- Academic Editor
†These authors contributed equally.
Coronary artery disease is a leading cause of death worldwide. Major adverse cardiac events are associated not only with coronary luminal stenosis but also with atherosclerotic plaque components. Coronary computed tomography angiography (CCTA) enables non-invasive evaluation of atherosclerotic plaque along the entire coronary tree. However, precise and efficient assessment of plaque features on CCTA is still a challenge for physicians in daily practice. Artificial intelligence (AI) refers to algorithms that can simulate intelligent human behavior to improve clinical work efficiency. Recently, cardiovascular imaging has seen remarkable advancements with the use of AI. AI-assisted CCTA has the potential to facilitate the clinical workflow, offer objective and repeatable quantitative results, accelerate the interpretation of reports, and guide subsequent treatment. Several AI algorithms have been developed to provide a comprehensive assessment of atherosclerotic plaques. This review serves to highlight the cutting-edge applications of AI-assisted CCTA in atherosclerosis plaque characterization, including detecting obstructive plaques, assessing plaque volumes and vulnerability, monitoring plaque progression, and providing risk assessment. Finally, this paper discusses the current problems and future directions for implementing AI in real-world clinical settings.
Despite advances in primary and secondary preventive therapy, coronary artery disease (CAD) remains the predominant cause of morbidity and mortality globally [1]. The luminal stenosis of the coronary artery had been thought to be the main variable for risk stratification and the choice of treatment. However, that concept has been challenged during the past decades by the theory that atherosclerotic plaque is more associated with major adverse cardiac events (MACE) rather than obstructive or nonobstructive coronary disease [2, 3]. Previous studies have demonstrated that plaque morphology and composition play a major role in plaque stability and subsequent risk of acute coronary syndrome (ACS) [4, 5].
This renewed interest in atherosclerotic plaque stabilization has resulted in the development of several imaging techniques, including invasive modalities such as intravascular ultrasound (IVUS), optical coherence tomography (OCT), and non-invasive modalities such as computed tomography (CT), magnetic resonance imaging (MRI) and positron emission tomography (PET) [6, 7]. In the recent guidelines, coronary CT angiography (CCTA) has become the first-line diagnostic technique for the management of patients with stable chest pain [8, 9]. CCTA enables the evaluation of the presence, luminal stenosis, composition, and vulnerability of plaques throughout the coronary tree [10, 11]. However, the increasing use of CCTA exams in daily clinical practice has created an enormous challenge for radiologists and clinicians to assess this valuable information on atherosclerotic plaques accurately and efficiently.
Artificial intelligence (AI), as well as related techniques such as radiomics, might be ideally suited to solve these challenges [12, 13, 14]. AI has increasingly been used in CT to assess atherosclerotic plaques and can potentially improve the radiologists’ workflow, reduce image post-processing time, and improve the accuracy of test results [15, 16, 17]. In addition, risk stratification and prognosis can be more accurately evaluated as AI enables the processing of large amounts of data [13]. Several reviews have been published to discuss the AI methods used for CCTA interpretation [17, 18, 19] or plaque characterization [20, 21]. This review focuses on the latest AI applications for characterizing atherosclerotic plaques on CCTA, ranging from detecting luminal stenosis to risk prediction. We performed a search of PubMed, EMBASE, Scopus and Google Scholar for articles published between database inception and June 31, 2023, using the search terms (“coronary CT angiography” OR “coronary computed tomography angiography” OR “cardiac CT”) AND (“artificial intelligence” OR “machine learning” OR “deep learning” OR “computer-aided diagnostic tools” OR “radiomics”) AND (“plaque” OR “atherosclerosis” OR “coronary artery disease” OR “heart” OR “cardiac” OR “cardio” OR “infarct”), with no language restrictions. Recent applications of AI-assisted CT plaque analysis are presented in Table 1 (Ref. [22, 23, 24, 25, 26, 27, 28, 29, 30, 31, 32, 33, 34, 35]). We also highlight existing problems and future directions before the widespread implementation of AI can be adopted in daily clinical practice.
