- Academic Editors
Background: To develop and validate a nomogram prediction model for
assessing the risk of major adverse cardiovascular and cerebrovascular events
(MACCE) in patients with nonvalvular atrial fibrillation (NVAF) and heart failure
with preserved ejection fraction (HFpEF) within one year of discharge.
Methods: We enrolled 828 patients with NVAF and HFpEF from May 2017 to
March 2022 in Zhongda Hospital as the training cohort, and 564 patients with NVAF
and HFpEF in Taizhou People’s Hospital between August 2018 and March 2022 as the
validation cohort. A total of 35 clinical features, including baseline
characteristics, past medical records, and detection index, were used to create a
prediction model for MACCE risk. The optimized model was verified in the
validation cohort. Calibration plots, the Hosmer-Lemeshow test, and decision
curve analyses (DCA) were utilized to assess the accuracy and clinical efficacy
of the nomogram. Results: MACCE occurred in 23.1% of all patients
within one year of discharge. The nomogram identified several independent risk
factors for MACCE, including atrial fibrillation duration
Nonvalvular atrial fibrillation (NVAF) is a common heart condition that is associated with adverse outcomes in heart failure with preserved ejection fraction (HFpEF) [1, 2]. Pathogenesis, treatment, and prognosis of valvular atrial fibrillation (AF) differ significantly from NVAF [3]. For the purpose of this discussion, we will focus solely on NVAF combined with HFpEF. Patients with AF rhythm, compared to those with HFpEF in sinus rhythm, exhibit pronounced atrial dysfunction and a substantially elevated risk of cardiovascular and cerebrovascular events [4]. Persistent AF, along with other pathophysiological changes associated with the condition, may contribute to a distinct clinical AF phenotype in HFpEF; however, the optimal approach to treating and preventing AF in HFpEF is unclear. There have been many recent developments in radiofrequency ablation, anticoagulation, and rhythm control of AF [5, 6, 7]. However, their therapeutic effect on patients with HFpEF and AF remains limited, and the likelihood of cardiovascular and cerebrovascular events remains elevated in patients with HFpEF and AF compared to those without heart failure (HF) with AF. Consequently, there is a need for an individualized and practical prediction model based on the available clinical data. Nomogram fulfills this unmet need as a user-friendly prediction model to facilitate patient management and decision-making. ThereforeIn this paper we present a nomogram, the first prediction model for estimating the risk of major adverse cardiovascular and cerebrovascular events (MACCE) in patients with NVAF and HFpEF within one year of discharge.
This multicenter retrospective study enrolled 861 consecutive patients with NVAF and HFpEF from May 2017 to March 2022 in Zhongda Hospital. Additionally, the validation cohort included 586 patients with NVAF and HFpEF recruited at the Taizhou People’s Hospital between August 2018 and March 2022. All participants in the MACCE group experienced cardiovascular and cerebrovascular events within one year of discharge. This study was approved by the Ethics Committee of Zhongda hospital and Taizhou people’s Hospital, respectively. Additional details can be found in Fig. 1.
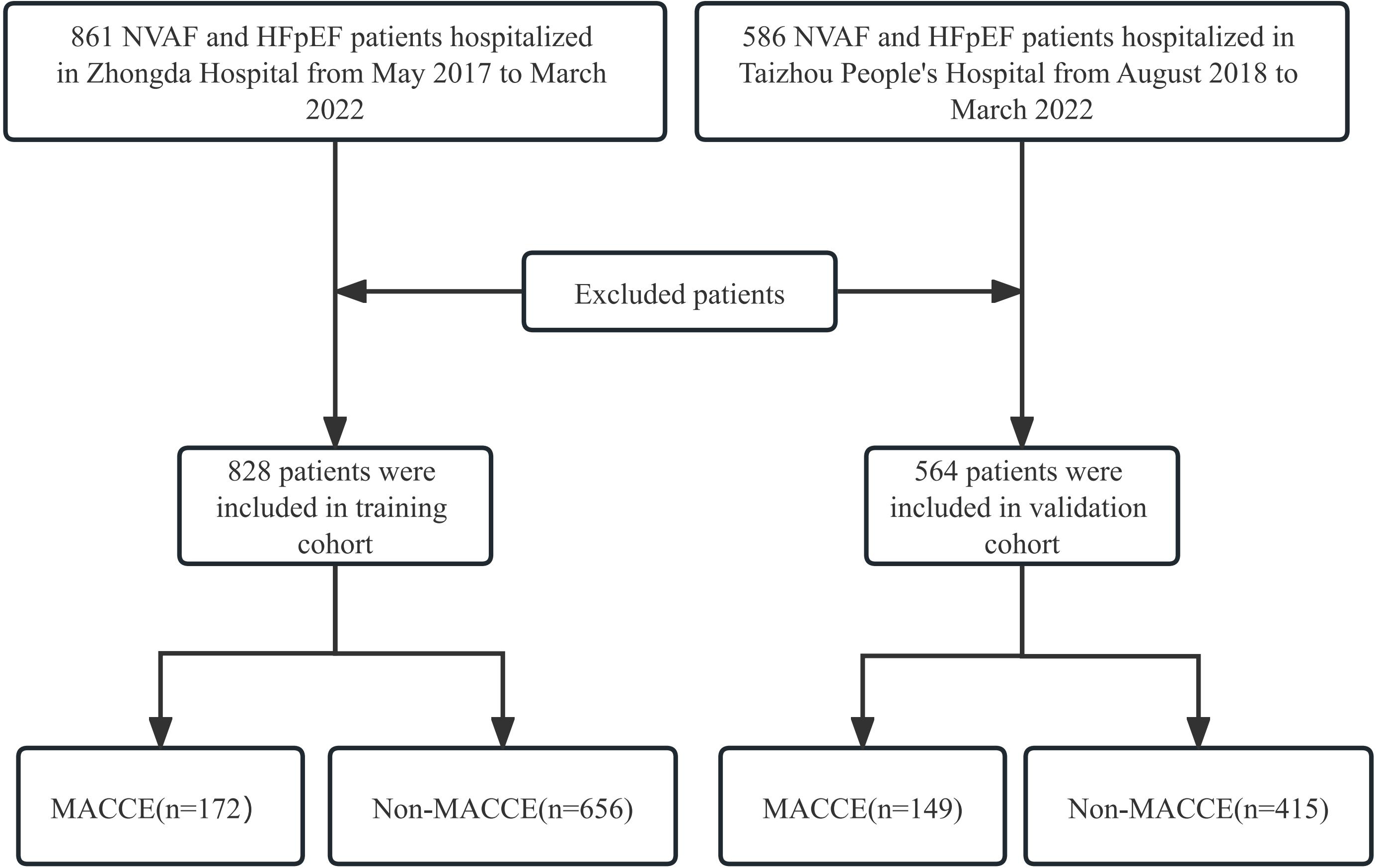
Flowchart of patient selection. HFpEF, heart failure with preserved ejection fraction; MACCE, major adverse cardiovascular and cerebrovascular events; NVAF, nonvalvular atrial fibrillation.
