- Academic Editor
Background: Diabetes mellitus (DM) plays a key role in the
pathophysiology of metabolic syndrome (MetS). This study aimed to investigate the
association among DM, low-attenuation plaque (LAP) volume, and cardiovascular
outcomes across metabolic phenotypes in patients with suspected coronary artery
disease (CAD) who underwent coronary computed tomography angiography (CCTA).
Methods: We included 530 patients who underwent CCTA. MetS was defined
as the presence of a visceral adipose tissue area
The global prevalence of diabetes mellitus (DM) and metabolic syndrome (MetS)
has increased significantly over the past decades, contributing to an increased
risk of atherosclerotic cardiovascular disease (ASCVD) [1]. Clinical studies have
demonstrated that being overweight (25–29.9 kg/m
Coronary computed tomography angiography (CCTA) facilitates the diagnosis of coronary artery disease (CAD) and offers a prognostic value based on high-risk coronary plaque features beyond stenosis severity [8]. Furthermore, a recent CCTA study demonstrated that a low-attenuation non-calcified coronary plaque burden was the strongest prognostic marker among other clinical factors such as the presence of CAD and coronary artery calcium score (CACS) [9]. This finding suggests the utility of CCTA for identifying high-risk patients. In a subanalysis of a large clinical trial of patients with chest pain and distinct metabolic phenotypes who underwent CCTA, Kammerlander et al. [4] demonstrated that metabolically unhealthy individuals without obesity were at a high risk of ASCVD events. Although DM plays a key role in the pathophysiology of MetS [10], its association with high-risk plaque volume detected by CCTA and cardiovascular consequences remains unclear. This study aimed to investigate the associations among DM, high-risk plaque volume, and cardiovascular outcomes across metabolic phenotypes in patients with suspected CAD who underwent CCTA.
The Institutional Review Board approved the pooled data analysis (no. 2021-A). All participants provided written informed consent for the use of de-identified data including clinical information, laboratory test results, and CCTA imaging results. This retrospective observational study included patients who underwent CCTA between January 2018 and December 2020, were clinically identified as having stable chest symptoms and underwent abdominal computed tomography (CT) to assess abdominal obesity. The exclusion criteria were as follows: (1) diagnosis of acute coronary syndrome; (2) history of coronary artery bypass graft or open-heart surgery; (3) congestive heart failure; (4) history of percutaneous coronary intervention; (5) insufficient patient information; and (6) loss to follow-up. Fig. 1 shows a flowchart of the study population comprising 530 patients.