Study | Input data | Methods | Remarks |
Detection of plaque stenosis | |||
Hong et al. [22] | 156 CCTA scans | CNN | The DL method had a strong correlation with experts for stenosis measurements. |
Choi et al. [23] | 232 CCTA scans | CNN | AI-based software (Cleerly CORONARY, Cleerly Healthcare, New York, USA) showed high diagnostic performance compared with expert readers. |
Griffin et al. [24] | 303 CCTA scans | CNN | AI-based software (Cleerly CORONARY, Cleerly Healthcare, New York, USA) showed high diagnostic performance compared with quantitative coronary angiography. |
Liu et al. [25] | 165 CCTA scans | CNN | AI-based software (CoronaryDoc, ShuKun Techonolgy, Beijing, China) improved radiologists’ diagnostic performance, especially for inexperienced readers. |
Quantification of plaque | |||
Zeleznik et al. [26] | 1636 CAC scans | CNN | The DL algorithm showed good agreement with expert manual scoring in both ECG-gated and non-gated CT. |
Velzen et al. [27] | 7240 cardiac CT | CNN | The DL algorithm enabled the quantification of coronary and thoracic calcium with good agreement with manual scoring in varied CT protocols and populations. |
and chest CT | |||
Lin et al. [28] | 921 CCTA scans | ConvLSTM network | The DL system had excellent agreement compared with expert readers and IVUS for plaque volume measurement. |
Jávorszky et al. [29] | 894 CCTA scans | Attention U-net | The DL algorithm had an excellent agreement with expert plaque segmentation. |
Characterization of vulnerable plaque | |||
Kolossváry et al. [30] | 25 CCTA scans | Radiomics | Radiomics had superior performance compared to the best conventional CT metrics for the detection of vulnerable plaque. |
Al’Aref et al. [31] | 46 CCTA plaque features in 124 patients | XGBoost | The ML algorithm outperformed a model containing diameter stenosis, lesion length, plaque volume, plaque burden, and HRP to predict culprit lesions. |
Lin et al. [32] | 120 CCTA scans | Radiomics | Radiomics offered incremental value for identifying culprit lesions when added to a model including HRP and plaque volumes. |
Clustering | |||
Chen et al. [33] | 299 CCTA scans | Radiomics | Radiomics showed moderate to good diagnostic performance in identifying vulnerable plaques associated with an increased risk of MACE. |
XGBoost | |||
Prediction of future adverse CAD events | |||
Motwani et al. [34] | 25 clinical and 44 CCTA features in 10,030 patients | LogitBoost | The ML model showed better risk prediction value compared with the Framingham risk score, segment stenosis score, or segment involvement score for 5-year all-cause mortality. |
Nakanishi et al. [35] | 46 clinical and 31 CT variables in 66,636 patients | LogitBoost | The ML model outperformed ASCVD risk, segment stenosis score, or segment involvement score in the prediction of both CVD and CHD death. |
Notes: AI, artificial intelligence; CCTA, coronary CT angiography; CNN, convolutional neural network; DL, deep learning; CAC, coronary artery calcification; HRP, high-risk plaque; MACE, major adverse cardiac events; ECG, electrocardiogram; IVUS, intravascular ultrasound; ConvLSTM, convolutional long short-term memory; ML, machine learning; ASCVD, atherosclerotic cardiovascular disease; CVD, cardiovascular disease; CHD, congenital heart disease; XGBoost, extreme gradient boosting; CAD, coronary artery disease; CT, computed tomography.
AI is a computer science discipline that aims to perform tasks by simulating human intelligence tasks. It contains several subfields, such as machine learning (ML) and deep learning (DL). Fig. 1 summarizes the basic terminology of AI and its relevant techniques.
