- Academic Editor
†These authors contributed equally.
Background: Autophagy is instrumental in various health conditions, including cancer, aging, and infections. Therefore, examining proteins and compounds associated with autophagy is paramount to understanding cellular biology and the origins of diseases, paving the way for potential therapeutic and disease prediction strategies. However, the complexity of autophagy, its intersection with other cellular pathways, and the challenges in monitoring autophagic activity make the experimental identification of these elements arduous. Methods: In this study, autophagy-related proteins and chemicals were catalogued on the basis of Human Autophagy-dedicated Database. These entities were mapped to their respective PubChem identifications (IDs) for chemicals and Ensembl IDs for proteins, yielding 563 chemicals and 779 proteins. A network comprising protein–protein, protein–chemical, and chemical–chemical interactions was probed employing the Random-Walk-with-Restart algorithm using the aforementioned proteins and chemicals as seed nodes to unearth additional autophagy-associated proteins and chemicals. Screening tests were performed to exclude proteins and chemicals with minimal autophagy associations. Results: A total of 88 inferred proteins and 50 inferred chemicals of high autophagy relevance were identified. Certain entities, such as the chemical prostaglandin E2 (PGE2), which is recognized for modulating cell death-induced inflammatory responses during pathogen invasion, and the protein G Protein Subunit Alpha I1 (GNAI1), implicated in ether lipid metabolism influencing a range of cellular processes including autophagy, were associated with autophagy. Conclusions: The discovery of novel autophagy-associated proteins and chemicals is of vital importance because it enhances the understanding of autophagy, provides potential therapeutic targets, and fosters the development of innovative therapeutic strategies and interventions.
Autophagy constitutes an essential biological process wherein cells facilitate the degradation and recycling of unnecessary or dysfunctional components via a lysosome-dependent mechanism [1]. This process functions as an adaptive response to stress, providing energy and molecular precursors while maintaining homeostasis and viability to enhance cell survival. Dysregulation in autophagy is associated with numerous diseases, including neurodegeneration, metabolic disorders, and cancers [2]. An increasing number of studies aim to identify and characterize molecular modulators capable of regulating autophagy and intervening in the pathogenesis of these diseases. However, the intricate mechanisms underpinning the regulation and execution of autophagy at the molecular level remain to be fully elucidated.
To date, four defined types of autophagy have been distinguished: macroautophagy, microautophagy, chaperone-mediated autophagy (CMA), and crinophagy. Macroautophagy, which is the most extensively researched type, involves the targeting and isolation of cytoplasmic components into a double membrane-bound vesicle known as the autophagosome, which then fuses with the lysosome, leading to the degradation and recycling of contents within the autolysosome [3, 4]. Contrastingly, microautophagy directly engulfs small cytoplasmic components into the lysosome via invagination of the lysosomal membrane or cellular protrusion [5]. CMA represents a highly complex and specific pathway wherein targeted proteins must bind to a chaperone protein, such as heat shock cognate 71 KDa protein (hsc70), to form a chaperone complex [6]. Finally, crinophagy, which is the least understood form of autophagy, involves the degradation of unnecessary secretory granules [7]. These distinct forms of autophagy operate via unique mechanisms and involve different genes and enzymes, contributing to the complex and diverse cellular functions of autophagy.
Since the discovery of the first autophagy gene through genetic screens in Saccharomyces cerevisiae, numerous genes and proteins associated with autophagy have been identified [8, 9]. As of now, over 36 proteins deemed critical for autophagy have been classified, typically denoted as autophagy-related gene (ATG) [10]. For instance, Atg8 significantly correlates with the size of the autophagosome [11], whereas Atg9 is associated with the quantity of autophagosomes [12]. Atg6 plays a role in autophagic programmed cell death, and it is linked to lymphoma, hepatocellular carcinoma, and other cancers [13]. Several studies aimed to identify the regulators of the autophagy pathway. Bernard et al. [14] identified Lysine Demethylase 4A (KDM4A/Rph1) as a transcriptional repressor of autophagy and used large-scale analysis of ATG expression to identify numerous novel autophagy regulators [15]. However, many autophagy-related molecules remain undiscovered due to limitations in experimental techniques.
