- Academic Editor
†These authors contributed equally.
Background: Acute kidney injury (AKI) frequently occurs after aortic
surgery and has a significant impact on patient outcomes. Early detection or
prediction of AKI is crucial for timely interventions. This study aims to develop
and validate a novel model for predicting AKI following aortic surgery.
Methods: We enrolled 156 patients who underwent on-pump aortic surgery
in our hospital from February 2023 to April 2023. Postoperative levels of eight
cytokines related to macrophage polarization analyzed using a multiplex cytokine
assay. All-subset regression was used to select the optimal cytokines to predict
AKI. A logistic regression model incorporating the selected cytokines was used
for internal validation in combination with a bootstrapping technique. The
model’s ability to discriminate between cases of AKI and non-AKI was assessed
using receiver operating characteristic (ROC) curve analysis. Results:
Of the 156 patients, 109 (69.87%) developed postoperative AKI. Interferon-gamma
(IFN-
Acute kidney injury (AKI) is one of the most common complications following aortic surgery and elevates the risks for: postoperative mortality, renal replacement therapy (RRT), prolonged intensive care unit (ICU) and hospital stay, higher medical costs, and a continued risk of death 10 years after surgery [1, 2, 3]. Early identification of high-risk AKI patients is crucial due to the condition’s rapid progression and poor prognosis. While serum creatinine and urine output are standard diagnostic markers, their limitations, their predictive value may be limited due to their delayed rise, and the fact that urine output may not be a reliable indicator during the polyuric phase [4, 5]. Therefore, alternative biomarkers based on the pathophysiological features of AKI for early risk identification are needed. In addition to some well-studied urinary biomarkers such as neutrophil gelatinase-associated lipocalin (NGAL), insulin-like growth factor binding protein-7 (IGFBP-7), and tissue inhibitor of metalloproteinase-2 (TIMP-2) [6, 7, 8, 9, 10, 11], blood biomarkers involved in the immune-inflammatory phase of AKI have also been identified and warrant further research.
Previous research has demonstrated that the innate immune response is closely
linked to the pathogenesis of renal ischemia-reperfusion injuries [12]. Among the
innate immune cells involved in this process, macrophages have been found to play
a complex role throughout the development of AKI via their polarization to either
a “pro-inflammatory” or “anti-inflammatory” phenotype [13]. Several animal
studies have confirmed that macrophage polarization is involved in the initiation
and repair of AKI, and influences the outcome [14, 15, 16]. Macrophage polarization
can be induced by various cytokines such as interferon-gamma (IFN-
Despite their potential, these biomarkers that were individually selected to predict AKI, have demonstrated limited sensitivity and specificity in clinical practice. Moreover, these existing studies were limited to specific aortic disease groups, lacking independent validation in broader populations. Therefore, utilizing a more comprehensive set of macrophage polarization-related cytokines in a cohort of patients with multiple aortic diseases, and integrating multiple cytokines into a simple model may improve the prediction of AKI risk assessment.
In this study, we conducted a systematic analysis of eight macrophage polarization-related cytokines in plasma samples from adult patients who underwent aortic surgery with cardiopulmonary bypass (CPB). We used all-subset regression to identify promising cytokines for inclusion in a model aimed at predicting postoperative AKI. In addition, we assessed the ability of each cytokine of interest to determine the risk of AKI as well as in-hospital outcomes.
This single-center, retrospective, observational study was performed at the Fuwai Hospital (National Center of Cardiovascular Diseases, Beijing, China). We enrolled 156 adult patients who underwent aortic surgery with CPB at the Fuwai Hospital between February 2023 and April 2023. Exclusion criteria included: (1) patients under 18 years of age or over 80 years of age; (2) the presence of comorbidities including urinary tract infection or obstruction, or chronic kidney disease; (3) a recent history of a kidney transplant or dialysis; (4) the use of medications with nephrotoxic effects two weeks before surgery; (5) severe rheumatic immune disease; (6) immunodeficiency syndromes. We obtained the clinical data from the medical records. Demographic and preoperative data included age, sex, body mass index (BMI), comorbidities, preoperative cardiovascular status, preoperative serum creatinine (last value before cardiac surgery), and the diagnosis of aortic disease. Operative details included type of surgery, CPB time, and arterial clamp times. Postoperative serum creatinine was obtained within 48 hours of surgery. This research was approved by the Institutional Ethics Committee of Fuwai Hospital (No. 2023-2005) and was conducted under the tenets of the Declaration of Helsinki. Written informed consent was obtained from all patients before enrollment.
Blood samples were taken between 12 and 24 hours following surgery in the
morning (8:00 AM) of the first postoperative day to evaluate cytokines.
Using a multiplex Bio-Plex Pro Human Cytokines Assay (Bio-Rad, Hercules, CA,
USA), eight macrophage polarization-related cytokines (including IFN-
AKI was defined in accordance with the Acute Kidney Injury Network (AKIN) guidelines: a greater than 50% rise in serum creatinine, or a more than 0.3 mg/dL (26.5 mol/L) increase in serum creatinine within 48 hours of aortic surgery, in comparison to baseline [21]. Baseline was defined as the minimum creatinine level 24 hours before aortic surgery. The stages of AKI depended on the variations in serum creatinine from the baseline creatinine and are shown in Supplementary Table 1. Composite outcomes included RRT and/or in-hospital death. Length of ICU stay, length of hospital stay, and time spent on mechanical ventilation were considered to be associated outcomes.
Unpaired Student’s t-test or the Mann-Whitney U test were used to
compare continuous variables, and were reported as medians with interquartile
ranges. The chi-squared test or Fisher’s exact test was used to compare
categorical variables that were reported as numbers and proportions. Analysis of
variance or the Kruskal-Wallis test, if applicable, was used to assess
differences between the three groups. Using univariate logistic regression
analysis, the correlation between each cytokine and AKI was determined.