The diagnostic criteria for NVAF [8] and HFpEF [9] set forth by the European Society of Cardiology were adhered to in this study. Patients meeting any of the following conditions were excluded from the study: (1) individuals with coronary artery disease who had undergone coronary artery bypass grafting or had received three or more coronary stents; (2) patients with severe congenital heart diseases such as pulmonary stenosis, large atrial septal defect, ventricular septal defect, aortic coarctation, patent ductus arteriosus, or tetralogy of Fallot; (3) individuals with severe end-stage diseases affecting vital organs, including acute or chronic liver failure, renal failure requiring blood purification, previous extensive cerebral infarction, or hemorrhage; (4) patients with malignant clonal diseases such as leukemia, lymphoma, or malignant solid tumors; and (5) individuals lost to follow-up.
The following demographic data were collected: sex, age, body mass index,
smoking history, systolic blood pressure, diastolic blood pressure,
classification of AF, duration of AF, ablation therapy of AF, and poor medication
compliance indicated by a score of less than 6 on the score of Medication
Adherence Report Scale [10]. Additionally, we collected information the
administration medications including angiotensin-converting enzyme
inhibitors/angiotensin receptor antagonists, beta-blockers, mineralocorticoid
receptor antagonists, angiotensin-receptor neprilysin inhibitors, and
sodium-glucose co-transporter-2 (SGLT-2) inhibitors. The presence of
comorbidities including hyperthyroidism, hypertension, coronary artery disease,
stroke, and chronic obstructive pulmonary disease, was also documented.
Lab tests were performed to measure serum levels of hemoglobin,
low-density lipoprotein cholesterol, total cholesterol, uric acid, serum
creatinine (Scr), estimated glomerular filtration rate (eGFR), serum potassium
(K
cEDS, the circumferential end-diastolic stress; DBP, diastolic blood pressure; LVIDs, left ventricular internal diameters; PWTs, Posterior wall thicknesses.
During the follow-up period, every patient was contacted via a phone call to ascertain the occurrence of MACCE. The primary outcome of follow-up was the occurrence of MACCE within one year of discharge [12], including all-cause death, nonfatal acute myocardial infarction, and nonfatal stroke.
Statistical analysis was performed using SPSS 20.0 (IBM, Inc., Chicago, IL, USA)
and R (version 3.6.2; R Foundation for Statistical Computing, Vienna, Austria).
The mean and standard deviation of continuous variables were calculated and
compared using the unpaired Student’s t-test or Mann-Whitney U-test
while categorical variables were compared using the
The final model to predict the occurrence of MACCE in patients with NVAF and
HFpEF after discharge was developed using the rms package of R (version 3.6.2, R
Foundation for Statistical Computing, Vienna, Austria). The performance of the
final predictive model was evaluated by the calibration plot, Hosmer-Lemeshow
test, and decision curve analysis (DCA) using the validation cohort (developed
with the R packages of rms and rmda). All statistical tests were two-tailed;
p
A total of 1392 patients were enrolled in the study, with 828 from Zhongda Hospital and 564 from Taizhou People’s Hospital. Out of these, 55 patients (33 from the training cohort and 22 from the validation cohort) were excluded from the study. There were no significant differences in the baseline data, including age, sex, body mass index, systolic blood pressure, diastolic blood pressure, hypertension, and stroke, between the MACCE and non-MACCE cohorts, except for the Scr and hemoglobin A1c levels, LVEDd, and cEDS. Detailed data analysis is shown in Table 1. The proportion of patients with MACCE in the training and validation cohorts was 20.8% and 26.4%, respectively. In the training cohort, 83 patients experienced nonfatal stroke, 37 patients experienced nonfatal myocardial infarction, and the remaining 52 patients experienced death from all-causes. In the validation cohort, 66 patients experienced nonfatal stroke, 29 patients experienced nonfatal myocardial infarction, and the remaining 54 patients experienced death from all-causes.