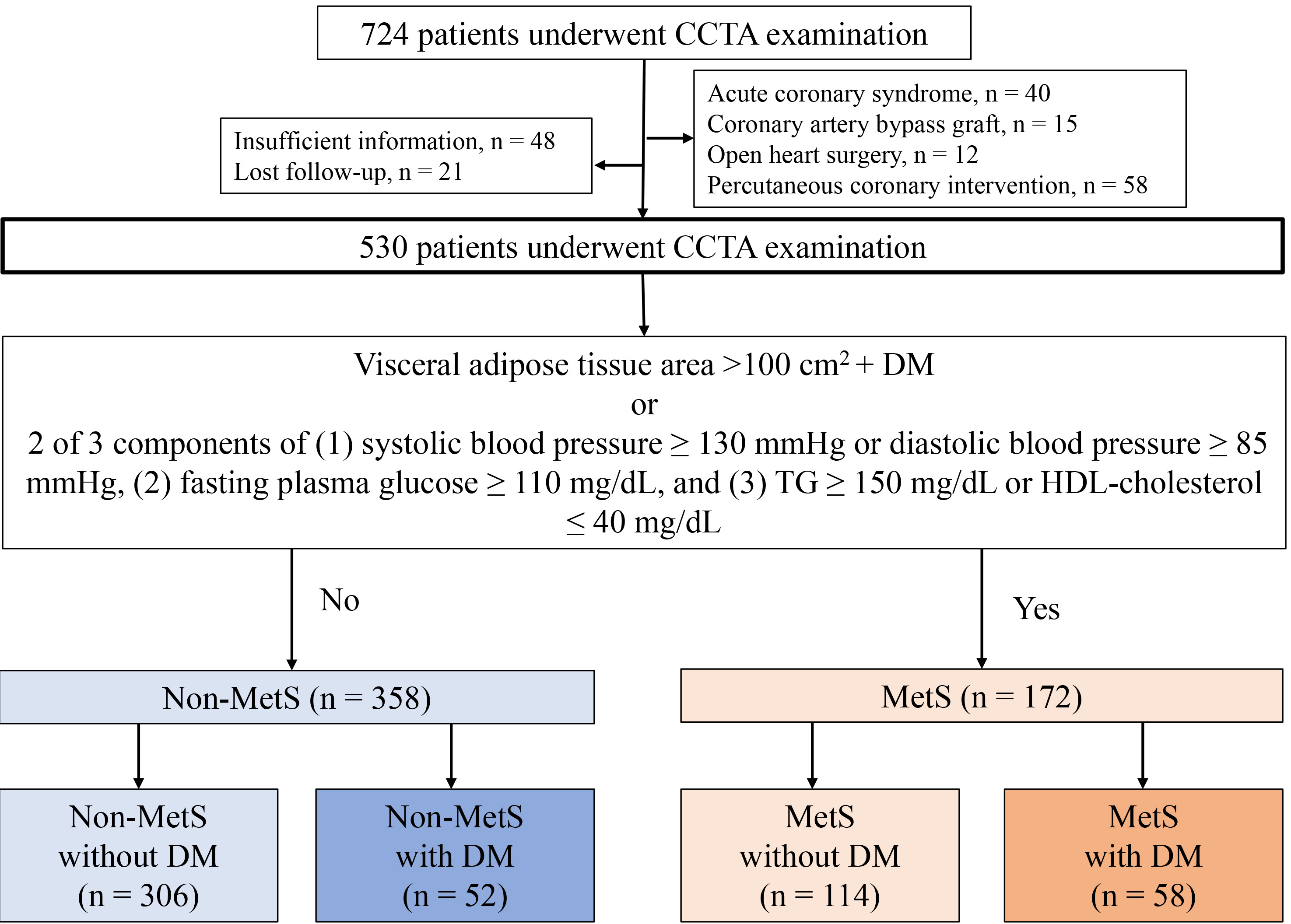
Flow chart of study patients. A flowchart illustrating the 530 patients who underwent CCTA examination and their categorisation into non-MetS and MetS groups. Patients were further categorised into four groups according to the presence or absence of metabolic equivalents of MetS and DM. CCTA, coronary computed tomography angiography; DM, diabetes mellitus; MetS, metabolic syndrome; TG, triglyceride; HDL, high-density lipoprotein.
Non-contrast-enhanced abdominal CT was performed before CCTA to quantify areas
with visceral adipose tissue (VAT). The VAT areas were measured at the L2–L3
level using SYNAPSE VINCENT (Fujifilm, Inc., Tokyo, Japan). Abdominal obesity was
defined as a VAT area
Pharmacological treatment and lifestyle modifications were recommended for all
patients, according to guidelines for hypertension, dyslipidemia, and diabetes
[14]. In addition, patients subsequently underwent invasive coronary angiography
or coronary revascularization based on the results of CCTA and noninvasive stress
tests [15]. According to the Japanese Atherothrombosis Society guidelines, the
treatment targets for low-density lipoprotein cholesterol for the primary
prevention of ASCVD are
CCTA was performed using a 320-row multidetector CT scanner (Aquilion ONE/NATURE
Edition; Canon Medical Systems Inc., Tokyo, Japan) [17]. A
The Agatston scores were categorised as 0, 1–100, 101–400, and
For coronary plaque analysis, coronary artery centerlines were identified
semi-automatically; the proximal and distal portions of the coronary plaque
lesions were manually defined; and the vessel wall, lumen, and plaque components
were auto-segmented and manually adjusted. Based on their composition, lesions
were categorised into calcified plaques (CPs) (
Epicardial adipose tissue (EAT) volume was measured from contrast-enhanced CT images using SYNAPSE VINCENT [17]. Several equidistant axial planes were extracted based on the heart size. The upper limit of the slice was set at the bifurcation of the pulmonary artery trunk, whereas the lower limit was set at the last slice that contained any heart structure. In each plane, the software auto-detected a smooth, closed pericardial contour as the region of interest; adipose tissue was identified with CT attenuation values ranging from –250 to –30 HU within the pericardial sac [17]. Finally, EAT volume was calculated as the sum of the EAT areas in each slice. The mean CT value within the measured EAT volume has been reported previously.