The logistic regression model contained all
variables that met the criteria for statistical significance in the univariate
logistic regression analysis. The variables were screened using all-subsets
regression, with the best model assessed by adjusted r-squared and Bayesian
information criterion (BIC). The area under the receiver operating characteristic
curve (AUC), which equates to the C-statistic, was calculated to assess the
model’s discriminating power. The Brier score was used to evaluate the model’s
calibration. The model was internally validated via bootstrapping. The net
benefit and improvement of the model over the clinical factor-based model were
compared using decision curve analysis (DCA), net reclassification improvement (NRI),
and integrated discriminant improvement (IDI). In each analysis,
statistical significance was defined as 2-tailed p
In our cohort of 156 adult patients undergoing aortic surgery, 109 (69.87%) developed postoperative AKI. Among them, 63 patients (40.38%) experienced moderate AKI (AKIN stage 1) and 46 (29.49%) had severe AKI (AKIN stage 2–3). Renal replacement therapy (RRT) was required for 18 patients (11.53%), and all 7 in-hospital deaths all occurred in the AKI group; there were no deaths in the non-AKI group. Baseline characteristics, intraoperative details, and perioperative outcomes are outlined in Table 1. There were no significant differences in age, sex, BMI, smoking, diabetes mellitus, hyperlipidemia, preoperative left ventricular ejection fraction, preoperative serum creatinine, arterial occlusion time, and hospital days between the AKI and non-AKI groups. In contrast, hypertension, cardiac function (as classified by the New York Heart Association), diagnosis of aortic disease, type of surgery, and CPB duration were significantly different between the two groups. Patients in the AKI group had an increased incidence of prolonged ventilation time and prolonged ICU stay. The distribution of surgical procedures is shown in Supplementary Table 2. Amongst the procedures, 47 (30.13%) were simple aortic root procedures, 18 (11.54%) were simple aortic arch procedures, 9 (5.76%) were simple descending aortic procedures, and 82 (52.57%) were combined procedures involving at least two sites in the aortic root, ascending aorta, arch, and descending aorta.
Non-AKI group (n = 47) | AKI group (n = 109) | p value | |||
Number (Proportion) | Number (Proportion) | ||||
Median (Q1–Q3) | Median (Q1–Q3) | ||||
Demographic data | |||||
Age, years | 57.00 (48.00–63.00) | 56.00 (47.00–65.50) | 0.49 | ||
Male, n % | 34 (72.34%) | 84 (77.06%) | 0.52 | ||
BMI, kg/m |
24.91 (22.59–27.68) | 25.95 (23.12–27.55) | 0.58 | ||
Comorbidities | |||||
Smoking, n % | 24 (51.06%) | 54 (49.54%) | 0.86 | ||
Diabetes Mellitus, n % | 5 (10.63%) | 5 (4.58%) | 0.28 | ||
Hyperlipidemia, n % | 25 (56.81%) | 49 (44.95%) | 0.18 | ||
Hypertension, n % | 20 (42.55%) | 80 (73.39%) | |||
Preoperative cardiovascular status | |||||
Preoperative LVEF, n % | 61.00 (56.00–63.00) | 60.00 (58.50–64.50) | 0.66 | ||
NYHA III–IV, n % | 3 (6.38%) | 24 (22.01%) | 0.018 | ||
Baseline renal function | |||||
Preoperative serum creatinine, µmol/L | 80.80 (75.00–97.17) | 88.00 (75.90–103.60) | 0.28 | ||
Disease diagnosis | 0.005 | ||||
Aortic aneurysm, n % | 30 (63.82%) | 43 (39.44%) | |||
Aortic dissection: Stanford A, n % | 11 (23.40%) | 55 (50.45%) | |||
Aortic dissection: Stanford B, n % | 1 (2.12%) | 5 (4.58%) | |||
Other, n % | 5 (10.63%) | 6 (5.50%) | |||
Marfan Syndrome, n % | 1 (2.12%) | 2 (1.83%) | 1.00 | ||
Operative details | |||||
Surgery types | |||||
Surgery simply referring to the aortic root, n % | 25 (53.19%) | 22 (32.83%) | |||
Surgery simply referring to the aortic arch, n % | 4 (8.51%) | 14 (12.57%) | |||
Surgery simply referring to descending aorta, n % | 5 (10.63%) | 4 (6.28%) | |||
Combined surgery, n % | 13 (27.65%) | 69 (57.29%) | |||
Concomitant surgery | |||||
CABG, n % | 6 (12.76%) | 21 (19.26%) | 0.32 | ||
Mitral or tricuspid valve surgery, n % | 1 (2.12%) | 9 (8.25%) | 0.28 | ||
CPB time, min | 121.00 (81.25–152.25) | 149.50 (120.50–201.75) | 0.001 | ||
CPB time |
15 (31.91%) | 60 (55.04%) | 0.008 | ||
Artery clamp time, min | 93.00 (61.50–122.50) | 99.00 (73.00–133.00) | 0.29 | ||
Outcomes | |||||
48 h highest serum creatinine, µmol/L | 86.54 (76.20–105.08) | 173.81 (146.87–227.74) | |||
Hospitalization, days | 15.00 (11.00–21.00) | 15.00 (12.00–25.00) | 0.42 | ||
ICU time, days | 3.00 (1.50–4.00) | 5.00 (2.00–8.00) | 0.005 | ||
Ventilation, hours | 16.00 (13.00–21.00) | 25.00 (16.00–64.50) | 0.001 | ||
RRT, n % | 0 (0.0%) | 18 (16.51%) | 0.003 | ||
In hospital death, n % | 0 (0.00%) | 7 (6.42%) | 0.10 |
AKI, acute kidney injury; BMI, body mass index; LVEF, left ventricular ejection fraction; NYHA, New York Heart Association; CABG, coronary artery bypass graft; CPB, cardiopulmonary bypass; ICU, intensive care unit; RRT, renal replacement therapy; Other aortic diseases include aortic penetrating ulcer, aortic intramural hematoma, and pseudoaneurysm; Combined surgery, procedures that involved at least two sites among the aortic root, ascending aorta, arch, and descending aorta.