Characteristic | Training cohort | p | Validation cohort | p | |||
MACCE | Non-MACCE | MACCE | Non-MACCE | ||||
(n = 172) | (n = 656) | (n = 149) | (n = 415) | ||||
Male (%) | 99 (57.6) | 373 (56.9) | 0.87 | 81 (54.4) | 229 (55.2) | 0.86 | |
Age, years, mean (SD) | 73.51 (7.81) | 74.37 (8.55) | 0.23 | 73.45 (8.24) | 72.85 (7.16) | 0.40 | |
BMI |
93 (54.1) | 304 (46.3) | 0.07 | 88 (59.1) | 217 (52.3) | 0.16 | |
SBP, mmHg, mean (SD) | 149.2 (15.9) | 147.6 (17.3) | 0.27 | 147.6 (16.3) | 146.3 (15.7) | 0.39 | |
DBP, mmHg, mean (SD) | 84.4 (9.3) | 85.7 (9.8) | 0.12 | 85.9 (8.8) | 86.5 (9.1) | 0.49 | |
Classification of AF | 0.32 | 0.97 | |||||
Paroxysmal AF, n (%) | 32 (18.6) | 112 (17.1) | 36 (24.2) | 93 (22.4) | |||
Persistent AF, n (%) | 35 (20.3) | 114 (17.4) | 31 (20.8) | 86 (20.7) | |||
Long-term persistent AF, n (%) | 66 (38.3) | 235 (35.8) | 42 (28.2) | 123 (29.6) | |||
Permanent AF, n (%) | 39 (22.7) | 195 (29.7) | 40 (26.8) | 113 (27.2) | |||
Duration of AF |
89 (51.7) | 198 (30.2) | 81 (54.4) | 152 (36.6) | |||
Ablation therapy of AF, n (%) | 57 (33.1) | 201 (30.6) | 0.53 | 73 (49.0) | 174 (41.9) | 0.14 | |
Smoke, n (%) | 38 (22.1) | 131 (20.0) | 0.54 | 36 (24.2) | 124 (29.9) | 0.18 | |
Hyperthyroidism, n (%) | 41 (23.8) | 53 (8.1) | 41 (27.5) | 78 (18.8) | 0.03 | ||
Hypertension, n (%) | 124 (72.1) | 505 (77.0) | 0.18 | 112 (75.2) | 282 (68.0) | 0.10 | |
CAD, n (%) | 98 (57.0) | 335 (48.3) | 0.17 | 62 (41.6) | 157 (37.8) | 0.42 | |
Stroke, n (%) | 60 (34.9) | 197 (30.0) | 0.22 | 39 (26.2) | 124 (29.9) | 0.39 | |
COPD, n (%) | 25 (14.5) | 78 (11.9) | 0.35 | 13 (8.7) | 45 (10.8) | 0.47 | |
Poor medication compliance, n (%) | 73 (42.4) | 164 (25.0) | 46 (30.9) | 87 (21.0) | 0.02 | ||