The primary endpoint was a major adverse cardiovascular event (MACE), a
composite of cardiovascular death, nonfatal myocardial infarction, unstable
angina, and symptom- or ischaemia-driven coronary revascularisation.
Cardiovascular death was defined as death resulting from cardiovascular causes,
including myocardial infarction, sudden cardiac arrest, heart failure, and stroke
[21]. Non-fatal myocardial infarction was defined as typical persistent chest
pain with elevated cardiac enzyme levels [21]. Unstable angina was defined as
new-onset angina, angina exacerbation with light exertion, or angina at rest
without elevated cardiac enzyme levels. Symptom- or ischaemia-driven coronary
revascularization was defined as coronary revascularization
All statistical analyses were performed using SPSS version 24 software (IBM
Corp., Armonk, NY, USA). Categorical variables were presented as absolute and
relative frequencies, and continuous variables were presented as mean
The mean age of the patients was 64
Non-MetS | MetS | p value | ||||
DM (–) (n = 306) | DM (+) (n = 52) | DM (–) (n = 114) | DM (+) (n = 58) | |||
Age | 64 (15) | 69 (10) | 62 (13) | 66 (12) | ||
Male, n (%) | 158 (52%) | 32 (62%) | 70 (61%) | 39 (67%) | 0.062 | |
BMI, kg/mm |
22.7 (3.4) | 22.1 (2.8) | 26.6 (4.2) | 27.7 (4.6) | ||
VAT area, cm |
78 (41) | 66 (32) | 148 (45) | 168 (62) | ||
Subcutaneous fat area, cm |
137 (70) | 105 (67) | 200 (98) | 192 (82) | ||
Systolic BP, mmHg | 136 (21) | 145 (22) | 148 (24) | 148 (25) | ||
Triglyceride, mg/dL | 134 (141) | 125 (98) | 221 (244) | 241 (488) | 0.001 | |
HDL-C, mg/dL | 67 (19) | 65 (17) | 56 (15) | 50 (13) | ||
LDL-C, mg/dL | 124 (33) | 110 (38) | 135 (36) | 115 (32) | ||
Haemoglobin A1c, % | 5.6 (0.3) | 7.1 (1.7) | 5.7 (0.3) | 7.2 (1.8) | ||
CRP (LogCRP), mg/L | 0.31 (0.86) | 0.20 (0.34) | 0.31 (0.71) | 0.35 (0.49) | ||
Hypertension | 168 (55%) | 44 (85%) | 107 (94%) | 54 (93%) | ||
Diabetes mellitus | 0 (0%) | 52 (100%) | 0 (%) | 58 (100%) | - | |
Dyslipidaemia | 155 (51%) | 36 (69%) | 113 (99%) | 49 (84%) | ||
Current or former tobacco user | 38 (12%) | 10 (19%) | 21 (18%) | 10 (17%) | 0.306 | |
CKD | 72 (24%) | 14 (27%) | 29 (25%) | 16 (28%) | 0.883 | |
Suita CVD risk score | 23.5 (10.8) | 35.5 (9.3) | 26.0 (9.0) | 33.9 (9.0) | ||
Atrial fibrillation | 27 (8.8%) | 10 (19%) | 9 (7.8%) | 9 (16%) | 0.056 | |
Medications | ||||||
ACE inhibitor or ARB | 47 (15%) | 15 (29%) | 34 (30%) | 21 (36%) | ||
Calcium channel blocker | 61 (20%) | 18 (35%) | 39 (34%) | 21 (36%) | 0.002 | |
11 (3.5%) | 5 (9.6%) | 10 (8.8%) | 4 (6.8%) | 0.102 | ||
Statins | 39 (13%) | 18 (35%) | 42 (37%) | 23 (40%) | ||
Insulin | 0 (0%) | 4 (7.7%) | 0 (0%) | 5 (8.6%) | - |
Values are given as means
ACE, angiotensin converting enzyme; ARB, angiotensin Ⅱ receptor blocker; BMI,
body mass index; BP, blood pressure; CKD, chronic kidney disease; CRP, C-reactive
protein; CVD, cardiovascular disease; VAT, visceral adipose tissue; HDL-C,
high-density lipoprotein cholesterol; LDL-C, low-density lipoprotein cholesterol;
DM (–), without diabetes mellitus; DM (+), with diabetes mellitus; MetS,
metabolic syndrome.