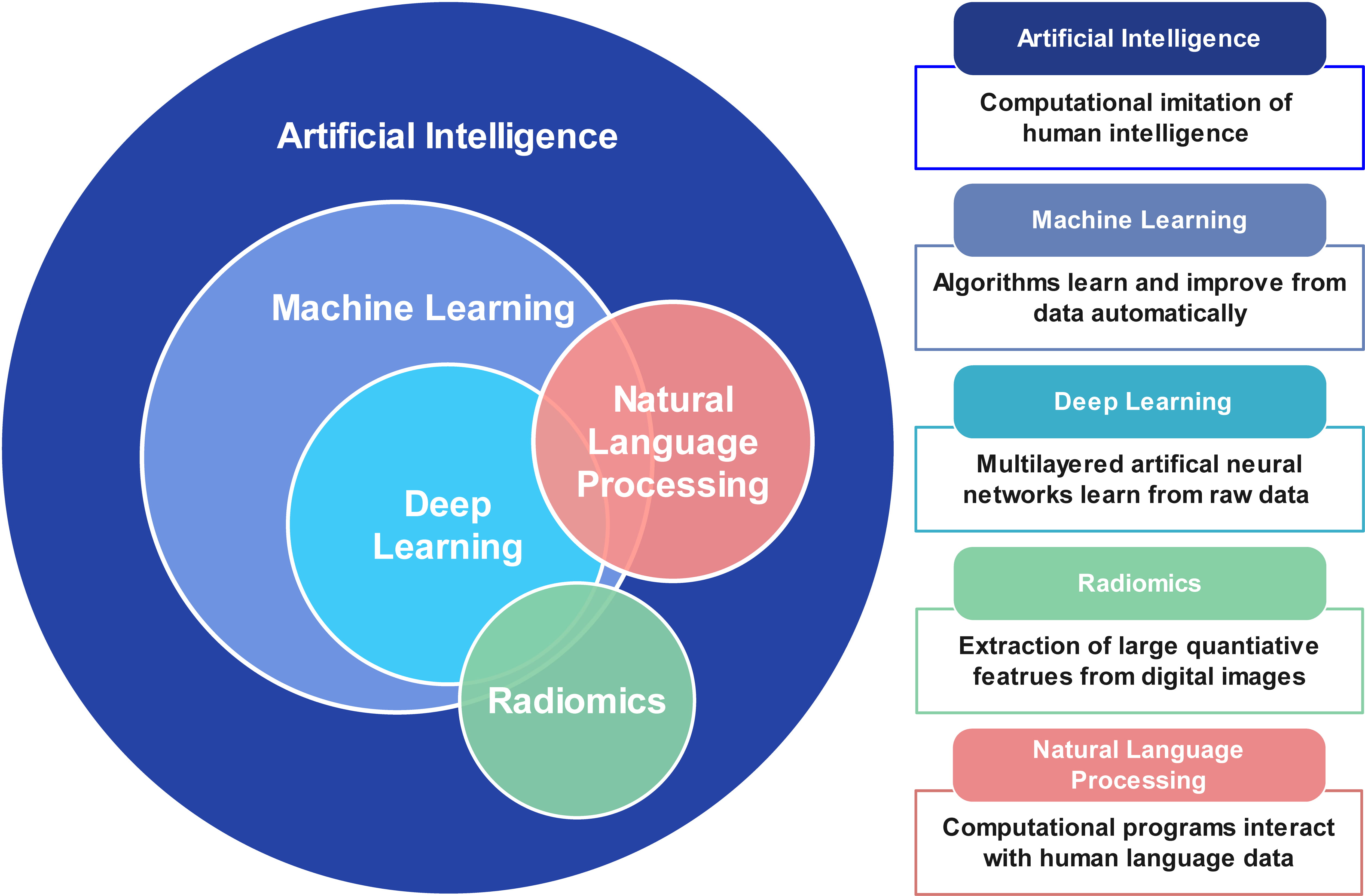
Basic terminology of artificial intelligence, machine learning, deep learning, and natural language processing.
ML is a branch of AI that involves a growing number of algorithms. ML can be classified into supervised learning, unsupervised learning, and reinforcement learning [36]. Supervised learning, including regression analysis, supports vector machines, and random forest, which refers to learning from labeled data to predict a known outcome. In contrast, unsupervised and reinforcement learning perform unlabeled learning to predict unknown associations. Principal component analysis and clustering are examples of unsupervised learning.
DL is a further subbranch of ML, which uses multilayers of artificial neural networks to automatically learn from raw data [37]. Convolutional neural networks (CNNs) are the most commonly used DL algorithms in medical image analysis. CNNs are composed of 3 major layers: convolution layer, pooling layer, and fully-connected layer. CNNs have been widely used for object detection, classification, and segmentation from images.
Radiomics is the process of extracting high-dimensional quantitative radiological imaging features indiscernible to the human reader [38]. These features characterize the complex spatial relationship between voxels within the region of interest. ML methods can then be combined with radiomics to identify valuable imaging markers to make predictions.
Natural language processing (NLP) methods are advanced AI technologies designed to understand and process human language [39]. In the medical field, NLP can help extract meaningful information from large amounts of data during health care processes, which may reduce clinicians’ workloads and support treatment decisions. Another emerging application of NLP is image analysis [40]. Transformer network is most commonly used in NLP for image processing, such as image detection, segmentation, and reconstruction. NLP can be performed with ML or DL to analyze complex multimodal information.
