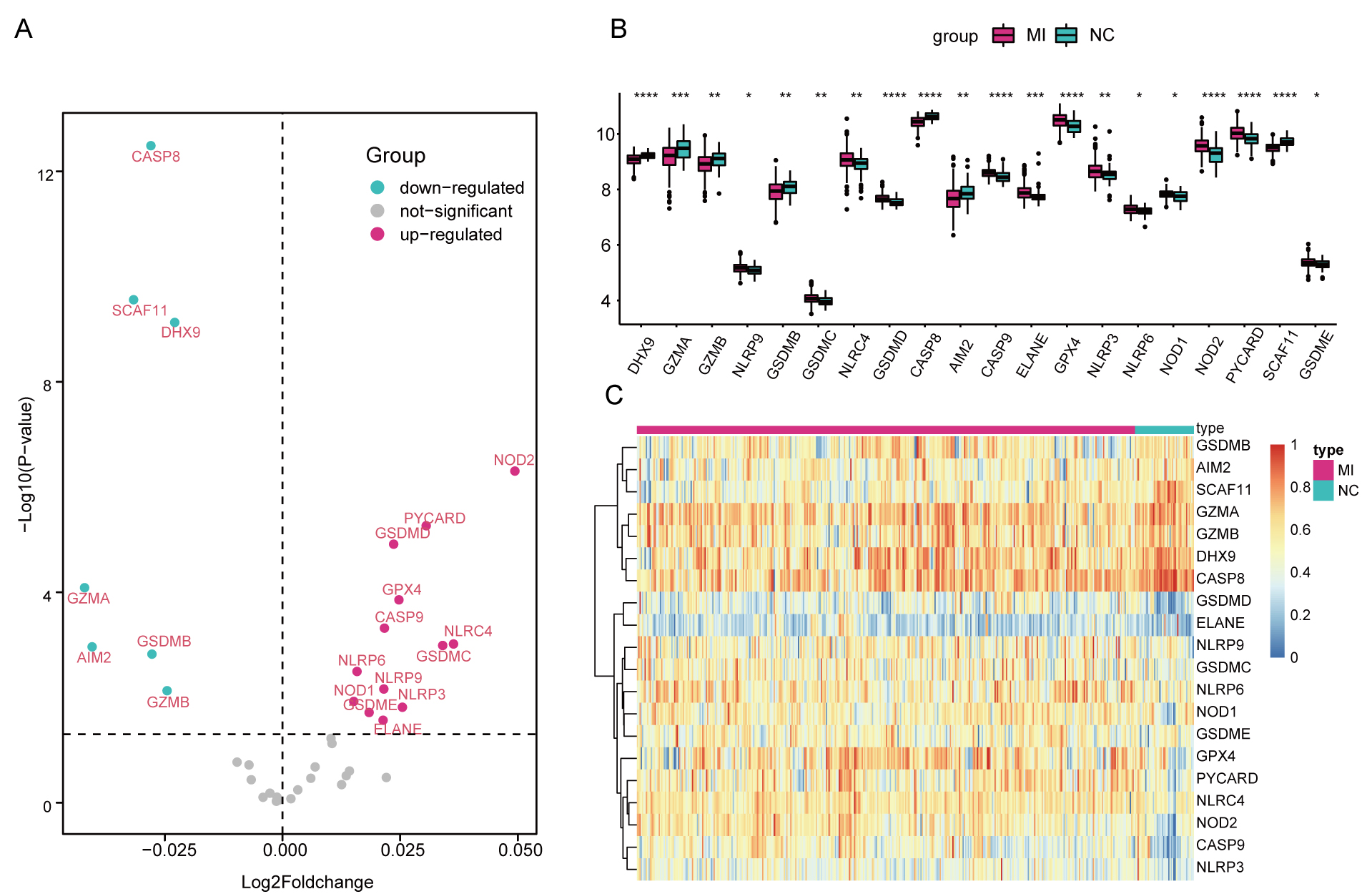
DEGs associated with pyroptosis in AMI. (A) Volcano Plot for
the DEGs. (B) Box Plots showing the differential gene expression in AMI and
normal samples. (C) Heatmap for the DEGs.