Elucidating the molecular underpinnings of autophagy is pivotal for comprehending the pathogenesis of autophagy-related diseases. A growing body of research focuses on the discovery of novel modulators that regulate autophagy [16]. Several computational strategies have been employed to identify potential autophagy genes, demonstrating superior efficiency compared with experimental methods. The sequence homology-based method, which involves comparing sequences of known autophagy genes with those of other genes, is widely utilized to pinpoint potential autophagy-related genes based on sequence similarity [17, 18]. Other techniques, such as co-expression analysis and Gene Ontology (GO) function-based analysis, have proven effective in unearthing novel autophagy genes [15, 19, 20]. In the present study, a novel computational approach was introduced to effectively identify potential autophagy-related modulators on the basis of molecular interactions. The information on existing autophagy-related modulators was sourced from the Human Autophagy Modulator Database (HAMdb) [21]. By utilizing known autophagy data, numerous novel chemicals and proteins were identified through a series computational methods, grounded in the network of proteins and chemicals. This study offers a convenient methodology for identifying new autophagy modulators. The findings illuminate the molecular mechanisms of autophagy and provide potential therapeutic targets for intervening in autophagy-related diseases.
The original autophagy-associated chemicals and proteins were sourced from HAMdb [21]. Serving as a comprehensive research tool, HAMdb was designed to facilitate the study of autophagy, a pivotal cellular process responsible for the degradation and recycling of superfluous or defective cellular organelles and proteins. HAMdb encompasses 796 proteins and 841 chemicals related to autophagy through literature review. These chemicals and proteins were assigned their respective PubChem and Ensembl identifications (IDs). In detail, 796 autophagy-related proteins involved 86 cell lines and 899 diseases, and 841 related chemicals involved 82 cell lines and 10 categories of diseases. After those not present in the constructed network were excluded, 563 related chemicals and 779 related proteins were considered in this study, as enumerated in Supplementary Table 1.
Chemical and protein organization necessitated interaction data, which were procured from Search Tool for Recurring Instances of Neighboring Genes (STRING, https://cn.string-db.org/, version 11.5) [22] and Search Tool for Interactions of Chemicals (STITCH, http://stitch.embl.de/, version 4.0) [23]. Specifically, STRING provided protein–protein interaction (PPI) data, and STITCH was utilized for chemical–chemical interaction (CCI) and chemical–protein interaction (CPI) information.
The CCI data consists of two chemicals, represented by their respective PubChem IDs, and a “Combined Score” ranging from 1 to 999, which denotes the strength of the linkage between these chemicals. Given the enormous quantity of chemicals within the CCI data, the extraction was confined to interactions involving chemicals documented in Kyoto Encyclopedia of Genes and Genomes (KEGG), resulting in 419,124 CCIs involving 11,548 chemicals. Each PPI comprised two proteins, denoted by Ensembl IDs, and one “Combined Score” within the same range. Among the PPI data, 5,969,249 interactions involved 19,385 proteins. As for the CPI data, each CPI included one chemical (PubChem ID), one protein (Ensembl ID), and a “Combined Score” also ranging between 1 and 999. The extraction was limited to the CPIs between the aforementioned 11,548 chemicals and 19,385 proteins, yielding a total of 333,440 CPIs.
One large heterogeneous network was constructed with the CCIs, PPIs and CPIs.
Such network defined the above chemicals and proteins as nodes, whereas CCIs,
PPIs and CPIs determined the edges of this network. The final network contained
30,933 (11,548 + 19,385) nodes and 6,721,813 (419,124 + 5,969,249 + 333,440)
edges. Furthermore, each edge was assigned a weight, which was defined as the
“Combined score” of the corresponding interaction. For convenience, such
network was denoted by
RWR is a graph-based algorithm used to rank nodes within a network [24, 25, 26, 27, 28, 29, 30]. It
operates by initiating a random walk on the graph, coupled with a “restart”
probability at each step that allows the walker to return to the initial node.