To analyze the distinct feature of macrophage polarization-related cytokines
between the AKI group and the non-AKI group, we assessed the levels of 8
cytokines in our cohort, comparing the
concentrations between the groups. As shown in Fig. 1, the AKI group exhibited
significantly higher concentrations of seven of the eight cytokines, with
IL-1
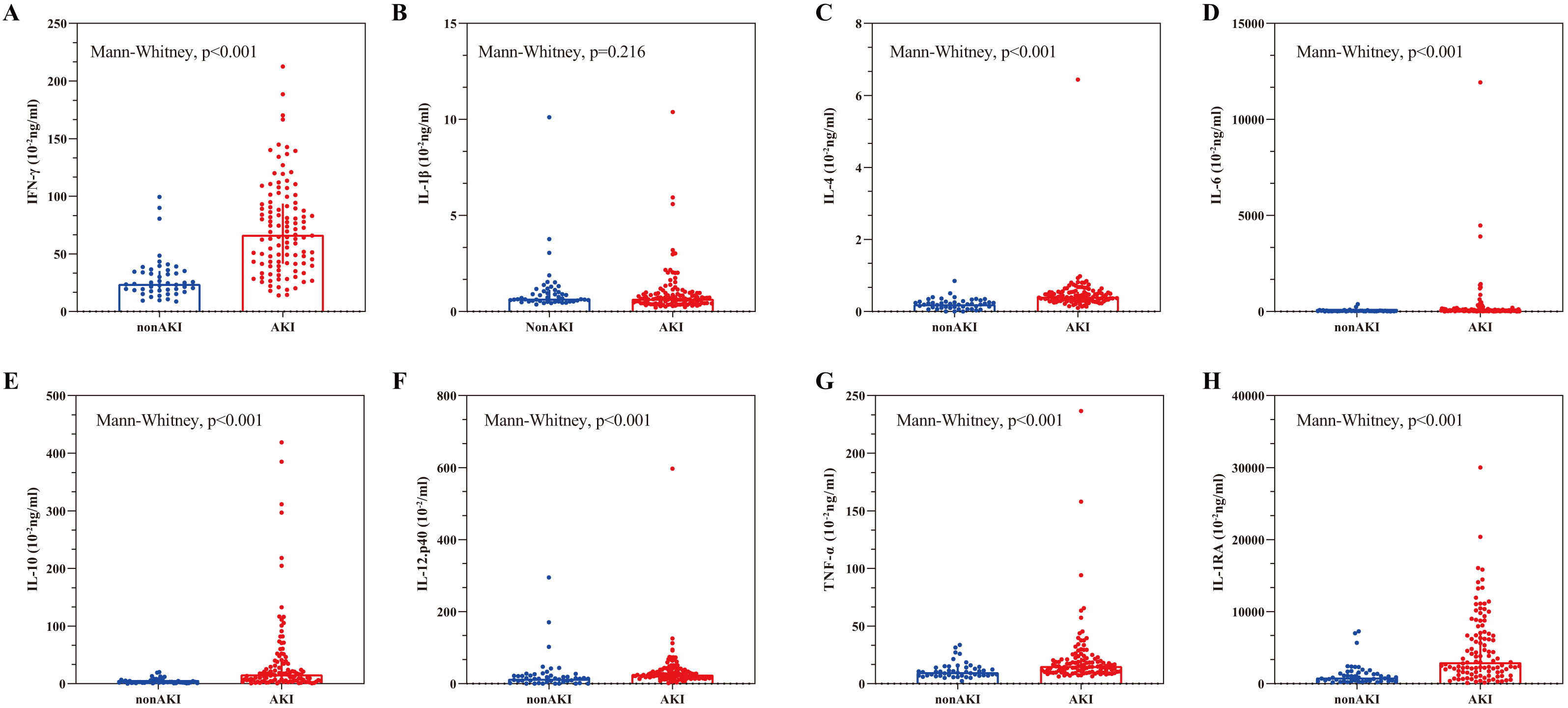
Comparisons of cytokine concentrations between AKI and non-AKI
groups. (A–H) The figure sequentially presents the concentrations of
IFN-
Factors | OR | 95% CI | p value |
lnIFN- |
13.55 | 5.77–31.81 | |
lnIL-1 |
0.76 | 0.46–1.25 | 0.280 |
lnIL-4 | 15.89 | 5.87–43.04 | |
lnIL-6 | 1.92 | 1.32–2.80 | 0.001 |
lnIL-10 | 2.94 | 1.97–4.37 | |
lnIL-12p40 | 1.97 | 1.23–3.16 | 0.005 |
lnTNF- |
6.41 | 2.75–14.94 | |
lnIL-1RA | 2.81 | 1.91–4.13 |
lnIFN-
We included these seven cytokines in an all-subsets regression analysis to
identify the most predictive model for postoperative AKI. The model performance
was evaluated using the adjusted r-squared value and Bayesian Information
Criterion (BIC) values as shown in Fig. 2. Although the model including IL-4,
IL-10, IFN-
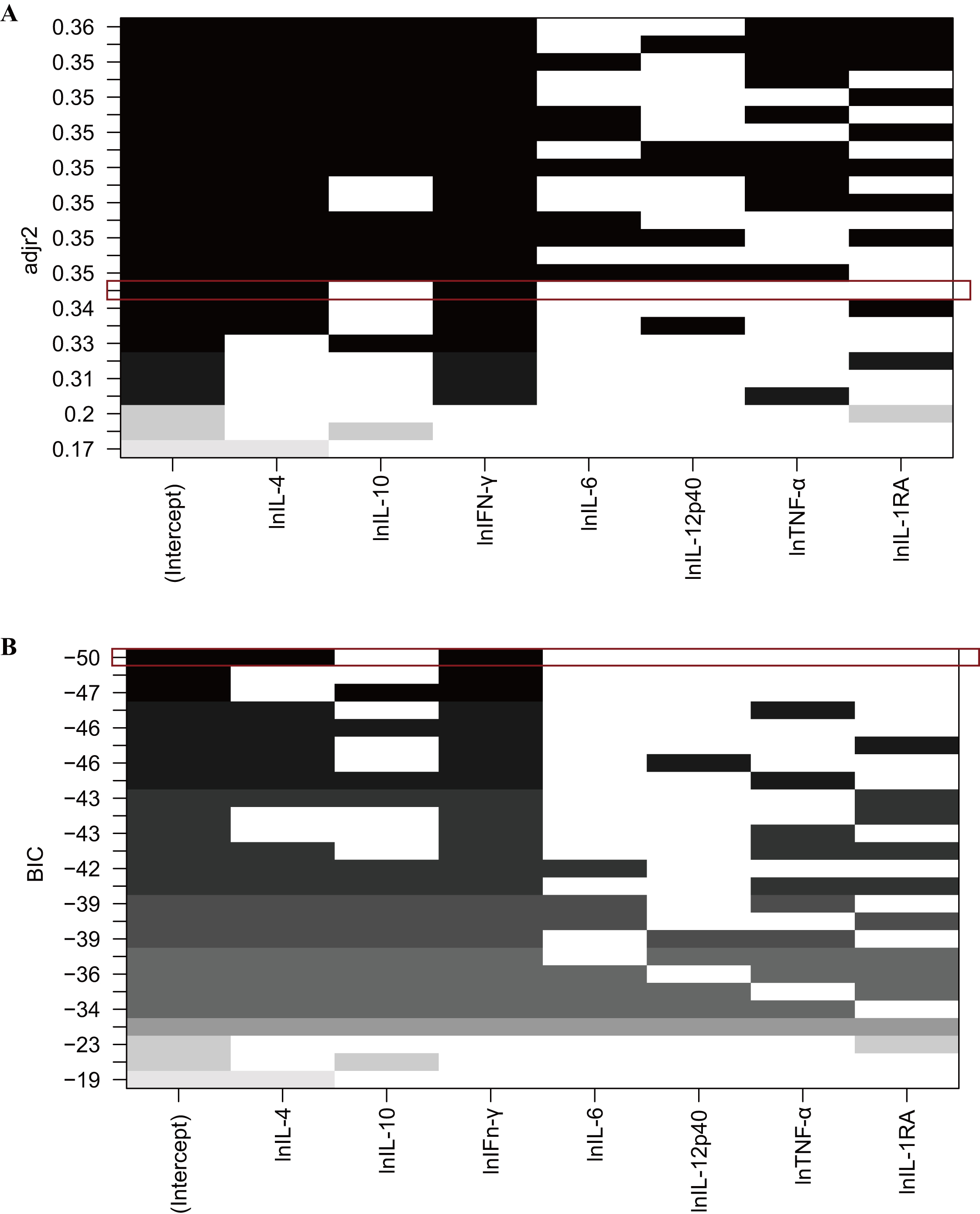
Model selection using adjusted r-squared value (A) and BIC value
(B). The empty bars signify variables not included in the models, while the gray
and black bars indicate the included variables. (A) Displays the adjusted
r-squared values of each model. For example, the red box highlights a model
incorporating lnIL-4 and lnIFN-
Cytokine-based model | Estimate | SE | Z values | p value |
Intercept | –3.373 | 1.970 | –1.712 | 0.086 |
lnIFN- |
1.871 | 0.547 | 3.418 | |