Table 2 shows the baseline CCTA findings of the four groups. The prevalence of a
CACS
Non-MetS | MetS | p value | ||||
DM (–) (n = 306) | DM (+) (n = 52) | DM (–) (n = 114) | DM (+) (n = 58) | |||
CACS | ||||||
CACS 0 | 153 (50%) | 13 (25%) | 49 (43%) | 14 (24%) | ||
CACS 1–100 | 77 (25%) | 13 (25%) | 36 (32%) | 14 (24%) | 0.569 | |
CACS 101–400 | 54 (18%) | 15 (29%) | 19 (17%) | 17 (29%) | 0.056 | |
CACS |
22 (7.2%) | 11 (21%) | 10 (8.8%) | 13 (22%) | ||
Stenosis severity on CCTA | ||||||
No CAD | 64 (21%) | 5 (9.6%) | 19 (17%) | 5 (8.6%) | 0.048 | |
Non-obstructive CAD | 131 (43%) | 17 (33%) | 51 (45%) | 26 (45%) | 0.490 | |
Obstructive CAD | 111 (36%) | 30 (58%) | 44 (39%) | 27 (46%) | 0.021 | |
Coronary plaque burden and composition | ||||||
NCP volume, % | 19.7 (5.7) | 20.6 (6.6) | 21.2 (6.9) | 23.4 (8.4) | ||
LAP volume, % | 2.4 (1.7) | 3.2 (2.6) | 3.3 (2.9) | 4.4 (5.0) | 0.001 | |
CP volume, % | 0.7 (2.4) | 2.1 (3.9) | 0.9 (2.9) | 1.6 (3.7) | 0.002 | |
LAP volume |
50 (16%) | 12 (23%) | 29 (25%) | 20 (34%) | 0.008 | |
Napkin-ring sign, n (%) | 54 (18%) | 14 (27%) | 27 (24%) | 12 (21%) | 0.313 | |
Spotty calcification, n (%) | 99 (32%) | 14 (27%) | 35 (31%) | 18 (31%) | 0.887 | |
EAT volume, mL | 105 (42) | 107 (40) | 148 (46) | 176 (46) | ||
EAT mean CT value, HU | –79.0 (5.3) | –77.7 (5.3) | –80.7 (4.0) | –79.5 (5.0) | 0.001 |
Values are given as means
CACS, coronary artery calcium score; CAD, coronary artery disease; CP, calcified
plaque; DM (–), without diabetes mellitus; DM (+), with diabetes mellitus; EAT,
epicardial adipose tissue; MetS, metabolic syndrome; NCP, non-calcified plaque;
LAP, low-attenuation plaque; CCTA, coronary computed tomography angiography; CT,
computed tomography; HU, Hounsfield units.
During a mean follow-up period of 2.7
Non-adjusted HR | 95% CI | p value | |
All patients (n = 530) | |||
Age | 1.03 | 1.002–1.07 | 0.040 |
Male | 1.65 | 0.72–3.88 | 0.229 |
CACS |
5.09 | 2.25–11.54 | |
LAP volume |
6.03 | 2.70–13.40 | |
Napkin-ring sign | 5.43 | 2.46–11.97 | |
Spotty calcification | 1.24 | 0.55–2.81 | 0.602 |
Obstructive CAD | 8.45 | 2.90–24.60 | |
EAT volume | 1.001 | 0.99–1.01 | 0.853 |
EAT mean CT value | 1.03 | 0.99–1.08 | 0.126 |
BMI |
0.45 | 0.16–1.20 | 0.111 |
VAT |
1.28 | 0.58–2.80 | 0.535 |
MetS | 1.39 | 0.62–3.10 | 0.415 |
DM | 3.74 | 1.70–8.20 | 0.001 |
Chronic kidney disease | 1.64 | 0.80–3.39 | 0.175 |
CRP | 1.25 | 0.70–2.23 | 0.449 |
CRP was log-transformed for analysis.