Current CCTA reporting is based on the visual estimation of stenosis severity
[41]. Accurate assessment of the degree of stenosis is critical to guide
treatment decisions by CCTA, including whether to perform invasive coronary
angiography. However, there is substantial inter-observer variability among
independent readers in real-world practice. A subanalysis of the Prospective
Multicenter Imaging Study for Evaluation of Chest Pain (PROMISE) trial
demonstrated that expert interpretation at the core laboratory reclassified 41%
fewer patients with obstructive plaques (defined as stenosis
AI-powered techniques have shown promising advances in detecting obstructive
CAD. Several AI methods have been developed for automatic coronary artery
segmentation and stenosis detection. Kang et al. [43] proposed an ML
algorithm to automatically detect
There is increasing evidence demonstrating that the measurement of plaque volume
and composition increases the prognostic value of patients who are at higher risk
for future CAD events [45, 46, 47]. In a subanalysis of the Scottish Computed
Tomography of the HEART (SCOT-HEART) trial, the investigators found that patients
with low attenuation plaque (LAP) burden
AI algorithms that aim to enhance the automation of quantifying plaques have,
therefore, been developed [24, 28, 29]. Liu et al. [53] trained a
vessel-focused 3D CNN to automatically segment coronary plaques with 25 patients.
They found the proposed algorithm achieved dice scores of 0.73, 0.68, and 0.83
for noncalcified plaques, mixed calcified plaques, and calcified plaques,
respectively. In a similar study, Jávorszky et al. [29] used a 3D
U-net model to segment plaques with 308 patients’ 894 CCTA scans. The results
showed the DL model had good agreement with expert analysis to quantify total
(intra-class correlation [ICC] = 0.88), noncalcified (ICC = 0.84), and calcified
(ICC = 0.99) plaque volumes. Recently, Lin et al. [28] developed and
validated a novel DL algorithm to automatically segment coronary plaques in a
multicenter cohort of 921 patients. The DL system showed excellent agreement with
IVUS (ICC = 0.95) for total plaque volume measurements at a much shorter analysis
time than for expert manual analysis (5.65
Newer quantitative AI-based algorithms are under development, which aims to
eliminate manual and labor-intensive processes. Recently, an AI-assisted CCTA