lnIL-4 | 1.829 | 0.471 | 3.880 |
SE, standard error; lnIFN-
A nomogram for the prediction of postoperative AKI in patients undergoing aortic
surgery was constructed (Fig. 3A). The nomogram and was based on the regression
coefficients and the intercept in the model featuring IFN-
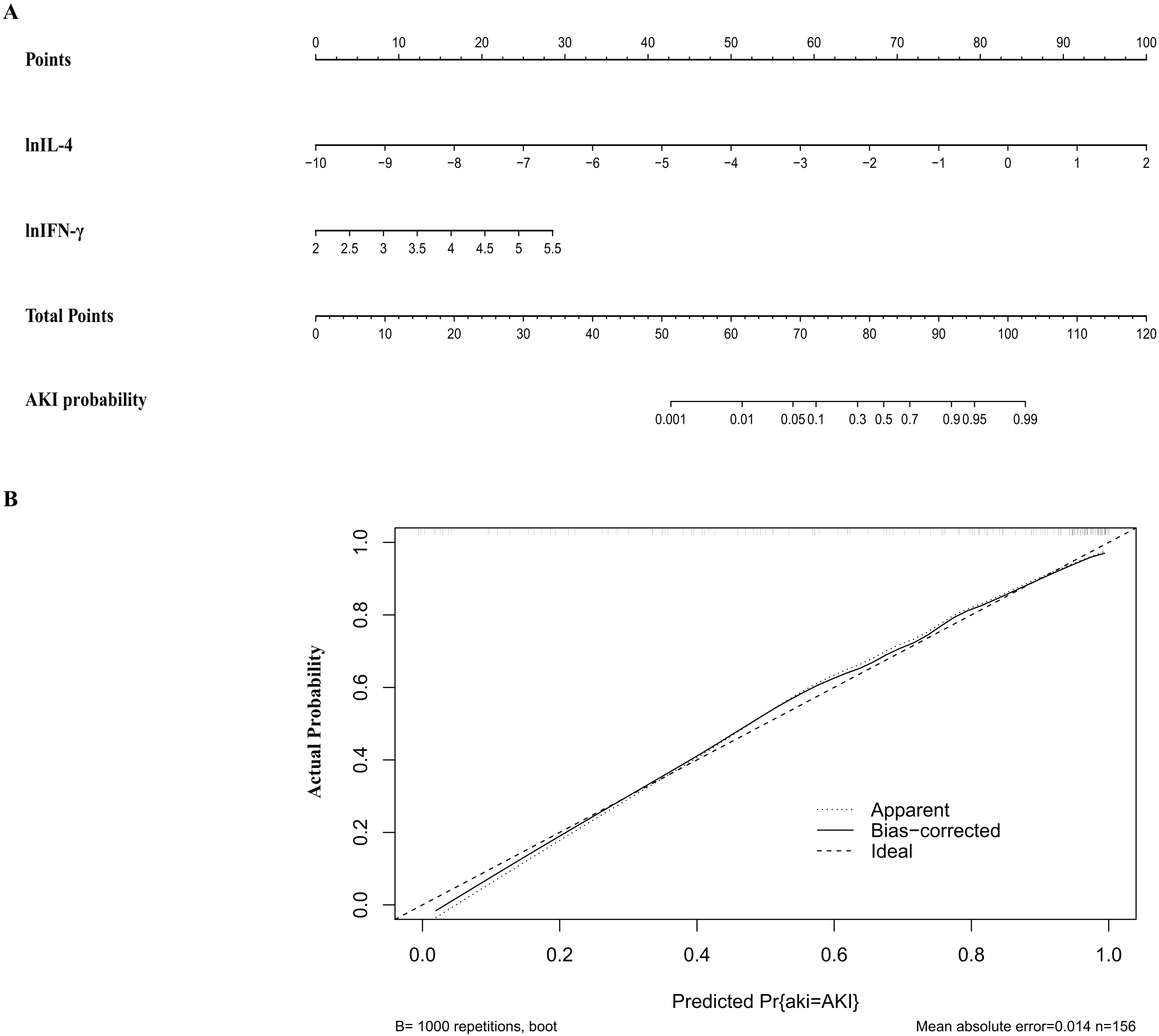
Nomogram (A) and calibration curve (B) of the cytokine-based
model. (A) Nomogram for cytokine-based AKI prediction. Logarithmic
transformation is recommended for IL-4 and IFN-
The cytokine-based predictive model was validated internally by the bootstrapping method (1000 resamples). This confirmed the model’s strong discriminative power, reflected by a closely matching corrected C-statistic of 0.89 (Table 4). The calibration curve for the cytokine-based model illustrated a strong congruence between the model’s predicted AKI risk and the actual AKI in the study patients (Fig. 3B).
Models | AIC | BIC | C-statistics | Corrected C-statistics | Brier | Sensitivity | Specificity | Categorical NRI | IDI |
Cytokine-based model | 115.75 | 124.90 | 0.90 (0.85–0.96) | 0.89 (0.84–0.95) | 0.11 | 0.78 | 0.89 | 0.43 (0.23–0.63) p |
0.34 (0.23–0.45) p |
Clinical factor-based model | 179.48 | 194.73 | 0.72 (0.63–0.82) | 0.69 (0.60–0.79) | 0.18 | 0.79 | 0.60 | ref | ref |
Cytokine-based model: lnIFN-
Previous studies [22, 23, 24, 25, 26] have found associations between clinical variables such as age, history of hypertension, preoperative blood creatinine, prolonged CPB time, and elevated AKI risk of AKI following aortic surgery. We next compared the predictive ability of these clinical factor-based models was to our cytokine-based model (Table 4).