BMI, body mass index; CACS, coronary artery calcium score; CAD, coronary artery
disease; CI, confidence interval; CRP, C-reactive protein; EAT, epicardial
adipose tissue; HR, hazard ratio; VAT, visceral adipose tissue; DM, diabetes
mellitus; MetS, metabolic syndrome; CT, computed tomography; LAP, low-attenuation
plaque.
Predictor | HR | 95% CI | p value | |
Model 1 | DM (Reference, non-DM) | 2.68 | 1.16–6.18 | 0.02 |
Low-attenuation plaque volume |
5.41 | 2.42–12.11 | ||
Model 2 | MetS (Reference, non-MetS) | 0.99 | 0.44–2.23 | 0.99 |
Low-attenuation plaque volume |
5.83 | 2.59–13.10 | ||
Model 3 | DM without MetS (Reference, non-DM without MetS) | 6.89 | 2.33–20.39 | 0.001 |
Low-attenuation plaque volume |
9.66 | 3.29–28.35 | ||
Model 4 | DM with MetS (Reference, non-DM without MetS) | 1.69 | 0.56–5.09 | 0.34 |
Low-attenuation plaque volume |
9.24 | 2.82–30.27 | ||
Model 5 | Non-DM without MetS (Reference, non-DM without MetS) | 2.24 | 0.58–8.53 | 0.23 |
Low-attenuation plaque volume |
4.62 | 1.37–15.51 | 0.013 |
Models 1–5 were adjusted for the Suita CVD risk score. CI, confidence interval; DM, diabetes mellitus; HR, hazard ratio; MetS, metabolic syndrome; CVD, cardiovascular disease.
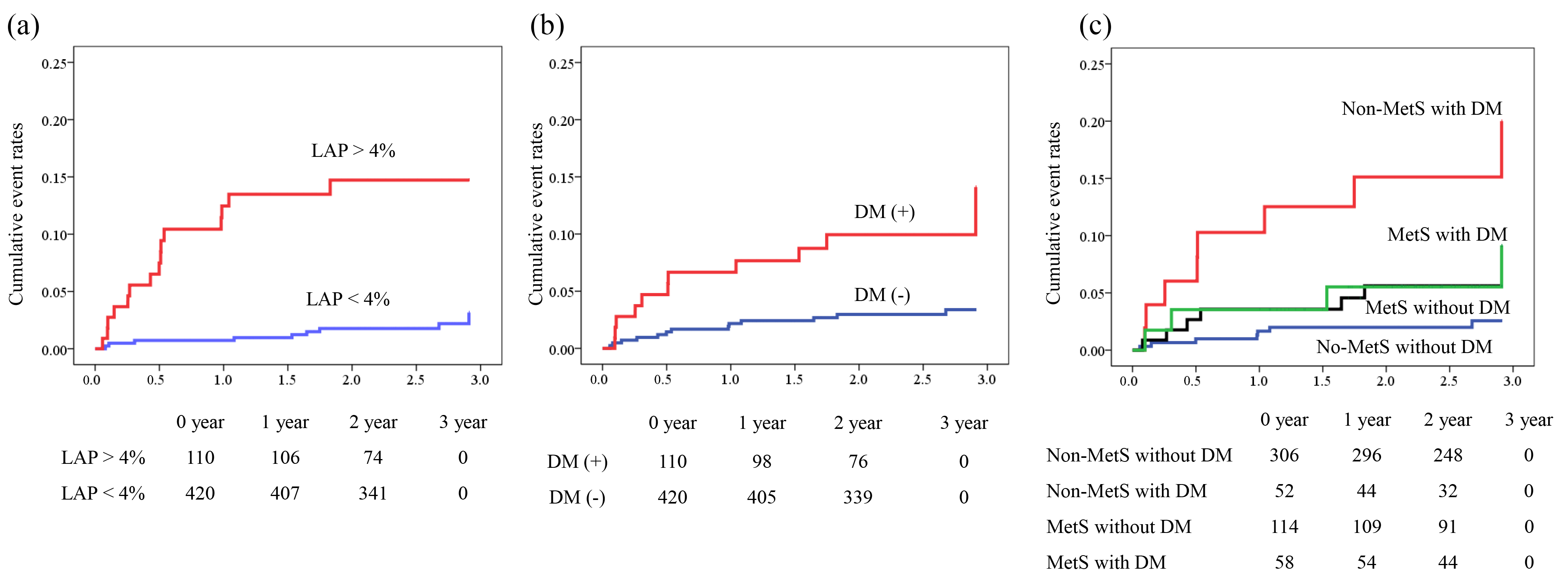
Kaplan–Meier analysis for prediction of MACEs. Kaplan–Meier
curves demonstrating significant differences in cumulative event rates between
metabolic phenotypes using a composite endpoint of cardiovascular death, acute
coronary syndrome, and symptom- or ischaemia-driven coronary revascularization.