* p
The “corrplot” package was used to perform correlation analysis in the pyroptosis-related DEGs in both all included samples and AMI samples. Results revealed that GZMA and GZMB were significantly associated in both two cohorts (All: 0.71, AMI: 0.69) (Fig. 2).
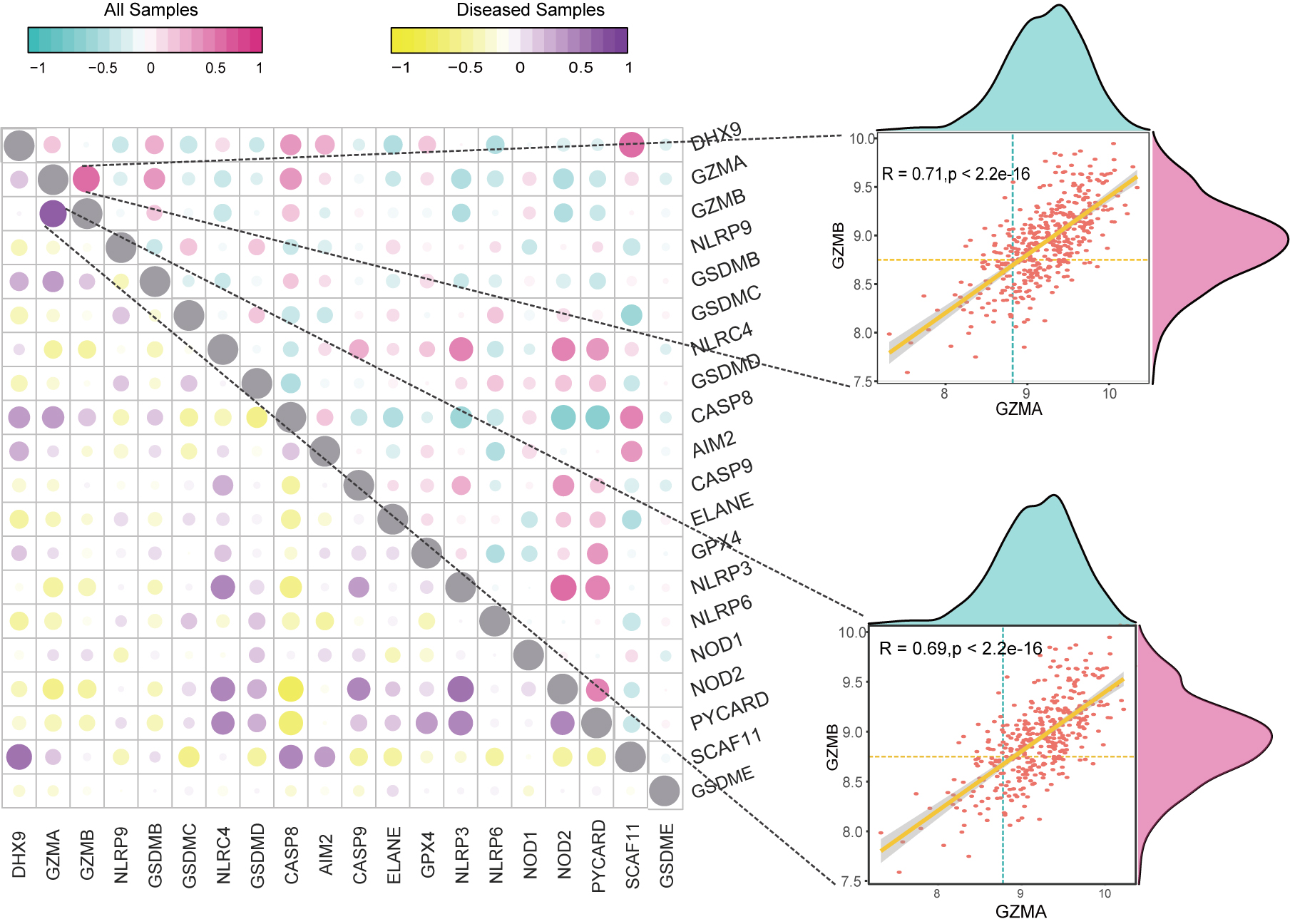
Correlation analysis on pyroptosis-related DEGs. Scatter Plot and Density Plot showing the correlation between GZMA and GZMB in all samples and AMI samples.