Compared to traditional clinical factor-based models for predicting
postoperative AKI, our cytokine-based model demonstrated superior performance
across multiple evaluation metrics. Specifically, the cytokine-based model has a
superior goodness of fit score (akaike information criterion [AIC]: 115.75 vs.
179.48; BIC: 124.90 vs. 194.73). Furthermore, the model has improved calibration,
with a Brier score of 0.11 compared to 0. 18 for the clinical model. The
discrimination performance was also improved, yielding a C-statistic of 0.90
(95% confidence interval [CI]: 0.85–0.96) vs. 0.72 (95% CI: 0.63–0.82).
Notably, the cytokine-based approach significantly enhanced patient
reclassification, with a net reclassification improvement (NRI) of 0.43 (95% CI:
0.23–0.63, p
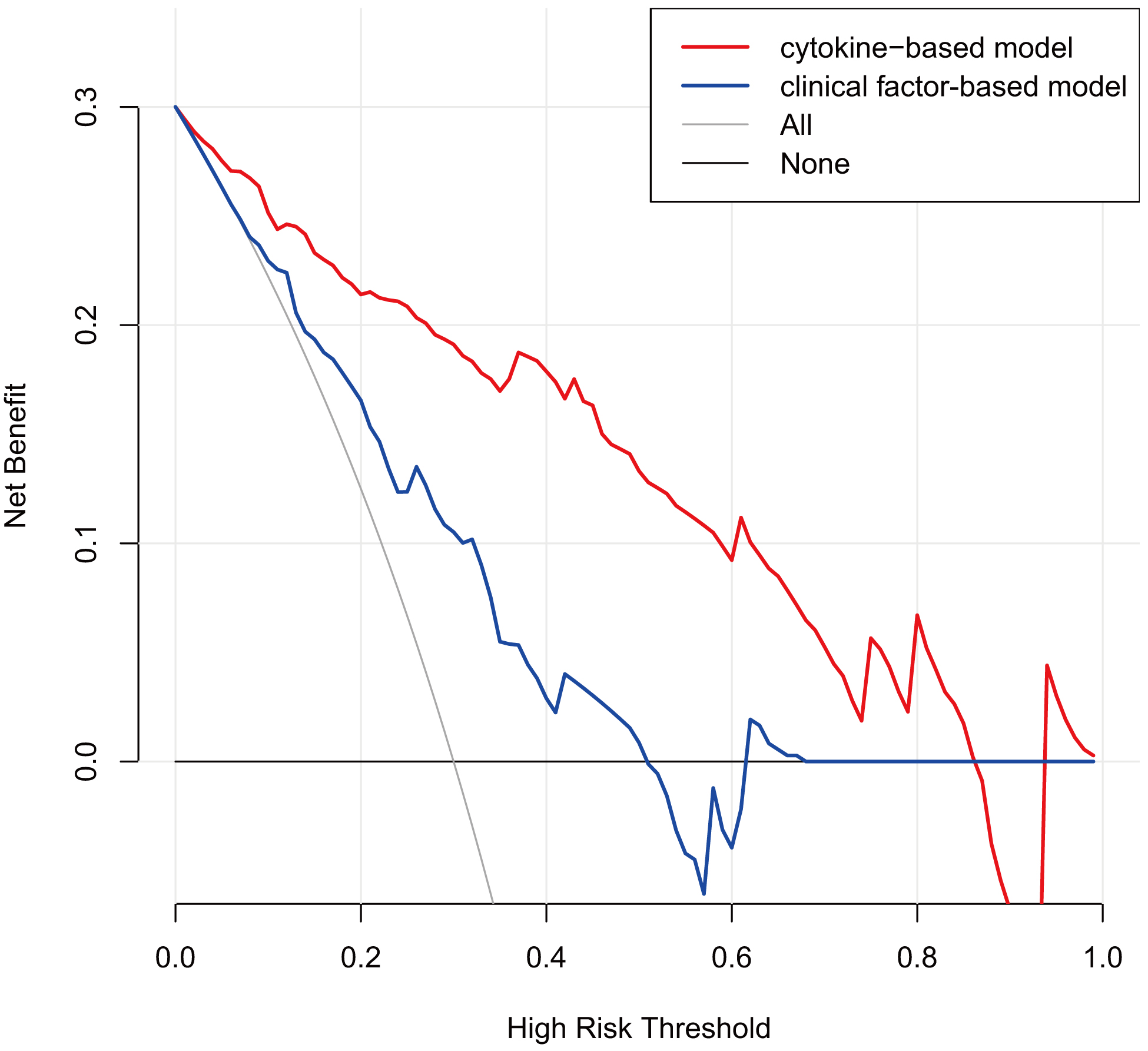
Decision curve analysis for comparing the cytokine-based model
and clinical factor-based model. Cytokine-based model: lnIFN-
We assessed the generalizability of our cytokine-based AKI prediction model,
partly due to the male-dominated gender distribution, wide age, and surgical
complexity. The cytokine-based model was used to predict AKI across a range of
age, gender, and surgical type groups (Table 5). The model showed robust
predictive power in both genders, albeit with slightly superior discrimination in
females (AUC: 0.96 vs. 0.88; Brier: 0.08 vs. 0.11). Similarly, the cytokine-based
model performed well in both the older group (age
Groups | AKI/non-AKI | AUC | Brier |
Male group | 84/34 | 0.88 | 0.11 |
Female group | 25/13 | 0.96 | 0.08 |
Age |
44/19 | 0.91 | 0.09 |
Age |
65/28 | 0.89 | 0.12 |
Aortic root/aortic arch/descending aorta surgery group | 40/34 | 0.92 | 0.01 |
Combined surgery group | 69/13 | 0.89 | 0.08 |
AUC, the area under the receiver operator characteristic curve; AKI, acute kidney injury; Combined surgery, procedures involving at least two sites among the aortic root, ascending aorta, arch, and descending aorta.