(a) Patients with and without LAP volume
In the subgroup analysis of each metabolic phenotype (non-DM without MetS as a
reference), DM without MetS functioned as a predictor of MACE independent of LAP
volume
This study investigated the association between distinct metabolic phenotypes,
defined by the presence or absence of MetS and DM, and cardiovascular outcomes in
symptomatic patients who underwent CCTA. The key findings of this study were as
follows: (1) among the four groups, the most unfavourable prognosis was observed
in patients without MetS but with DM, compared to those with MetS with or without
DM; (2) a LAP volume
Several large clinical trials have demonstrated that myocardial ischaemia is an important surrogate marker for improving outcomes in patients with stable CAD, whereas ischaemia-guided management has a limited ability to prevent acute coronary events compared to optimised medical therapy [22, 23, 24]. These findings raise questions about the credibility of ischaemia-guided management of patients with stable CAD, redirecting attention toward coronary microvascular dysfunction and high-risk plaque burden [24]. An increased plaque burden, especially of noncalcified plaques, has been reported in patients with MetS [25]. Yonetsu et al. [25] used optical coherence tomography to demonstrate that MetS is associated with an increased burden of lipid-rich plaques. Although these findings indicate a potential link between obesity, metabolic disorders, and unfavourable coronary plaque features, there is limited knowledge regarding coronary plaque burden in distinct metabolic phenotypes with and without DM.
Previous clinical studies have reported an inverse association between BMI and cardiovascular prognosis (obesity paradox) [3, 4, 5]. In patients with ASCVD and DM, Pagidipati et al. [5] demonstrated that overweight or obese individuals had a lower cardiovascular risk than those with normal weight. In a subanalysis of a large clinical trial of patients with chest pain who underwent CCTA, Kammerlander et al. [4] demonstrated that of all patients with distinct metabolic phenotypes, metabolically unhealthy individuals without obesity exhibited a significantly high risk of plaque burden and ASCVD events. This paradoxically benign effect of obesity may be explained by its protective effect against atherosclerosis. Although obesity can cause inflammation in perivascular adipose tissues and exacerbate atherosclerotic lesion formation, adipose tissue plays a role in atheroprotection under healthy conditions [26]. Clinically, obesity may be associated with metabolic reserves in older patients by protecting against malnutrition, frailty, and osteoporosis [3]. Patients with obesity lack sarcopenia and have limited exercise capacity and reduced mobility, which are associated with increased cognitive decline, heart failure, and mortality. This might explain our observation that patients with MetS had better cardiovascular outcomes, albeit with a high prevalence of LAP (4%).