plaque software (Cleerly CORONARY, Cleerly Healthcare, New York, USA) has
received FDA approval, which uses a series of CNNs to automatically finish
coronary artery segmentation, lumen and arterial wall determination, stenosis,
and plaque quantification [24]. Initial validation studies have shown that the
software had high diagnostic performance in detecting
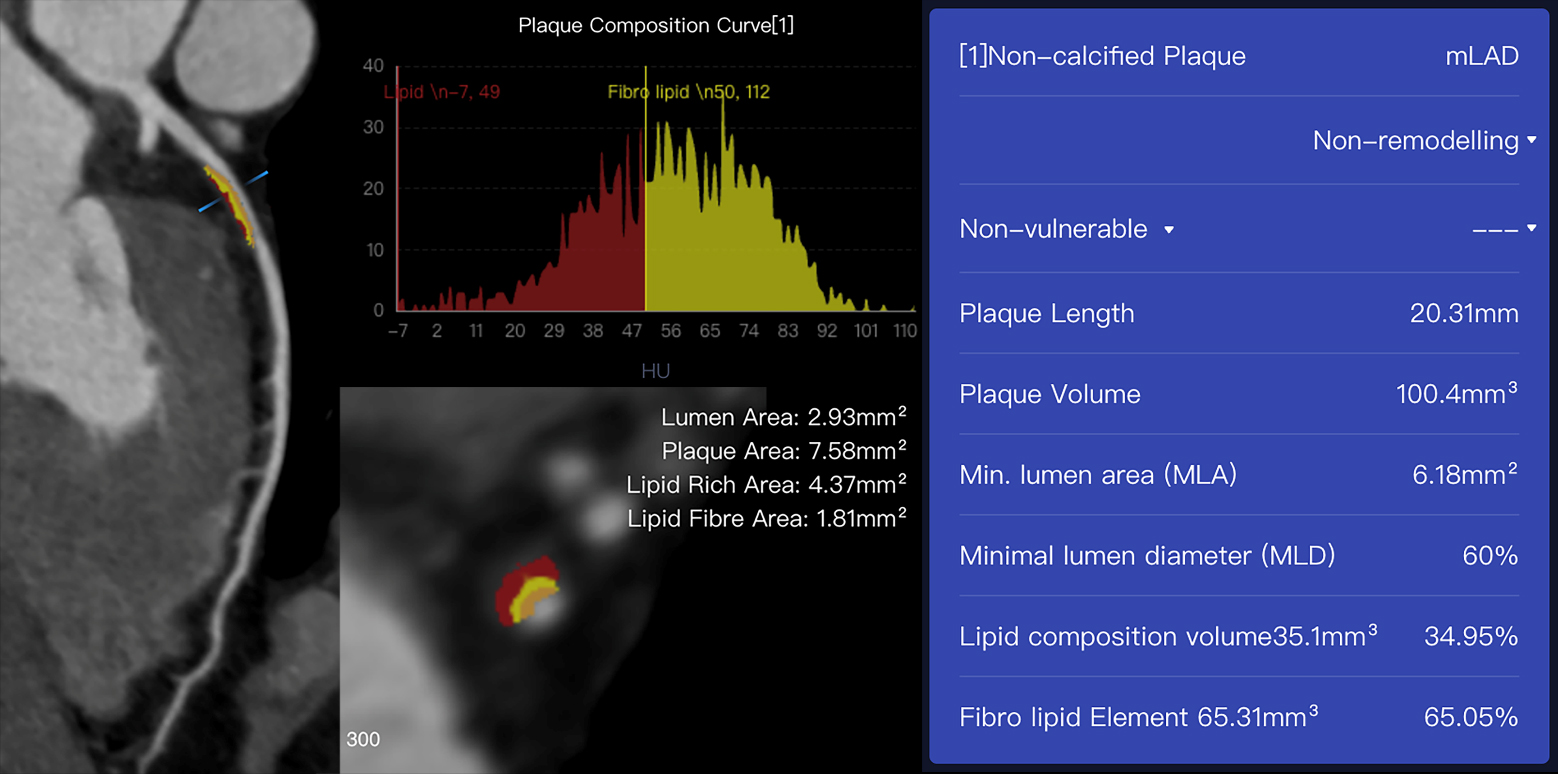
An example case of plaques assessed using AI-assisted CCTA
software (CoronaryDoc, ShuKun Techonolgy, Beijing, China). A 41-year-old male
with a history of exertional chest pain had a non-calcified plaque with 100.4
mm
Vulnerable plaque rupture is a leading cause of ACS [54]. Histologic and intravascular imaging findings have demonstrated that plaque features associated with culprit lesions at ACS are a large lipid-rich necrotic core, a thin fibrous cap, micro-calcification, positive remodeling, chronic inflammation, and neovascularization [55]. The early identification of vulnerable plaques is important for individualized risk assessment and clinical treatment [6, 56, 57].
CCTA can not only identify both calcified and noncalcified plaques but also characterize specific high-risk plaque features. The major high-risk plaque features include positive remodeling, LAP, the napkin ring sign, and spotty calcification [41]. Multiple studies have shown that the presence of high-risk plaque (HRP) on CCTA was associated with subsequent major adverse cardiovascular events [58, 59, 60]. However, these HRP features are generally assessed visually and only have a modest interobserver agreement, even among expert readers (Kappa = 0.15–0.69) [59, 61]. This highlights the need for improved automated methods for assessing HRP and standardized reporting.
However, studies on the applications of AI for identifying vulnerable plaques are scarce. In a sub-study of the CLARIFY trial, an AI-based software was evaluated to detect HRP features (including positive remodeling and LAP) in 232 patients [62]. Unfortunately, the results showed that AI had poor agreement with 3 expert readers, with a weighted Kappa coefficient of 0.22, 0.17, and 0.26, respectively. To date, automatically quantifying HRP features using AI remains a great challenge.
Radiomics techniques might be feasible to solve these challenges. Several
studies have demonstrated that CCTA-based radiomics methods may help detect
vulnerable plaques both in vivo and ex vivo [30, 32, 63].