Plasma cells, T cells CD8, T cells follicular helper were found more abundant in
AMI samples versus the healthy control (p
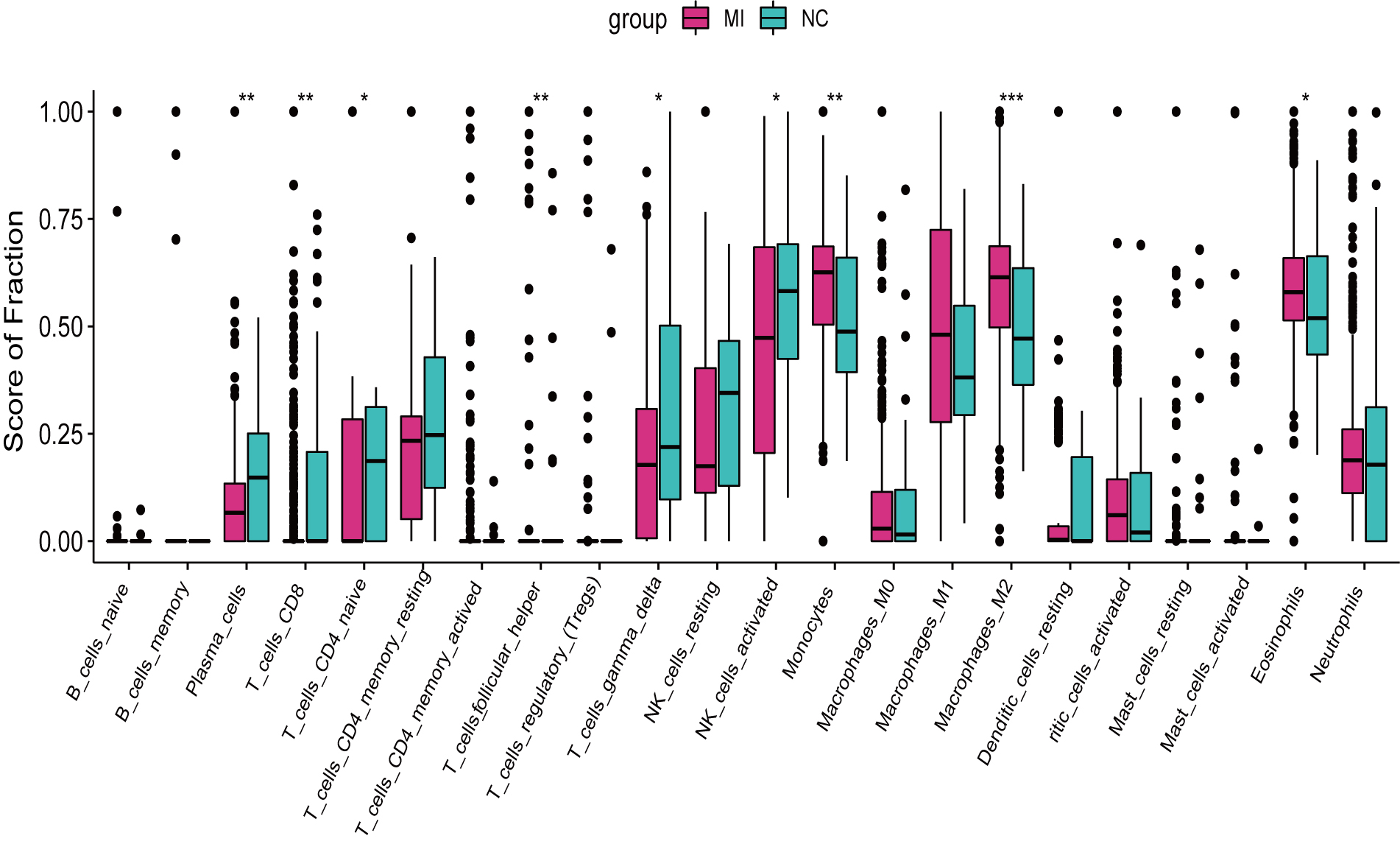
Infiltrating immune cells in AMI and normal samples. Red for
normal samples and Green for AMI samples. * p