ACEI/ARB, n (%) | 101 (58.7) | 393 (59.9) | 0.78 | 59 (39.6) | 153 (35.7) | 0.56 | |
Beta-blocker, n (%) | 74 (43.0) | 269 (41.0) | 0.63 | 66 (44.3) | 186 (44.8) | 0.91 | |
MRA, n (%) | 123 (71.5) | 492 (75.0) | 0.35 | 97 (65.1) | 265 (63.9) | 0.79 | |
Antiplatelet, n (%) | 139 (80.8) | 511 (77.9) | 0.41 | 115 (77.2) | 311 (74.9) | 0.59 | |
DOACs, n (%) | 71 (41.3) | 319 (48.6) | 0.09 | 60 (40.3) | 186 (44.8) | 0.34 | |
VKAs, n (%) | 29 (16.9) | 77 (11.7) | 0.07 | 21 (14.1) | 54 (13.0) | 0.74 | |
ARNI, n (%) | 58 (33.7) | 196 (29.9) | 0.33 | 41 (27.5) | 137 (33.0) | 0.22 | |
SGLT-2 inhibitor, n (%) | 48 (27.9) | 268 (40.9) | 52 (34.9) | 193 (46.5) | 0.01 | ||
Hb, g/L, mean (SD) | 115.28 (9.67) | 116.42 (10.31) | 0.19 | 117.63 (12.69) | 118.32 (10.67) | 0.52 | |
LDL-C, mmol/L, mean (SD) | 4.24 (1.81) | 3.99 (1.78) | 0.10 | 4.38 (1.38) | 4.42 (1.54) | 0.78 | |
TC, mmol/L, mean (SD) | 5.84 (2.13) | 6.03 (2.25) | 0.32 | 5.74 (1.79) | 5.41 (1.88) | 0.06 | |
UA, µmol/L, mean (SD) | 439.41 (122.52) | 441.66 (128.9) | 0.84 | 447.12 (115.38) | 432.85 (132.08) | 0.24 | |
Scr, µmol/L, mean (SD) | 147.23 (66.91) | 135.45 (58.37) | 0.02 | 153.65 (40.19) | 118.55 (38.06) | ||
eGFR, mL/(min |
55.79 (11.35) | 58.15 (14.88) | 0.05 | 56.95 (12.12) | 59.13 (13.65) | 0.09 | |
K |
3.89 (0.73) | 3.77 (0.87) | 0.10 | 4.04 (0.61) | 3.98 (0.67) | 0.34 | |
Na |
129.42 (9.65) | 130.06 (10.43) | 0.47 | 131.86 (8.27) | 130.35 (9.82) | 0.09 | |
HbA1c, %, mean (SD) | 8.22 (2.07) | 7.78 (2.14) | 0.02 | 8.58 (1.82) | 7.21 (1.74) | ||
NT-proBNP, pg/mL, mean (SD) | 7291.5 (2381.4) | 6829.6 (2127.9) | 0.01 | 7568.1 (2154.7) | 6472.6 (1848.0) | ||
LVEF, %, mean (SD) | 61.3 (7.4) | 62.6 (8.6) | 0.07 | 63.7 (7.5) | 65.1 (9.7) | 0.11 | |