In addition, increased fasting plasma glucose levels were observed in patients
without non-MetS DM. Hyperglycaemia and insulin resistance have been reported to
be key drivers of calcification in DM [10, 27]. Liu et al. [28] showed
that higher glucose levels and their variability are associated with plaque
rupture in patients with ST-segment elevation myocardial infarction. In line with
these observations, we found that a LAP
CAD is a chronic inflammatory disease associated with the underlying risk of metabolic disorders [29]. A close relationship has been reported between abdominal visceral obesity and increased coronary atherosclerotic burden [7, 30]. Our results demonstrated that MetS patients with or without DM had increased EAT and LAP volumes, whereas non-MetS patients with DM had the worst outcomes with lower EAT and LAP volumes, indicating an alternating pathophysiology of acute coronary syndrome. Our findings are consistent with those of Kammerlander et al. [4], who demonstrated that both metabolically unhealthy obese and non-obese patients exhibit increased high-risk plaques. The increased prevalence of obstructive CAD and vascular CP in DM patients may explain this finding [31]. Distinct plaque characteristics may reflect the different stages (advanced or less advanced) of coronary atherosclerosis, resulting in different responses to lipid-lowering therapy [32]. Furthermore, previous studies investigating plaque structural stress have demonstrated that microcalcifications contribute to increased stress, leading to plaque rupture and myocardial infarction [33]. These observations provide insight into the poorer outcomes observed in DM patients without MetS in this study.
Although we observed that the EAT volume was not correlated with cardiovascular outcomes, the mean CT value of the EAT was (Table 3). EAT has been associated with coronary atherosclerosis, calcification, and cardiovascular outcomes, and has attracted attention as a therapeutic target [34, 35, 36]. This association has motivated the development of imaging methods that enable the assessment of inflammation in pericoronary adipose tissue, which interacts with the underlying vascular wall by producing proinflammatory adipokines [37]. In a retrospective CCTA study, Oikonomou et al. [38] demonstrated that an increased fat attenuation index (FAI) around the epicardial coronary arteries predicted cardiovascular outcomes. Moreover, a recent meta-analysis demonstrated that higher pericoronary FAI values offer additional prognostic value for MACE in 6335 patients analyzed in prospective follow-up clinical studies [39]. A higher CT attenuation of the EAT indicates an increased inflammatory status, which supports our finding that non-MetS patients with DM had the worst prognosis. Noninvasive assessment of coronary plaque burden and metabolic phenotypes allows for further risk stratification of symptomatic patients undergoing CCTA.
This study included a relatively small number of patients, and event rates
during follow-up were relatively low (
Individuals with DM (without MetS) had a significantly higher risk of developing MACEs than those with MetS. This observation indicates that DM is an independent predictor of ASCVD events, regardless of the presence of obstructive CAD or high-risk plaque volume.
This study investigated the association among DM, high-risk coronary plaque burden, and MACEs across metabolic phenotypes stratified by the presence or absence of MetS and DM in patients with suspected CAD who underwent CCTA.
Among the four metabolic phenotypes, the incidence of MACEs was the highest in
the non-MetS with DM group, followed hierarchically by the MetS with DM, MetS
without DM, and non-MetS without DM groups. A LAP volume of
ACS, acute coronary syndrome; ASCVD, atherosclerotic cardiovascular disease; CACS, coronary artery calcium score; CAD, coronary artery disease; CCTA, coronary computed tomography angiography; CP, calcified plaques; DM, diabetes mellitus; LAP, low-attenuation plaque; MACE, major adverse cardiovascular events; MetS, metabolic syndrome; NCP, noncalcified plaque.
The data that support the findings of this study are available from the corresponding author on reasonable response.
KO, KS, and DF contributed to the study conception and design. KO, KH, HY, HI, YK, NK, and DF contributed to data acquisition, analysis, and interpretation. KO, KS, KH, HY, HI, YK, and NK drafted the manuscript. DF critically revised the manuscript. All authors read and approved the final manuscript, and agreed to be accountable for all aspects of the manuscript to ensure its integrity and accuracy.
The Fujiikai Kashibaseiki Hospital Institutional Review Board approved the pooled data analysis (No. 2021-A). All participants provided written informed consent for using de-identified data, including their clinical information, laboratory test results, and CCTA imaging results.
Not applicable.
This work was partially supported by the JSPS Kakenhi Grants (Number 22K08109 to KO).
The authors declare no conflict of interest.
Publisher’s Note: IMR Press stays neutral with regard to jurisdictional claims in published maps and institutional affiliations.