This “restart” probability serves as an indicator of the initial node’s
importance, and the algorithm uses it to assign a score to every node in the
network. In a given network with
where
The network’s architecture can significantly affect the outcome when applying the RWR algorithm for identifying critical nodes. A limited group of nodes may receive high probabilities due to their unique positioning within the network, regardless of their correlation with autophagy. Therefore, a permutation test was conducted to determine the statistical significance and verify the relevance of each preliminary candidate chemical and protein. The results generated 1000 sets of chemicals and proteins, each of which contained the same quantity of chemicals and proteins related to autophagy in the seed nodes for the RWR algorithm. Consequently, a probability for each candidate chemical and protein within each of the 1000 random sets was computed. This methodology allowed a comparison between the probabilities of the actual seed nodes and those of the randomly generated sets, thus assisting in determining the statistical significance of each probability. The Z-score was used as the metric for evaluating significance as follows.
where
A double interaction test was conducted to obtain most important candidate
chemicals. Such test adopted the CCI and CPI information mentioned in Section
2.2. For a CCI containing two chemicals
Evidently, a candidate chemical with high MISs meant it can be related to autophagy with a high probability. Considering that 900 is a crucial cutoff of highest confidence for CCIs and CPIs, it was set as the threshold for two MISs, i.e., chemicals with both MISs no less than 900 were chosen for further investigations.
Similar to the interaction tests for chemicals, interaction tests were designed
for proteins. This test was based on PPIs and CPIs, also named double interaction
test. For a PPI containing two proteins
With the same argument, 900 was set as the threshold of the above two MISs to access most important proteins.
The final test aimed to filter candidate genes in accordance with their functional term alignments with genes already confirmed to associate with autophagy. If a candidate gene’s functional terms closely mirror those of a validated autophagy-related gene, this considerably increases the probability that the candidate gene also has a connection to autophagy [31, 32, 33]. The enrichment score (ES) was calculated using the following formula to assess the relationship between a gene and a GO term or a KEGG pathway:
where
For each candidate gene
A threshold of 0.98 was established for the MES to isolate significant candidate genes, suggestive of a potential association with autophagy.
In this study, a random walk-based method was designed to identify autophagy-associated proteins and chemicals. The RWR algorithm was in charge of identifying latent proteins or chemicals from the heterogeneous network on the basis of currently known autophagy-associated proteins and chemicals. The latent proteins and chemicals were filtered by three screening tests, including permutation, interaction, and enrichment tests. The false positive proteins and chemicals can be controlled by permutation test, whereas the other screening tests can help select most essential proteins and chemicals that have strong associations with validated ones. Through the above statistical analysis, the remained proteins and chemicals were deemed to have strong associations with autophagy.
This study introduces a method that employs a random walk-based strategy to detect potential novel proteins and chemicals related to autophagy. Fig. 1 provides a graphical depiction of the entire procedure.