LVEDd, mm, mean (SD) | 48.14 (6.52) | 46.86 (6.39) | 0.02 | 48.54 (5.48) | 45.05 (6.13) | ||
cEDS, kdyne/cm |
42.97 (9.21) | 38.49 (10.63) | 43.55 (10.19) | 36.43 (8.71) |
MACCE, major adverse cardiovascular and cerebrovascular events; ACEI/ARB,
angiotensin converting enzyme inhibitor/angiotensin receptor antagonist; AF,
atrial fibrillation; ARNI, angiotensin-receptor neprilysin inhibitor; BMI, body
mass index; cEDS, circumferential end-diastolic stress; COPD, chronic obstructive pulmonary disease; DBP, diastolic blood
pressure; eGFR, estimated glomerular filtration rate; Hb, hemoglobin; HbA1c,
hemoglobin A1cl; K
The training cohort underwent least absolute shrinkage and selection operator
(LASSO) regression analysis to identify predictors for the nomogram. Among the
predictors screened, six variables demonstrated the strongest correlation with
the occurrence of MACCE in patients with NVAF and HFpEF within one year of
discharge in the training cohort (Fig. 2). These predictors included duration of
AF of
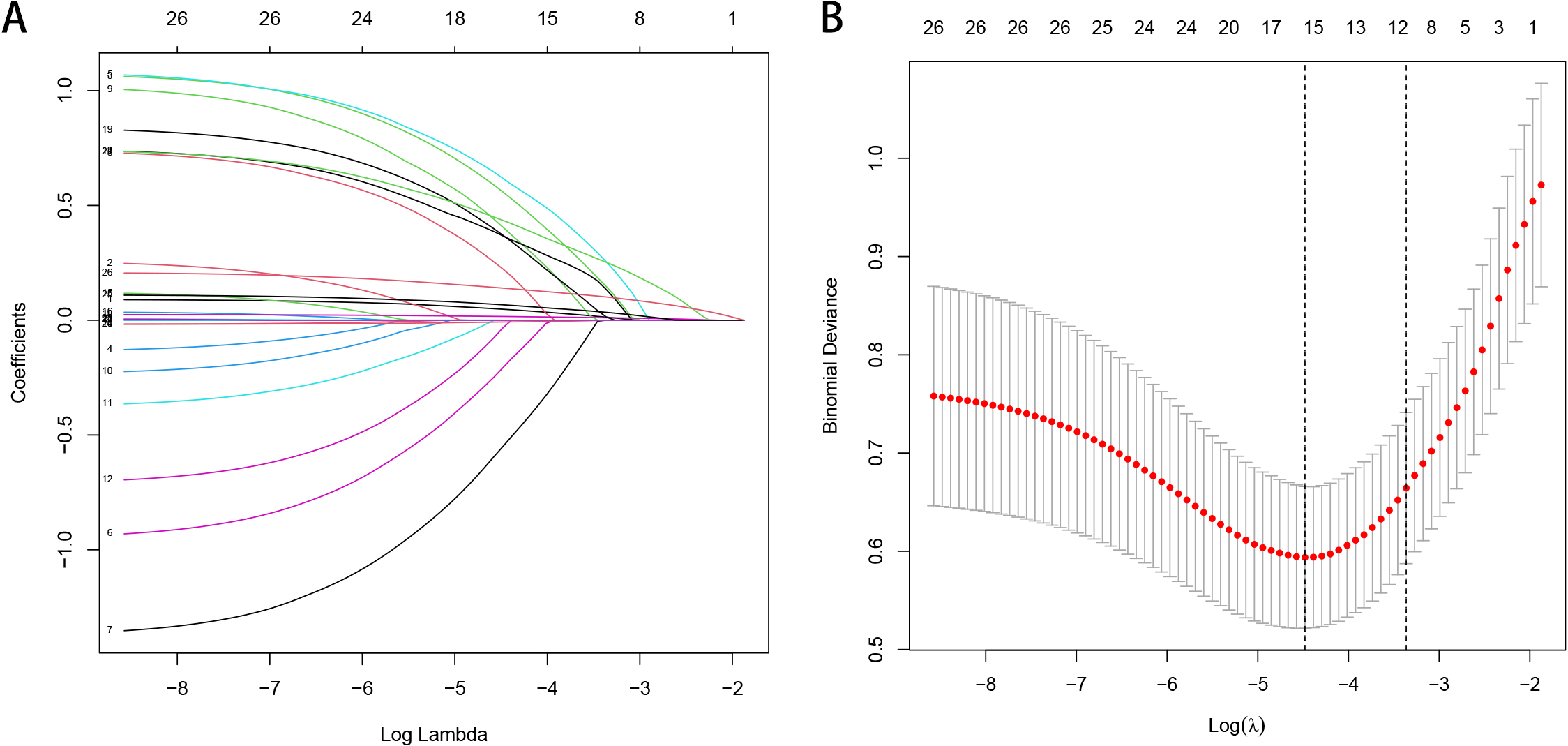
Feature selection via the LASSO binary logistic regression
model. (A) LASSO coefficient profiles of the 24 texture features. A coefficient
profile plot was produced against the log (
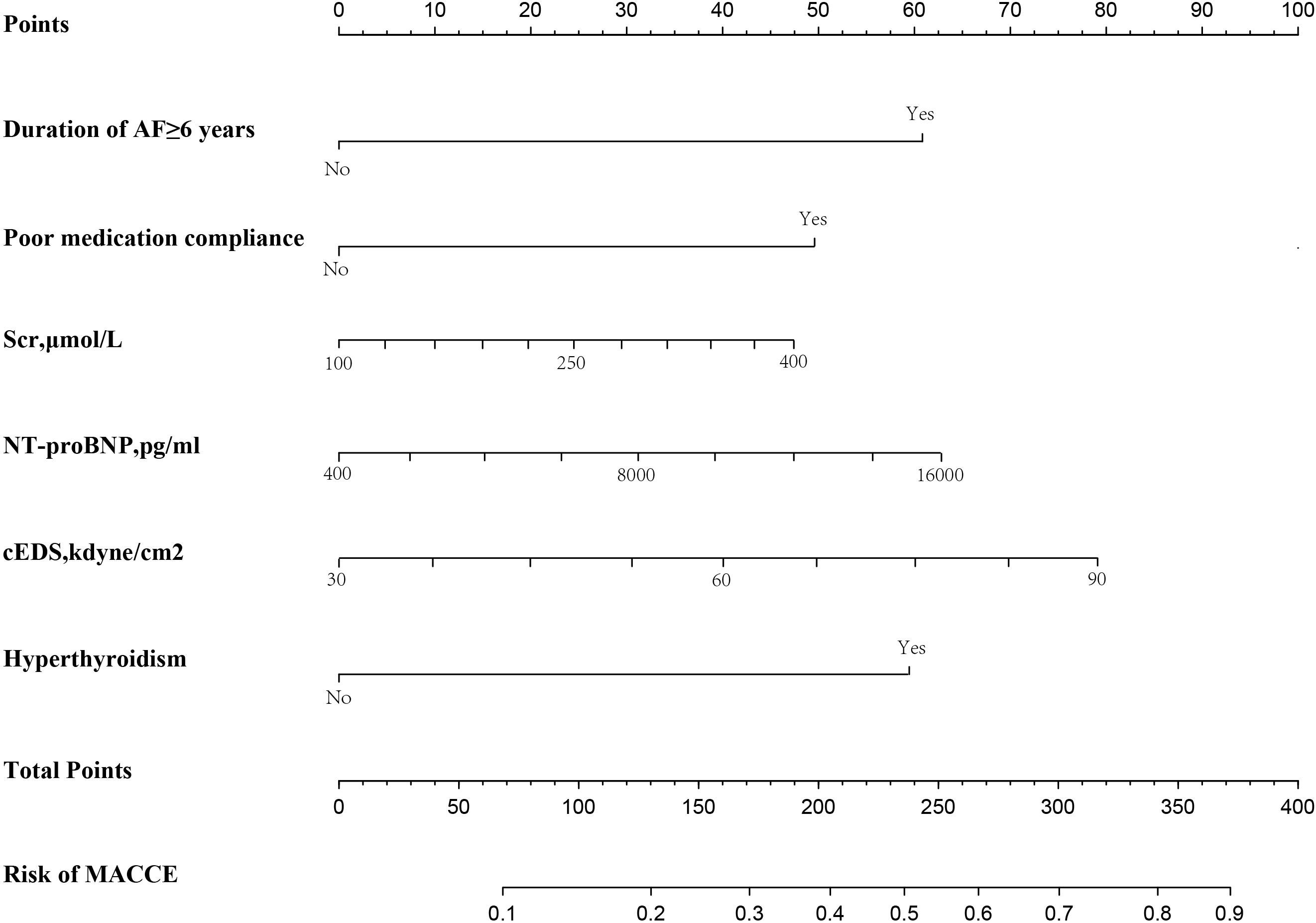
Nomogram to predict the risk of MACCE in patients with NVAF and HFpEF within one year of discharge. AF, atrial fibrillation; cEDS, circumferential end-diastolic stress; MACCE, major adverse cardiovascular and cerebrovascular events; NT-proBNP, N-terminal pro-brain natriuretic peptide; Scr, serum creatinine; NVAF, nonvalvular atrial fibrillation; HFpEF, heart failure with preserved ejection fraction.