We evaluated the individual discriminative ability of IL-4 and IFN-
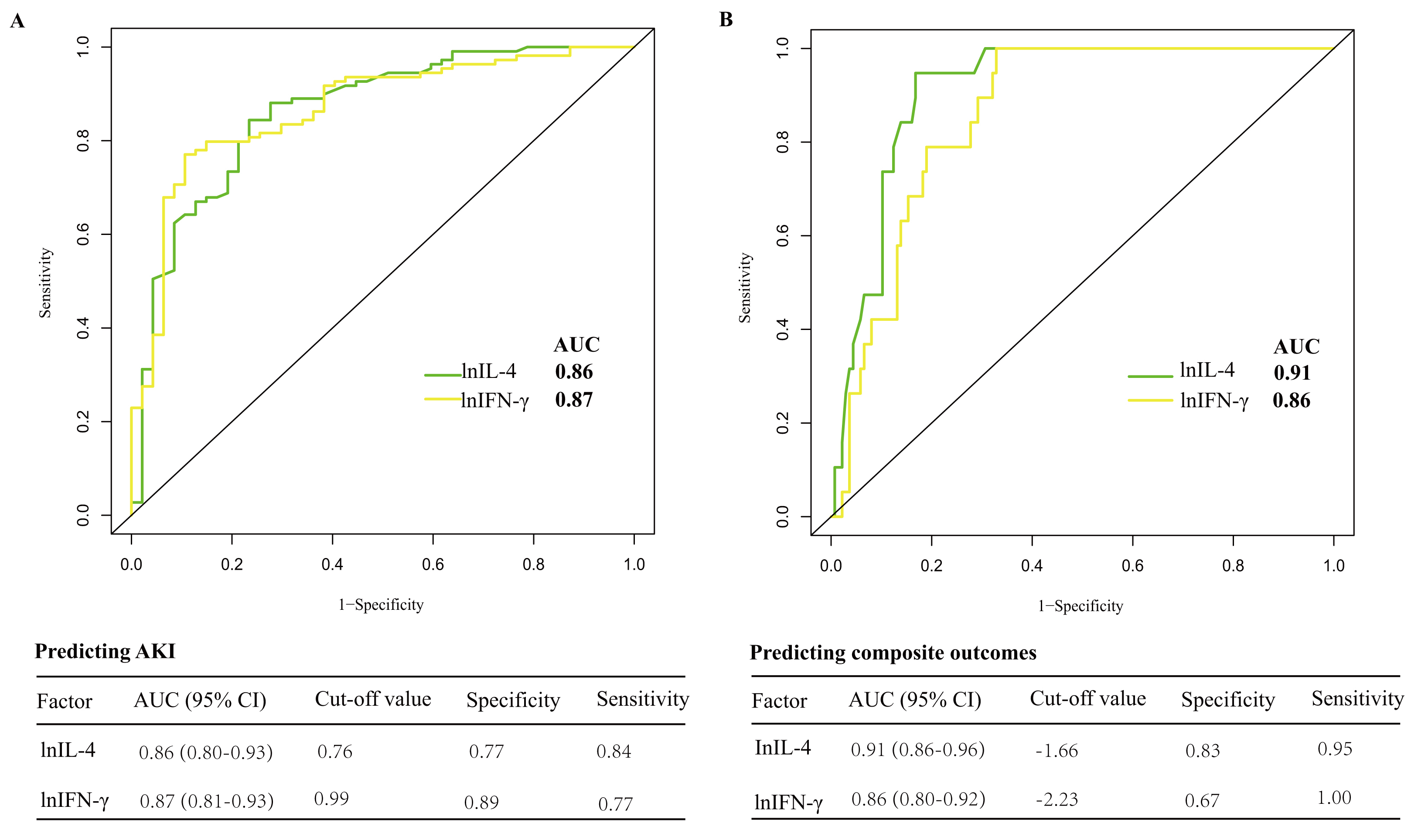
Discriminability of selected cytokines for AKI (A) and composite
outcomes (B). (A) In predicting AKI, the AUC for lnIL-4 was 0.86, with a
specificity of 0.77 and a sensitivity of 0.84; the AUC for lnIFN-
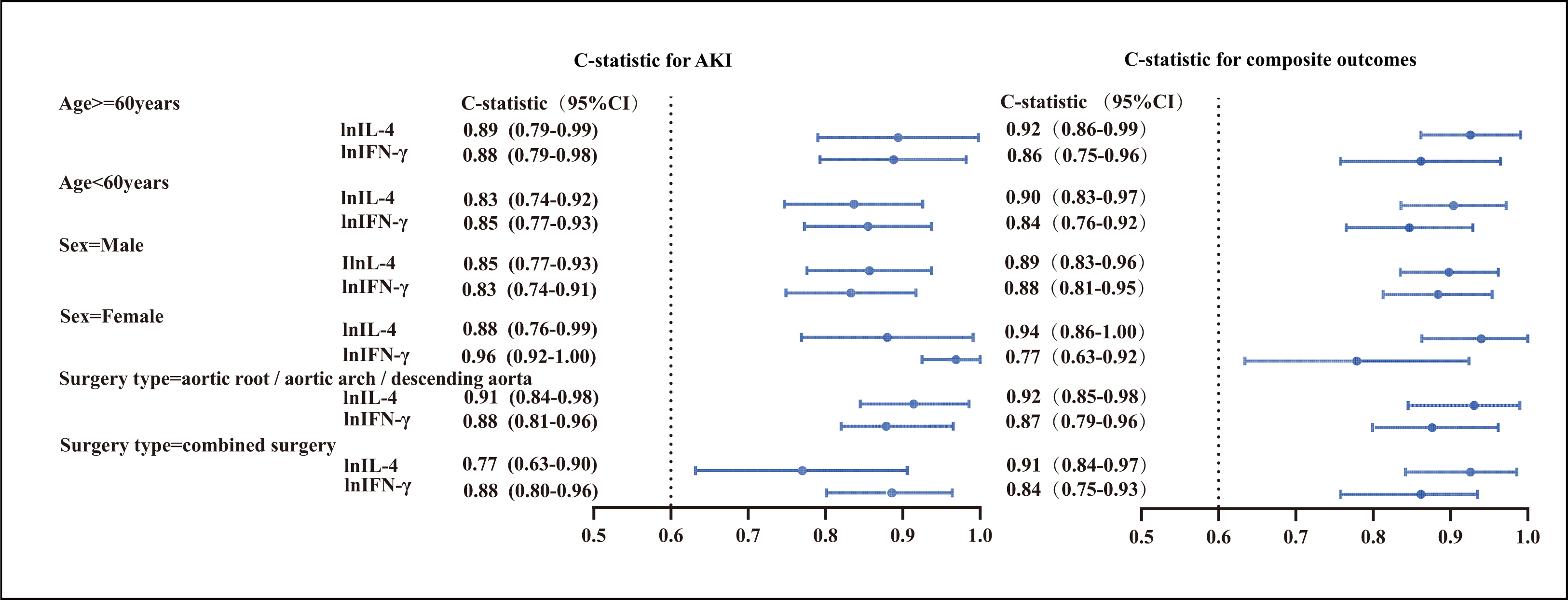
Discriminability of selected cytokines for AKI and composite
outcomes in stratified groups based on age, gender, and surgery type.