Kolossváry et al. [63] trained a radiomics-based ML model to
diagnose histologically verified atherosclerotic lesions on 95 coronary plaques
in 7 male donors. The results found that the radiomics model outperformed
multiple conventional models (visual assessment, histogram-based assessment, and
average Hounsfield unit) in identifying advanced atherosclerotic lesions. In a
subsequent study, Kolossváry et al. [30] applied CCTA-based
radiomics to identify vulnerable plaques in 44 plaques in 25 patients. The study
showed that radiomics was superior to conventional CCTA parameters in identifying
IVUS-validated attenuated plaques, OCT-defined thin-cap fibroatheroma, and
increased NaF
In addition, coronary inflammation can be measured on CCTA using deep learning
methods, and it has been developed as a biomarker named the fat attenuation index
(FAI) [65]. The perivascular FAI around culprit lesion precursors increases
significantly in patients with ACS compared to non-culprit lesions [66]. FAI was
also reported to be associated with NaF
It has been thought that most ACS results from the rupture of nonobstructive
plaques on angiography months to years before the event [69, 70, 71, 72]. Serial invasive
angiography studies showed that nonobstructive lesions can progress rapidly
before the acute event occurs [71, 72, 73]. In addition, natural history studies using
intravascular imaging also found plaques with high-risk features, including
lipid-rich plaques, positive vessel remodeling, and thin-cap fibroatheroma, had a
higher probability of progressing [74, 75]. Prior CCTA studies demonstrated that
in patients with plaque progression, ACS events are substantially higher compared
to patients without progression (14.3% vs. 0.3%, p
CCTA enables earlier identification of atherosclerosis and the monitoring of dynamic changes in plaque composition [50]. However, since the composition of each coronary plaque is so diverse, conventional qualitative and quantitative baseline CCTA analysis might be limited in detecting plaques at risk of rapid progression. Han et al. [77] applied an ML framework incorporating clinical variables and qualitative and quantitative CCTA plaque features to identify individuals with a higher risk of subsequent plaque progression. The ML model showed higher diagnostic performance in predicting individuals with rapid plaque progression compared to clinical and laboratory models. It should be noted that in this study, plaque progression analysis was based on per-patient level, which pooled the plaque volumes across the major arteries. However, prior studies have shown that the patterns of plaque progression are variable among the major coronary arteries [78]. The identification of specific signatures for predicting plaque progression at a per-lesion level on CCTA is more optimal and has been investigated in many intravascular studies [4, 5, 79]. Novel techniques, such as radiomics, perivascular adipose tissue density, and hemodynamics combined with ML, may provide a better understanding of plaque progression.
Intravascular imaging modalities have been established as the gold standard for assessing the clinically relevant progression of atherosclerosis [80, 81]. Several large clinical trials evaluating the dynamic changes in coronary plaques in response to various treatments have made use of these invasive techniques [82, 83]. However, the target populations in these studies were limited to the inclusion of patients at higher risk. The utility of CCTA in evaluating plaque volume and high-risk features has made it possible to extend its application to monitor the response of various therapies in lower-risk groups.
Several cohort studies and a few randomized controlled trials (RCTs) have been performed to assess temporal changes in plaque volumes with serial CCTA. In a prospective observational study of 121 psoriasis patients, Elnabawi et al. [84] found biologic treatment was associated with a significant reduction of noncalcified plaque burden compared to non-biologic treatment at 1-year follow-up CCTA. The international PARADIGM (Progression of Atherosclerotic Plaque Determined by Computed Tomographic Angiography Imaging) trial evaluated the use of statins-induced changes in CCTA-based plaque analysis among 1255 patients [85]. The authors found that statin therapy was related to rapid progression of calcified plaque volume but slower progression of overall plaque volume [85]. Budoff et al. [86] conducted an RCT to explore the impact of icosapent ethyl on plaque characterization using CCTA. Eighty patients were randomized to receive either icosapent ethyl or placebo. After 18 months of follow-up, LAP volume was reduced by 17% in the icosapent ethyl group, while the placebo group experienced an increase in LAP volume (109%; p = 0.0061) [87]. These data suggest the possibility of applying CCTA in monitoring dynamic changes in plaques over time. With the development of future fully automated plaque analysis software, plaque volumes, and high-risk features could be easily compared between serial scans. CCTA may help to provide more accurate treatment decisions in daily clinical practice.
Current CAD prevention guidelines recommend the use of population-based risk calculators, such as the Pooled Cohort Equations and SCORE 2, to estimate a 10-year risk of cardiac events [87, 88]. However, predicting outcomes among patients on an individual level remains challenging.