Variables | B | SE | Wald | p | OR | 95% CI | |
Duration of AF |
1.085 | 0.342 | 10.065 | 0.002 | 2.959 | 2.541 | 3.382 |
Poor medication compliance | 0.936 | 0.032 | 7.252 | 0.010 | 2.554 | 1.732 | 5.276 |
Scr | 1.173 | 0.065 | 6.343 | 0.019 | 3.228 | 1.864 | 9.207 |
Hyperthyroidism | 1.337 | 0.303 | 19.471 | 0.000 | 3.808 | 3.460 | 4.213 |
NT-proBNP | 0.611 | 0.294 | 7.013 | 0.000 | 2.641 | 1.306 | 6.068 |
cEDS | 0.625 | 0.240 | 6.775 | 0.009 | 1.868 | 1.167 | 2.991 |
Constant | 0.147 | 0.395 | 4.399 | 0.026 | 1.479 |
MACCE, major adverse cardiovascular and cerebrovascular events; AF, atrial fibrillation; cEDS, circumferential end-diastolic stress; CI, confidence interval; NT-proBNP, N-terminal pro-brain natriuretic peptide; OR, odds ratio, Scr, serum creatinine; SE, standard error.
To verify the clinical validity of the nomogram, we adopted DCA in both the training and validation cohorts (Fig. 4A,B). The DCA quantified the net benefit at different threshold probabilities, indicating a wide range of high-risk threshold probabilities in both cohorts. Moreover, a calibration plot was generated to evaluate the performance of the nomogram in both cohorts (Fig. 5A,B). To further assess the goodness-of-fit, the Hosmer-Lemeshow test was conducted, yielding p-values of 0.573 for the training cohort and 0.628 for the validation cohort. These results indicate a high level of agreement between the observed and predicted probabilities of MACCE in both cohorts, providing additional evidence for the reliability of the nomogram.
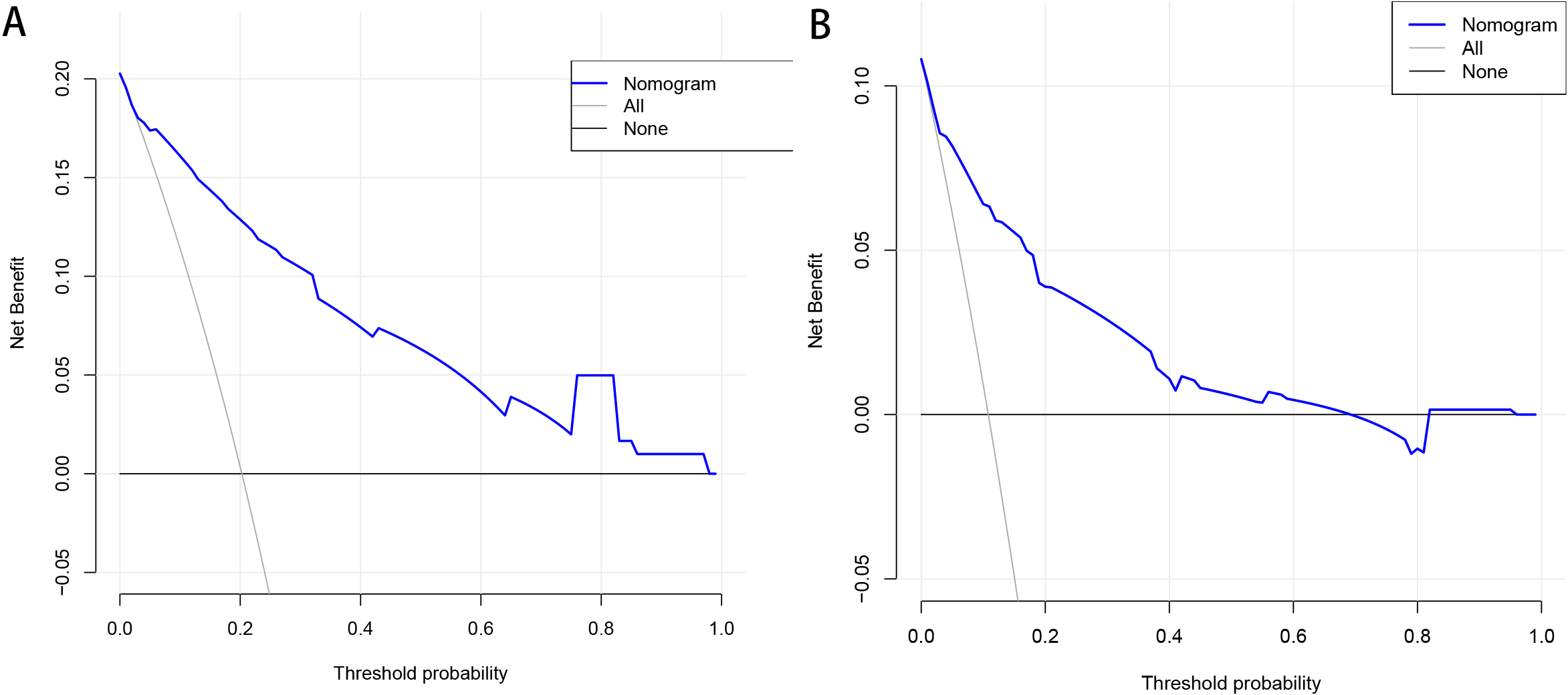
Decision curve analysis for the training cohort (A) and the validation cohort (B). The horizontal line indicates that all samples are negative and the net benefit is zero. The oblique line indicates that all samples are positive. The net benefit has a negative slope.