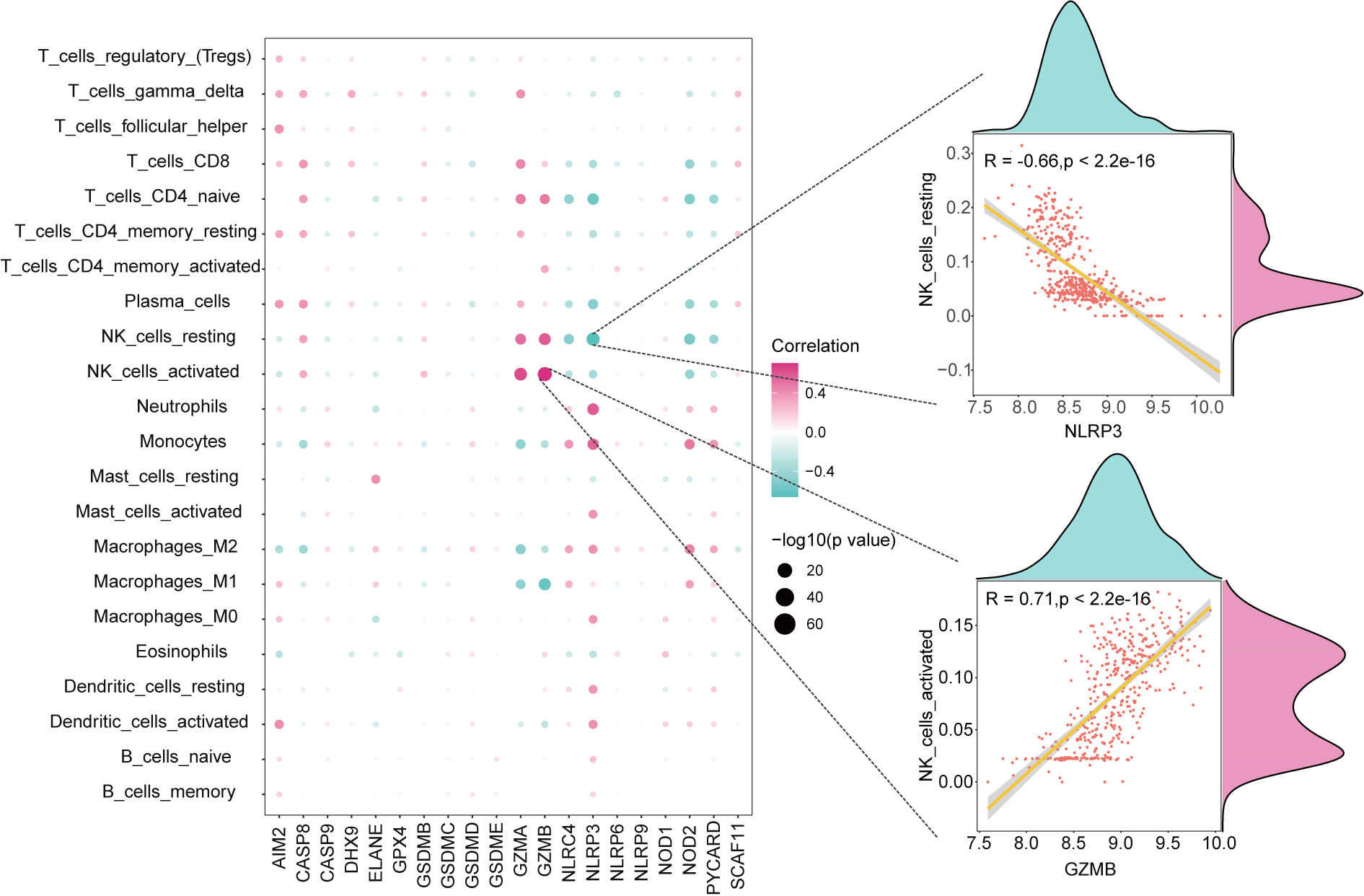
Correlation analysis between pyroptosis-related DEGs and immune cells. Scatter Plot and Density Plot showing the associations between GZMB and NK_cells_activated, NLRP3 and NK_cells_resting.