AI has the potential to provide personalized risk evaluation using the
integration of multiple quantitative imaging and clinical variables [89]. Several
studies have focused on using AI to predict clinical outcomes in both
asymptomatic and symptomatic patients [34, 35, 90]. In a large sample study of
66,636 asymptomatic patients, a comprehensive ML model incorporating 46 clinical
variables and 31 non-contrast CT measurements was superior for the prediction of
10-year coronary heart disease and cardiovascular disease deaths (AUC = 0.86)
compared to the coronary artery calcium score (AUC = 0.82), atherosclerotic
cardiovascular disease (ASCVD) score (AUC = 0.83) and CT variables alone (AUC =
0.83) (all p
In cardiovascular imaging, AI algorithms have the potential to improve clinical workflow, accelerate post-processing time, provide objective and accurate results, and guide patient management [13]. Clinically available AI-assisted software for assessing stenosis severity has been shown to be accurate and fast. Integration of fractional flow reserve derived from CCTA (CT-FFR) will further guide the subsequent interventional therapy. Fully automated plaque quantification software is under development, which would expand the potential for CCTA in the evaluation of progression and guide the treatment of atherosclerosis. Radiomics, with automated plaque segmentation, feature extraction, and ML analyses, may increase the diagnostic accuracy and precision of high-risk plaque in routine clinical practice. Finally, personalized AI-based risk scores integrating a vast number of clinical and imaging metrics will be calculated in real time, identifying high-risk patients and providing long-term risk evaluation for future cardiac events.
While AI holds great promise for improving current atherosclerosis imaging, there are several barriers to be addressed and resolved. First, the lack of transparency impedes AI adoption in clinical decision-making. Explanations regarding AI results need to be offered to clinicians and patients. The development of explainable AI approaches is an answer to this limitation [92]. For example, SHAP uses a game theory approach to determine the overall importance of variables and explain how the variables impact individual predictions. Second, many current AI algorithms are developed on small datasets with low variability regarding both image acquisition and patient demographics. These AI algorithms could perform well in the development stage but not generalize well in other datasets. Robust validation with external datasets and prospective, real-world, varied datasets is desired before applying AI tools in clinical practice. Third, adequate evaluation of AI algorithms before adoption in clinical is critical [93]. Despite numerous AI algorithms that have been developed and shown promising outcomes, there are few cardiovascular AI technologies validated in current RCTs [94]. The human-AI interaction and the effect of AI in the radiologic workflow are poorly understood in current clinical studies. An RCT is the best way to determine the actual clinical value of an AI system in which one arm receives AI-assisted care and the other receives usual care [95].
AI-assisted CCTA has the potential for a comprehensive assessment of atherosclerotic plaque characterization by minimizing human error and saving interpreting time. AI can be also used to provide personalized risk stratification for CAD patients by integrating multiple quantitative imaging and clinical data. However, the explainability, robustness, and generalizability of AI need to be addressed before its wide acceptance in the clinic. Future studies are expected to validate the clinical impact of AI algorithms in daily practice.
AI, artificial intelligence; CAD, coronary artery disease; MACE, major adverse cardiac events; ACS, acute coronary syndrome; IVUS, intravascular ultrasound; OCT, optical coherence tomography; CCTA, coronary CT angiography; ML, machine learning; DL, deep learning; CNNs, convolutional neural networks; NLP, natural language processing; LAP, low attenuation plaque; FDA, Food and Drug Administration; ICC, intra-class correlation; HR, hazard ratio; AUC, area under the curve; ASCVD, atherosclerotic cardiovascular disease; ECG, electrocardiogram; CVD, cardiovascular disease; CHD, congenital heart disease.
Among the authors in the list, LJZ and HX designed the research study and revised it critically for important intellectual content. QC, FZ and CXT searched and organized the literature, was the main drafter of the manuscript and critically revised the important content. XG, GX, YZ and XY participated in the collation and analysis of the literature and provided advice on revising the structure of the manuscript. LJZ contributed to funding acquisition. All authors read and approved the final manuscript. All authors have participated sufficiently in the work and agreed to be accountable for all aspects of the work.
Not applicable.
Not applicable.
This research was supported by the Jiangsu Province Key Project of Comprehensive Prevention and Control of Chronic Diseases (BE2020699).
The authors declare no conflict of interest.
Publisher’s Note: IMR Press stays neutral with regard to jurisdictional claims in published maps and institutional affiliations.