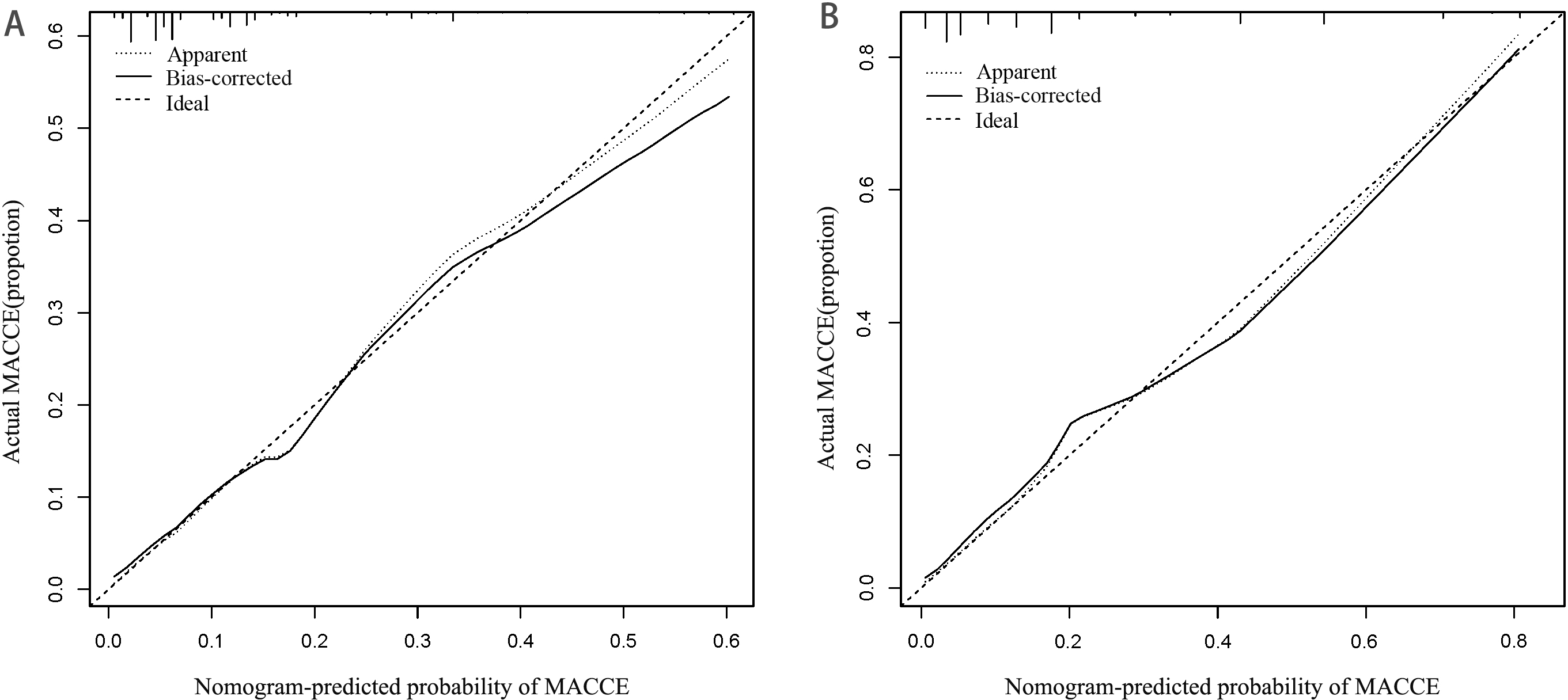
Calibration curve of a nomogram for the training cohort (A) and the validation cohort (B). The X-axis represents the overall predicted probability of MACCE within one year of discharge and the Y-axis represents the actual probability. Model calibration is indicated by the degree of fitting of the curve. MACCE, major adverse cardiovascular and cerebrovascular events.
AF is prevalent in HFpEF and is strongly associated with adverse clinical outcomes [2, 13]. Moreover, AF burden in HFpEF is linked to a decline in left atrial distensibility [14]. These pathological changes contribute to the development of HFpEF, which is characterized by progressively enhanced ventricular interactions, worsening of pulmonary vascular disease, and right HF. A meta-analysis [15], including 14 eligible studies, demonstrated that patients with HFpEF and AF experience a poor prognosis and heavy medical burden. Therefore, establishing a prediction model is crucial for improving clinical decision-making.