C-statistics and 95% CIs of cytokines (IFN-
We further evaluated the performance of the 2 cytokines in stratifying
postoperative AKI risk. As presented in Table 6, elevated concentrations of
IFN-
Cytokines | Crude odds ratios (95% CI) | p value | Adjusted I odds ratios (95% CI) | p value | Adjusted II odds ratios (95% CI) | p value |
IFN- |
||||||
Tertile 1 | ref | ref | ref | |||
8.70–33.23 (10 |
||||||
Tertile 2 | 6.47 (2.71–15.49) | 6.71 (2.74–16.41) | 12.39 (3.63–42.20) | |||
32.23–74.25 (10 | ||||||
Tertile 3 | 28.37 (7.77–103.59) | 32.10 (8.47–121.60) | 55.32 (10.65–287.33) | |||
74.25–212.58 (10 |
||||||
p for group trend | ||||||
IL-4 group | ||||||
Tertile 1 | ref | ref | ref | |||
0–0.27 (10 |
||||||
Tertile 2 | 7.46 (3.71–18.05) | 7.21 (2.96–17.53) | 5.32 (1.85–15.31) | 0.002 | ||
0.27–0.42 (10 | ||||||
Tertile 3 | 51.00 (11.07–235.07) | 54.44 (11.56–256.38) | 68.05 (10.80–428.62) | |||
0.42–6.44 (10 | ||||||
p for group trend |
Adjusted I: adjusted for age and sex. Adjusted II: adjusted for age, sex, BMI,
preoperative serum creatinine, hypertension, types of surgery, prolonged
cardiopulmonary bypass time, NYHAIII-IV. IFN-
Specifically, compared to patients in the lowest tertile of cytokine levels,
those in the upper tertiles had markedly increased adjusted odds ratios for
developing AKI. For IFN-
Additionally, the concentrations of IFN-
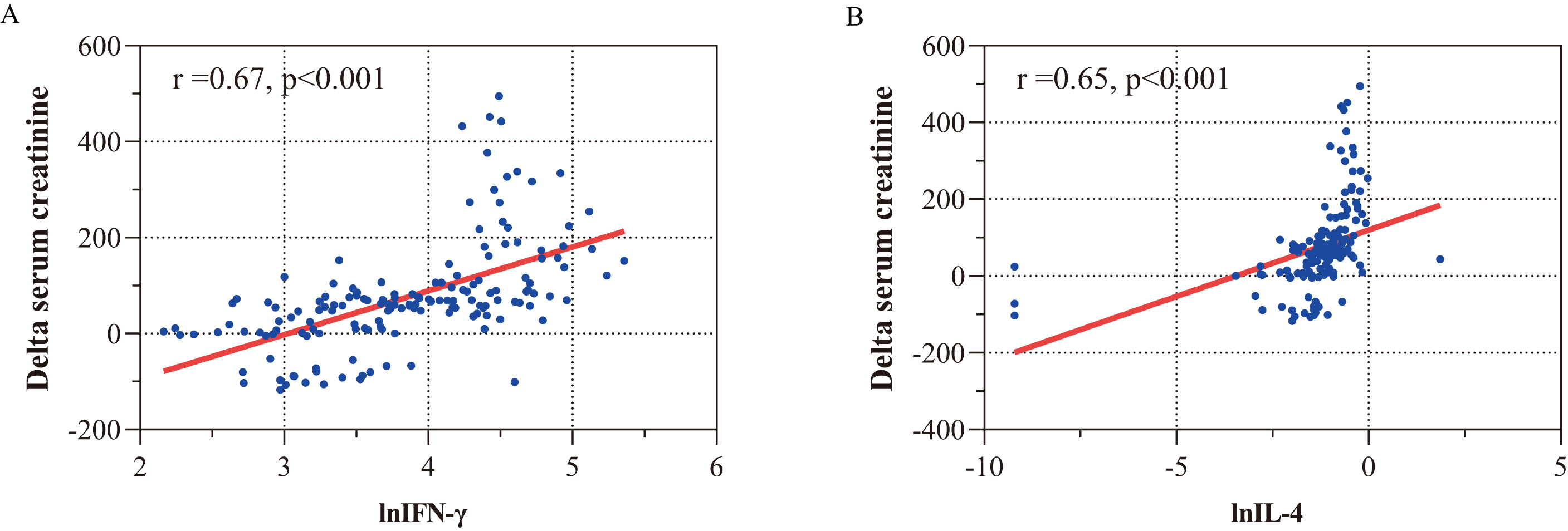
Correlations between IFN-
Cytokine | Tertiles | Hospitalization time (days) | ICU stay (days) | Ventilation time (hours) | AKIN | AKIN | Composite outcomes |
(median (IQR)) | (median (IQR)) | (median (IQR)) | stage 2 | stage 3 | (n (%)) | ||
IFN- |
Tertile 1 (8.70–33.23) | 14.00 (11.25–22.75) | 2.00 (2.00–4.00) | 16.00 (11.00–24.50) | 3 (5.77%) | 1 (1.92%) | 0 (0.00%) |
Tertile 2 (33.23–74.25) | 15.50 (12.00–23.00) | 3.00 (2.00–6.00) | 18.00 (15.75–28.75) | 6 (11.54%) | 3 (5.77%) | 4 (7.69%) | |
Tertile 3 (74.25–212.58) | 18.00 (11.25–26.50) | 6.00 (4.00–12.00) | 52.00 (24.00–156.00) | 12 (23.08%) | 21 (40.38%) | 15 (28.85%) | |
p value for group trend | 0.608 | ||||||
IL-4 (10 |
Tertile 1 (0–0.27) | 14.00 (11.00–22.00) | 3.00 (2.00–4.00) | 17.00 (15.25–29.50) | 4 (7.84%) | 0 (0.00%) | 0 (0.00%) |
Tertile 2 (0.27–0.42) | 14.50 (11.25–25.00) | 4.00 (2.00–5.25) | 19.50 (14.00–36.25) | 6 (11.54%) | 3 (5.77%) | 1 (1.92%) | |
Tertile 3 (0.42–6.44) | 18.00 (13.00–24.00) | 5.50 (2.25–13.00) | 35.00 (18.25–139.25) | 11 (20.75%) | 22 (41.51%) | 18 (33.96%) | |
p value for group trend | 0.238 |
Composite outcome, renal replacement therapy and/or in-hospital death; IQR,
interquartile range; IFN-
Our study found that elevated levels of cytokines related to macrophage
polarization, specifically IFN-
AKI is a frequent complication following aortic surgery and is strongly linked to poor clinical outcomes, including the need for RRT and increased mortality. Current diagnostic measures for AKI, including serum creatinine levels, fall short in early detection of renal tubular injury due to their delayed elevation and low sensitivity [27]. A study involving 303 patients was designed to assess the correlation between AKI (as defined by serum creatinine) and diffuse histologic criteria for acute kidney injury based on renal biopsy. Remarkably, approximately one-third of patients meeting the histological AKI criteria failed to meet AKI diagnostic criteria. This was primarily because serum creatinine did not rise quickly enough to fulfill the definition of AKI [28].