In the univariate analysis, 20 pyroptosis-related genes associated with AMI risk
were filtered (Fig. 5A). Following cross-validation in a LASSO regression model (Fig. 5B),
9 genes of vital significance were screened, including NLRP9, GSDMD,
CASP8, AIM2, GPX4, NOD1, NOD2, SCAF11, GSDME). Based on the 9 genes, a riskscore
was established (Fig. 5C). Further multivariate analysis presented that,
CASP8, AIM2, GPX4, NOD1, SCAF11, GSDME exhibited remarkable significance
in predicting the risk of AMI (Fig. 5D, all p
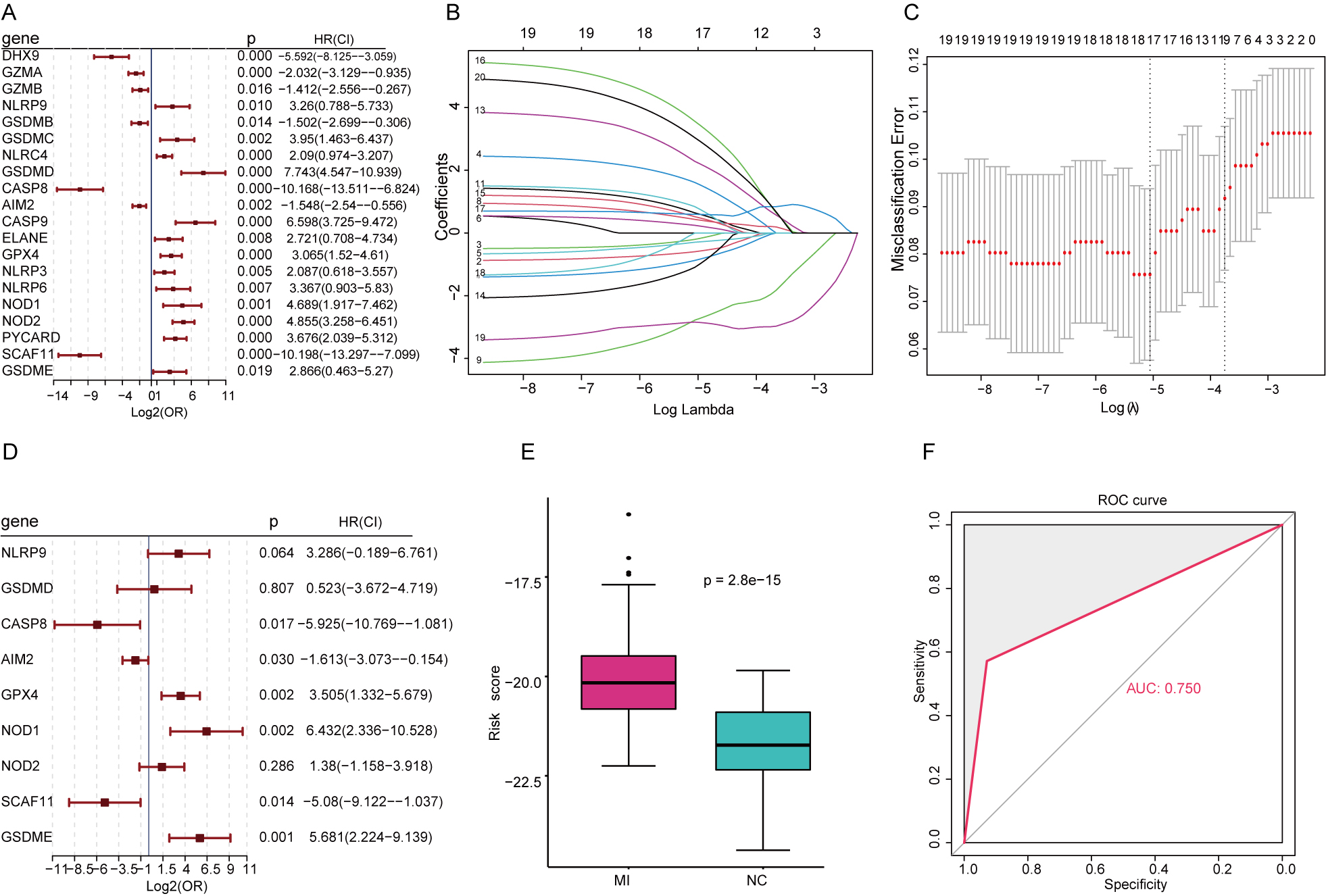
Comprehensive analysis on the predictive value of the pyroptosis-related risk model. (A) Univariate Cox regression analysis in pyroptosis-related DEGs. (B) LASSO regression analysis. (C) Cross-validation. (D) Multivariate analysis on significant genes. (E) Riskscore in AMI and normal samples. (F) External validation.
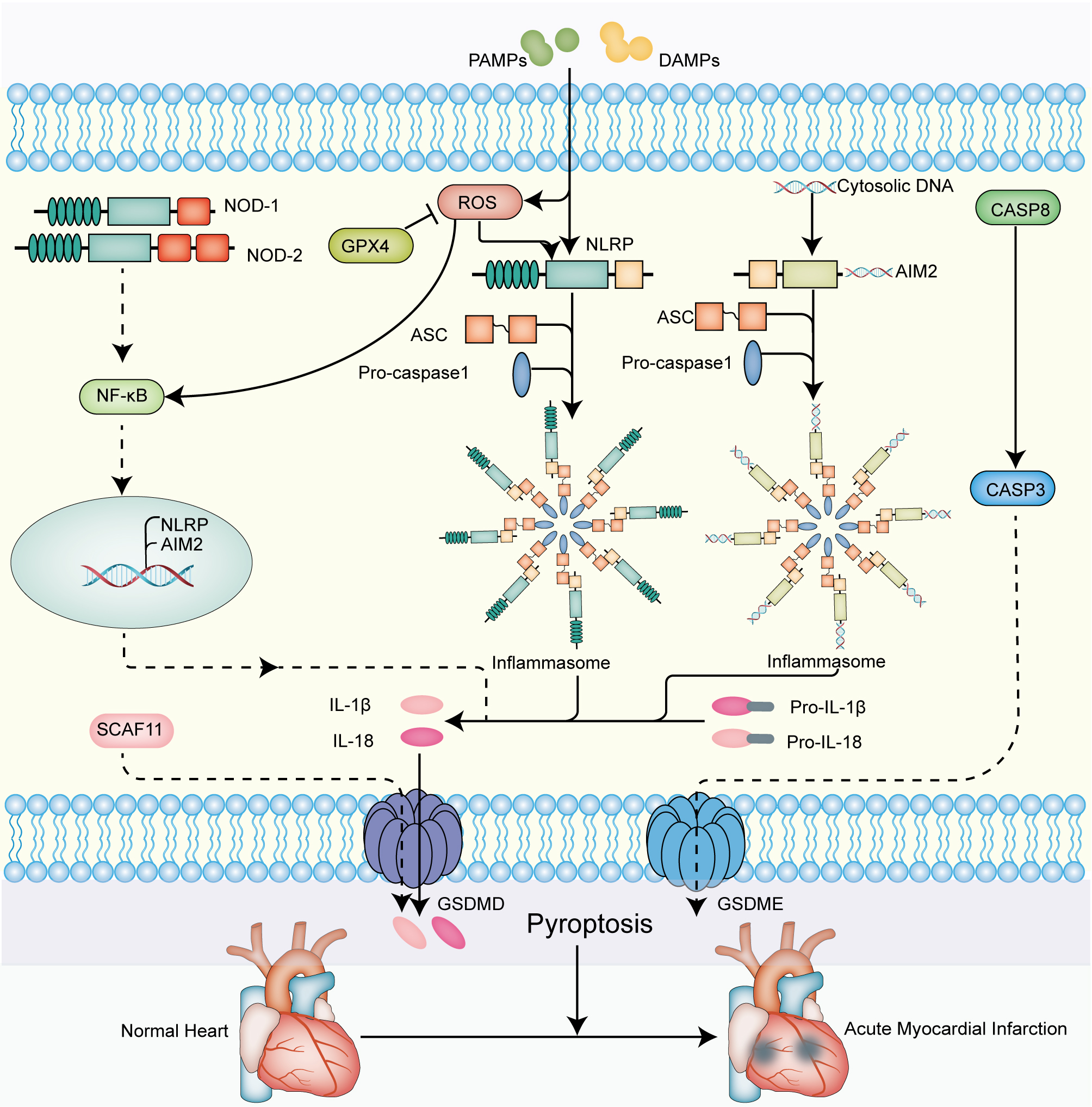
The molecular signaling mechanisms of