In our study, we identified several independent risk factors for MACCE in
patients with NVAF and HFpEF using multivariable logistic regression. These risk
factors include a duration of AF of
In contrast to using the receiver operator characteristic curve and the area under the curve to evaluate the model’s discriminative ability, we used DCA to validate the clinical utility of our model [19, 20]. The DCA results illustrate significant benefits in both the training and validation cohorts, indicating favorable clinical utility. Since our model is the first of its kind to predict the risk of MACCE in patients with NVAF and HFpEF, we couldn’t compare it to any existing ones. However, it is worth noting that DCA has a precedent of successful use, as it has been applied to validate Wu’s radiomics nomogram for preoperative prediction of lymph node metastasis in bladder cancer [21].
In patients with HF and normal left ventricular ejection fraction, AF significantly reduces cardiac output from baseline, leading to renal hypoperfusion and neuroendocrine changes resulting in chronic renal dysfunction. Our study revealed that elevated Scr and serum NT-proBNP levels were independent risk factors for the end-point event, suggesting an intrinsic interaction between them. Both basic [22] and clinical studies [23] establish a link between declining renal function and elevated serum NT-proBNP levels, which contribute to adverse clinical outcomes. The AKINESIS (Acute Kidney Injury N-gal Evaluation of Symptomatic heart faIlure Study) study confirmed that improved renal function is associated with mortality in acute HF, although it is not independent of hyperemia. Interestingly, achieving adequate hyperemia, as reflected by lower brain natriuretic peptide levels, was found to have a stronger associon with mortality in acute HF [24]. Hence, it is essential to remain vigilant for the possibility of renal hypoperfusion when diastolic dysfunction occurs in patients with AF.
Studies focusing on targeted metabolisms have revealed that HFpEF is associated
with more severe systemic microvascular endothelial dysfunction and inflammation
compared to HF with reduced ejection fraction [25]. This results in increased
fibrosis in HFpEF [25]. Consequently, myocardial fibrosis leads to decreased
ventricular distensibility and increased left ventricular cEDS. In our study, although
significantly elevated LVEDd (p
SGLT-2 inhibitors demonstrated a significant reduction in the risk of MACCE in
our study population (p
However, in this study, the impact of SGLT-2 inhibitors in patients with HFpEF and AF may be diminished when considering confounding factors, especially medication adherence. Additionally, the TOPCAT (Treatment of Preserved Cardiac Function Heart Failure with an Aldosterone Antagonist Trial) [30] analysis of three phenotypes in HFpEF revealed varying effects of spironolactone treatment. In the OPTIMIZE-HF (Organized Program to Initiate Lifesaving Treatment in Hospitalized Patients with Heart Failure) [31] study, digoxin treatment in elderly patients with HF (regardless of HF with reduced ejection fraction or HFpEF) reduced the risk of readmission without significantly affecting mortality. Hence, it is essential to recognize that no single drug can independently influence a patient’s endpoint events.
Results from the ARISTOTLE (Apixaban for Reduction in Stroke and Other Thromboembolic Events in Atrial Fibrillation) trial [32] indicate that thyroid disease, regardless
of classification, does not affect the clinical outcomes in patients with AF.
However, the combination of AF and hyperthyroidism led to significantly worse
outcomes. Our study focused on NVAF, where HF was a comorbidity, and our patients
had multiple underlying illnesses. In recent years, the CHA
Our nomogram offers a precise estimation of the risk of MACCE within one year of discharge, incorporating six easily obtainable early in the admission process. Our model has undergone external validation, confirming its effectiveness and accuracy. As a result, the findings of our study could enhance patient adherence to treatment and follow-up, enabling them to better understand the disease risk and make informed decisions.
Our study has some limitations. (1) This was a retrospective analysis study and although we included patients from two centers, the sample size was not big enough; (2) The high proportion of people lost to follow-up might have led to bias; (3) Considering that the coronavirus disease 2019 epidemic might have an impact on our study results, we need to revalidate the model after the disease is completely controlled; (4) To make it easier for clinicians to use our model, an app for assessment needs to be developed and continuously improved.
The novel nomogram prediction model developed in this study to predict the risk
of MACCE in patients with NVAF and HFpEF within 1 year after discharge based on
six independent predictors, namely duration of AF of
The datasets used and/or analyzed during the current study available from the corresponding author on reasonable request.
YW performed statistical analysis, graphical editing; YW, YHZ, HJ gathered the clinical information; and JDD designed the clinical trial and submitted the manuscript for publication. All authors wrote and approved the final manuscript. All authors have participated sufficiently in the work and agreed to be accountable for all aspects of the work.
All methods used in our study were in accordance with relevant guidelines and regulations. Since written informed consent was not required for this retrospective study according a national legislation and institutional requirements, written informed consent for participation was waived in this study with the approval of Clinical Research Ethics Committee of Taizhou People’s Hospital (No. TZRY-20180308) and Ethics Institutional Review Board of Zhongda Hospital (No. 2017ZDKYSB126).
Not applicable.
This work was supported by Taizhou People’s Hospital Research Start-up Fund Project. No. QDJJ202114.
The authors declare no conflict of interest.
Publisher’s Note: IMR Press stays neutral with regard to jurisdictional claims in published maps and institutional affiliations.