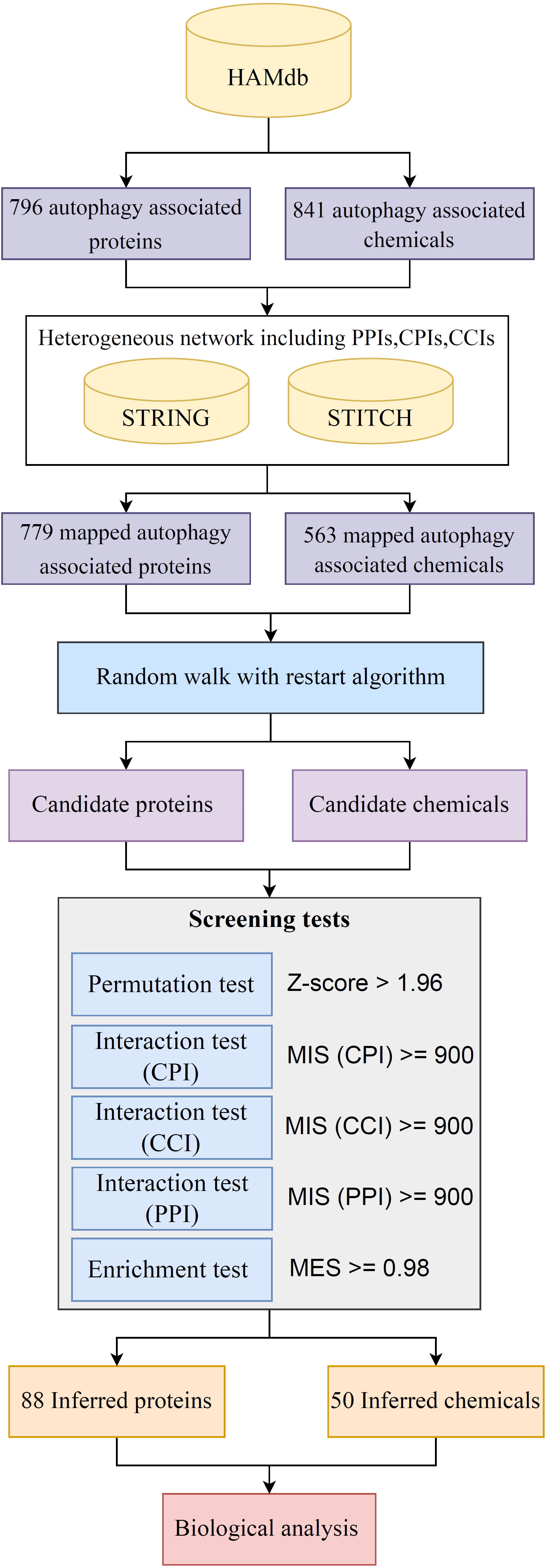
Flowchart of autophagy-associated chemicals and proteins mining process. The comprehensive strategy for identifying potential proteins and chemicals related to autophagy involved multiple steps: (1) extraction of validated autophagy associated chemicals and proteins from Human Autophagy Modulator Database (HAMdb); (2) construction of a heterogeneous network incorporating protein–protein interactions (PPIs), chemical–protein interactions (CPIs), and chemical–chemical interactions (CCIs); (3) application of the random-walk-with-restart (RWR) algorithm to this network for the extraction of high-probability candidate proteins and chemicals; (4) conducting screening tests to refine these candidates, thereby finalizing the inferred proteins and chemicals; and (5) analysis of the biological correlation among the selected proteins, chemicals, and the disease, as confirmed by literature. MIS, maximum interaction score; MES, maximum enrichment score.
The developed network underwent analysis via the RWR algorithm, using autophagy-related proteins and chemicals as seed nodes. Each node within the network received a probability value reflecting its correlation with the seed nodes. Supplementary Tables 2,3 present the results from the RWR search conducted on the network, built around seed proteins and chemicals, in conjunction with the outcomes from the screening tests.
The results for proteins within the developed network, derived using autophagy-related proteins and chemicals, are presented below. The permutation test computed a Z-score for each protein in the network, as shown in Supplementary Table 2. First, proteins exhibiting a Z-score exceeding 1.96 were deemed significant, leading to the detection of 2253 proteins. Subsequently, an interaction test was performed on these proteins, attributing the MIS of PPI and the MIS of CPI to each protein, as documented in Supplementary Table 2. From these proteins, 668 displayed a MIS for PPI and CPI surpassing 900 and were thus chosen. Lastly, enrichment test was applied to examine the proteins acquired post-filtration. The MES of each protein was computed, setting a threshold at 0.98. Any protein whose MES fell beneath this threshold was removed from the protein list, retaining 88 proteins, which are presented in Supplementary Table 2.
The results for chemicals within the curated network, based on autophagy-associated proteins and chemicals, are presented below. A permutation test was conducted for each chemical in the network, generating a Z-score compiled in Supplementary Table 3. Initially, chemicals demonstrating a Z-score exceeding 1.96 were deemed noteworthy, leading to the identification of 425 chemicals. Afterwards, an interaction test was performed on these chemicals, attributing the MIS of CCI and the MIS of CPI to each chemical, as outlined in Supplementary Table 3. Of these chemicals, 50 showcased an MIS for CCI and CPI surpassing 900 and were thus selected.
In the subsequent analyses, the 88 identified proteins and 50 identified chemicals were referred to as inferred proteins and inferred chemicals, respectively, hypothesizing their significant association with autophagy.
Multiple analyses were conducted to assess the credibility of the inferred proteins and chemicals. The quantity of interacting proteins and chemicals linked to autophagy was determined for each inferred protein and chemical, adhering to a minimum confidence score of 900. These results were visualized as box plots, with both distributions illustrated in Fig. 2. Detailed information about interacting proteins and chemicals, along with their respective quantities, is provided in Supplementary Table 4.