pyroptosis-related genes that mediate pathogenesis of AMI. On the one hand, the
activation of the intracellular NLRP family is provoked by the various
pathogen-associated molecular patterns (PAMPs) and damage-associated molecular
patterns (DAMPs), leading to the formation of the inflammasome. On the other
hand, the activation of NLRP inflammasome is regulated by ROS, whereas
GPX4 suppresses the generation of ROS. Ultimately, the results in releasing of
mature IL-1
AMI is an irreversible damage to myocardium driven by coronary artery thrombosis or occlusion [23]. Recent studies demonstrated that pyroptosis was involved in the pathogenesis of cardiac injury in AMI [24, 25]. Moreover, Inhibition of pyroptosis can cut down myocardial infarct size [26]. In the study, we found that pyroptosis-related genes were highly correlated with infiltrating immune in AMI. In addition, a 9 pyroptosis-related gene based on predictive model for AMI risk was constructed, and identified to have favorable performance in discriminating between high and low risk patients in an external validation set.
A growing number of studies have demonstrated the crucial role of infiltrating
immune cells in patients with cardiovascular disease, such as neutrophils,
monocyte macrophages and helper T1 cells [27, 28, 29, 30]. It remains to be clarified on
the abundance of the immune cells in AMI patients. Here, CIBERSORT algorithm was
applied to find higher proportions of Plasma cells, T cells CD8 and T cells
follicular helper in AMI group versus the healthy control group, which is
consistent with the existing studies [31, 32]. On T cells follicular helper,
currently there has no relevant report yet and its clinical application value
requires further investigation. It was reported that there is certain
relationship between cell pyroptosis and immune system function. By releasing
TNF-
The predictive significance for AMI was composed of 9 pyroptosis-related genes,
including NLRP9, GSDMD, CASP8, AIM2, GPX4, NOD1, NOD2, SCAF11, GSDME.