Therefore, there is a considerable need for a reliable biomarker to predict early AKI, as timely intervention can lead to the prevention of multiple clinical complications. In recent years, several novel biomarkers for AKI have been evaluated in aortic surgery. The most studied biomarkers, derived from urine or plasma samples, are related to cell cycle arrest, such as NGAL, liver-type fatty acid-binding protein (L-FABP), IGFBP-7, TIMP-2, and cystatin C [7, 8, 9, 10, 11, 29, 30, 31, 32]. A few studies have evaluated the predictive ability of blood immune-inflammatory biomarkers such as plasma secretory leukocyte peptidase inhibitor (SLPI), pentraxin 3 (PTX3), IL1-RA, MCP-1, suppressor of tumorigenicity 2 (ST-2), IL-6, and IL-10 [19, 20, 33, 34]. However, these heterogeneous studies mainly evaluated the association between an individual or multiple selected cytokines and AKI based on a small number of samples and most were limited to a specific setting, with wide variations in predictive performance. As a result, it has been suggested that these studies may have introduced a selection bias that has undermined the ability of individual biomarkers to predict AKI.
Our study differs from previous research by aiming to develop and validate a
novel robust predictive model specifically for detecting AKI in patients who
underwent aortic surgery with CPB. We evaluated eight macrophage
polarization-related cytokines (including IFN-
To objectively select the most predictive combination of cytokines for
estimating AKI among these 7 cytokines, we used all-subset regression, also known
as best subset selection. It can fit all possible combination models of
predictive variables and then select the best model under the condition of the
existing variables according to the adjusted r-squared and BIC. The adjusted
r-squared value reflects the explanatory power of the combination of predictors
on the dependent variable, while the BIC value reflects the goodness of fit of
the model. Given the high adjusted r-squared and the lowest BIC, IFN-
In clinical practice, the standard models for predicting postoperative AKI typically rely on patient clinical data. Several clinical characteristics of patients, such as age, preoperative serum creatinine, history of hypertension, poor preoperative cardiac functional status, and prolonged CPB time, have been considered risk factors for AKI after aortic surgery [22, 23, 24, 25, 26]. Zhang and his colleagues reported 4 clinical prediction models for AKI based on perioperative variables for patients with acute type A aortic dissection (ATAAD) undergoing Sun’s procedure, with the AUC ranging from 0.710 to 0.848 [35]. Kim and his colleagues [36] reported a simplified clinical score including 6 clinical variables (age, preoperative glomerular filtration rate, left ventricular ejection fraction, operation time, intraoperative urine output, and intraoperative use of furosemide) to stratify the risk of postoperative AKI in patients undergoing aortic surgery, with an AUC of 0.740. Although the variables of these prediction models were easy to collect, most of them lacked specificity, and their predictive power may largely depend on the richness of the variables. In our study, we compared a standard clinical factor-based prediction model using age, sex, hypertension history, NYHA III-IV, and prolonged CPB time to our cytokine-based prediction model described above. Consistent with the existing literature, our clinical factor-based model had an average predictive power with an AUC of 0.72, which was much lower than our cytokine-based prediction model with an AUC of 0.90. In addition, our cytokine model had many advantages over the clinical factor model in terms of patient reclassification and net clinical benefit, as well as model parsimony.
To the best of our knowledge, while both IFN-y and IL-4 are known to play
contribute towards AKI pathophysiology, their predictive value in post-surgical
AKI has not been previously documented in the existing literature.
IFN-
Since IFN-
There were some notable differences between our study and other studies reported
in the literature on this subject. For example, the serum concentrations of
IFN-
This study has multiple limitations warranting discussion. First, its retrospective, observational, and single center nature potentially introduces selection bias, rendering the findings less generalizable. Since some selection bias may be inevitable, the results cannot be considered conclusive. Second, while we conducted internal validation using 1000 bootstrap samples, external validation from a broader array of hospitals is required to confirm the model’s robustness. Third, this study had a limited sample. Since the criteria for AKI are not uniform, we could have missed some patients who had subsequently developed AKI had we followed the Acute Kidney Injury Network guideline, which only takes into account a blood creatinine rise within 48 hours following surgery. Finally, our predictive model is limited because it is based on cytokines, whose assays may not be available in some hospitals.
In patients undergoing aortic surgery with CPB, we found a strong association
between macrophage polarization-related cytokines and postoperative AKI. Our
internally validated model, incorporating IFN-
AKI, acute kidney injury; AIC, akaike information criterion; AKIN, acute kidney injury network; BIC, Bayesian information
criterion; CPB, cardiopulmonary bypass; DCA, decision curve analysis; ICU,
intensive care unit; IDI, integrated discrimination improvement; NGAL, neutrophil
gelatinase-associated lipocalin; IGFBP-7, insulin-like growth factor binding
protein-7; TIMP-2, tissue inhibitor of metalloproteinase-2; GFR, glomerular
filtration rate; LVEF, left ventricular ejection fraction; NRI, net
reclassification improvement; ROC, receiver operator characteristic curve; AUC,
area under the curve; RRT, renal replacement therapy; IFN-
The datasets used and/or analyzed during the current study are available from the corresponding author upon reasonable request.
LQW and ZYC designed the research study. SZ participated in data collection. SZ, MJC, PFC, and DMZ analyzed the data. MJC drafted the article. All authors contributed to editorial changes in the manuscript. All authors read and approved the final manuscript. All authors have participated sufficiently in the work and agreed to be accountable for all aspects of the work.
This study was conducted after the acquisition of written informed consent from the participating patients and upon approval by the Ethics Committees of Fuwai Hospital (No. 2023-2005).
The authors wish to thank Zhongli Chen (Department of Cardiology, Fuwai Hospital, National Center for Cardiovascular Disease of China, Beijing, China) for her assistance in performing the statistical analysis for this work.
This work was supported by the National Natural Science Foundation of China (NO.: NSFC8210022135) and the Chinese Academy of Medical Sciences Innovation Fund for Medical Sciences (NO.: 2021-1-I2M-016).
The authors declare no conflict of interest.
Publisher’s Note: IMR Press stays neutral with regard to jurisdictional claims in published maps and institutional affiliations.