The use of an external validation set further proved the good performance of the
9-gene signature in predicting the risk of AMI. NLRP9 (NLR Family Pyrin
Domain Containing 9) is a member of the NLRs family that comprises a leucine-rich
repeat (LRR), nucleotide-bindingoligomerization domain (NACHT), and a caspase
activation andrecruitment domain (CARD) or a pyrindomain (PYD). As reported,
NLRP9 under inflammation would exhibit stronger expression [39], while
knock out of NLRP9 gene could suppress inflammatory reactions and
decrease tissue injury [40]. NOD (Nucleotide Binding Oligomerization Domain),
including NOD1 and NOD2, shares similar structures to
NLRP9 and exerts vital effects during the occurrence of atherosclerosis and
further progression to AMI. In an animal experiment, mice of NOD1/2
knock-out presented with less lipid deposition and macrophage aggregation as
compared to the normal mice [41]. AIM2 (Absent In Melanoma 2) contains a
N-terminal PYD signal domain and a C-terminal HIN200 domain, and it can recognize
double-stranded DNA. Research revealed that activation of AIM2 inflammasome could
aggravate atherosclerosis [42]. The NLRP inflammasome is capable of bringing on
the activation of caspase in pyroptosis. Caspase-8 is a
cysteinate-aspartate specific protease and one of the major initiators involved
in cell pyroptosis. It would show increasing expression with the occurrence of
AMI [43], which is in agreement with the finding of the present study. In cases
of myocardial ischemia reperfusion (I/R), Caspase-8 could increase
myocardial NO oxidation and mitochondrial reactive oxygen species (ROS), while
the ROS could further mediate pyroptosis via the NF-
There have been multiple biomarkers available for diagnosis and prognosis of AMI. Wang et al. [54] performed weighted gene co-expression network analysis to study potential AMI-related biomarkers. Zhang et al. [55] identified 4 key genes which were capable of diagnosing the plaque changes in recurrent AMI. Nevertheless, there is still a gap on biomarkers predictive for the risk of AMI. Distinct features of AMI were a rapid onset and high mortality. Early revascularization can greatly reduce myocardial injury and the mortality for patients. Thus, early identification and prevention for AMI are extremely important to reduce the incidence and mortality, while improving patient prognosis. In this study, we first conducted a prognostic classifier depending on pyroptosis-related genes in AMI. A previous study found that a novel pyroptosis-related gene signature had been remarkably effective to predict the prognosis of uveal melanoma [56]. Similarly, pyroptosis-related risk model had been used in order to predict the prognosis of Hepatocellular carcinoma [57]. In the present study, pyroptosis-related gene signature showed promising value in predicting the risk for AMI, moreover, which has a better value as a predictor of AMI through ROC analysis than others [58, 59, 60, 61, 62]. The model formula established in this study is simple and easy to implement. Intelligent and accurate early warning of AMI is achieved mainly by collecting blood specimens from people and measuring the gene expression profiles, which will provide a reliable reference basis for clinical decision-making and bring excellent convenience.
This study also has some limitations and shortcomings. First, this study is devised retrospective based on public databases and only one external set was used for validation. In the future, larger clinical sample data are in demand to support the finding of the study. Second, in despite of the good predictive performance of the pyroptosis-related genes we found here, there is paucity of studies on pyroptosis, and the specific biological effects in AMI are largely unknown. Third, the model we established lacks further validation, and in vivo and in vitro experiments as well as clinical trials are required.
In all, this study uncovered the close relationship between pyroptosis and AMI, and further identified the association between pyroptosis and immune response. The predicting model was built based on pyroptosis-related differential genes. It may provide a new way for predicting the risk of AMI, which has potential clinical value.
AMI, acute myocardial infarction; DEGs, differential expressed genes; GEO, Gene Expression Omnibus; LRR, leucine-rich repeat; NACHT, nucleotide-bindingoligomerization domain; CARD, caspase activation andrecruitment domain; PYD, pyrindomain; ROS, reactive oxygen species.
QXM, HC and WHS designed the research study. HHW, QY, JWL and RJ collected and assembled of data. KHG and ZWZ analyzed the data. KHG, ZWZ and PFC wrote the manuscript. All authors contributed to editorial changes in the manuscript. All authors read and approved the final manuscript.
Publicly available datasets were downloaded and analyzed in this study. The patients involved in the database have obtained ethical approval. Informed consent and ethical approval were not required for this study in accordance with the national legislation and the institutional requirements.
Not applicable.
This study was supported by grants from the National Natural Science Foundation of China (82171698, 82170561, 81741067, 81300279), the Natural Science Foundation for Distinguished Young Scholars of Guangdong Province (2021B1515020003), the Climbing Program of Introduced Talents and High-level Hospital Construction Project of Guangdong Provincial People’s Hospital (DFJH201803, KJ012019099, KJ012021143, KY012021183).
The authors declare no conflict of interest.