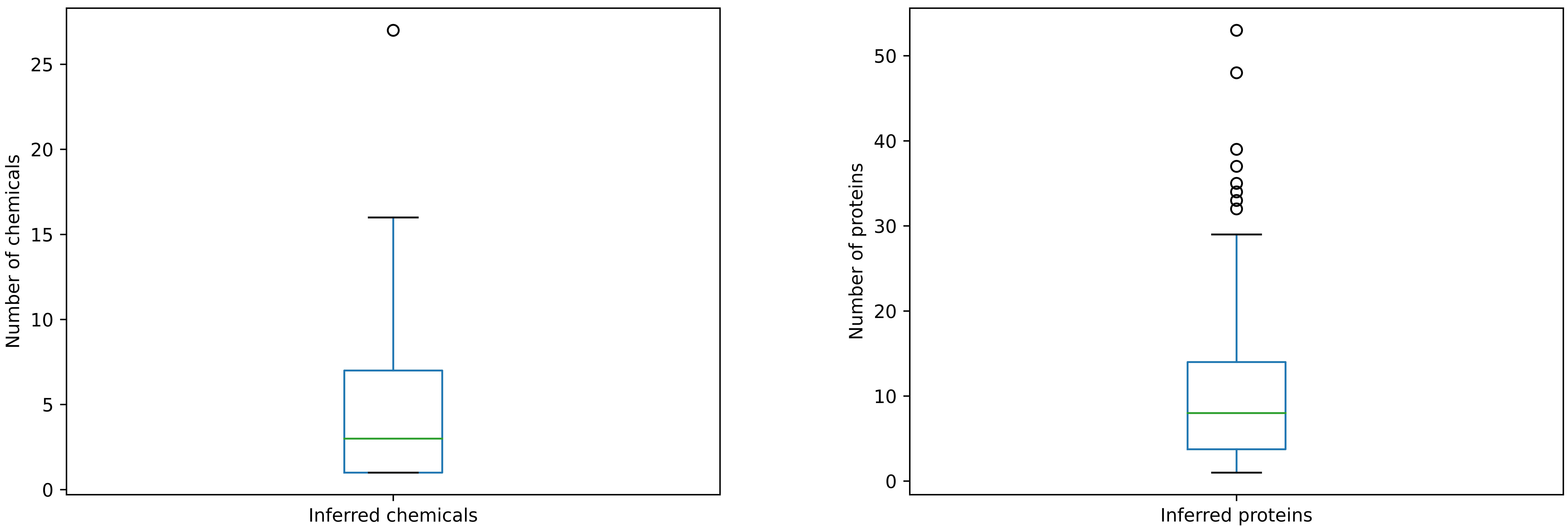
Box plot demonstrating the number of interactions between autophagy-associated proteins and chemicals with inferred proteins and chemicals. A significant number of inferred proteins and chemicals exhibited interactions with autophagy-associated entities, i.e., approximately 12 proteins and five chemicals, respectively, with all interactions supported by confidence scores exceeding 900. These results highlight a strong association between the identified proteins/chemicals and autophagy.
Several inferred proteins demonstrated interactions with more than 10 proteins associated with autophagy, all of which had confidence scores exceeding 900. On average, each inferred protein interacted with approximately 12 proteins related to autophagy, with each interaction bearing high confidence scores. Similarly, many inferred chemicals revealed interactions with over five chemicals associated with autophagy, all with confidence scores surpassing 900. On average, each inferred chemical interacted with around five chemicals linked to autophagy, with each interaction substantiated by high confidence scores. These results imply a robust association between the identified proteins/chemicals and autophagy.
Dysregulation of autophagy is implicated in a myriad of human diseases,
including immune disorders, neurodegeneration, and cancer. The exploration of
novel molecules that can modulate autophagy continues to be a significant focus
for developing disease interventions. The induction and regulation of autophagy
involve various cellular signaling pathways. For example, MTOR kinase complex 1
(MTORC1) senses extra- or intracellular stresses, including nutrient starvation
condition in mammalian cells, and dissociates from the complex and ATG13 and
ULK1/2, leading to the dephosphorylated of ATG13 and formation of the
ATG1-ATG13-ATG17 complex and ultimately induces the macroautophagy [34].
Autophagy activity is regulated by cAMP-dependent protein kinase A (PKA) and TOR
pathways involving major kinases, including AMPK; MTORC1; and proteins TSC1/2,
LC3, and CAMKK2/CaMKK
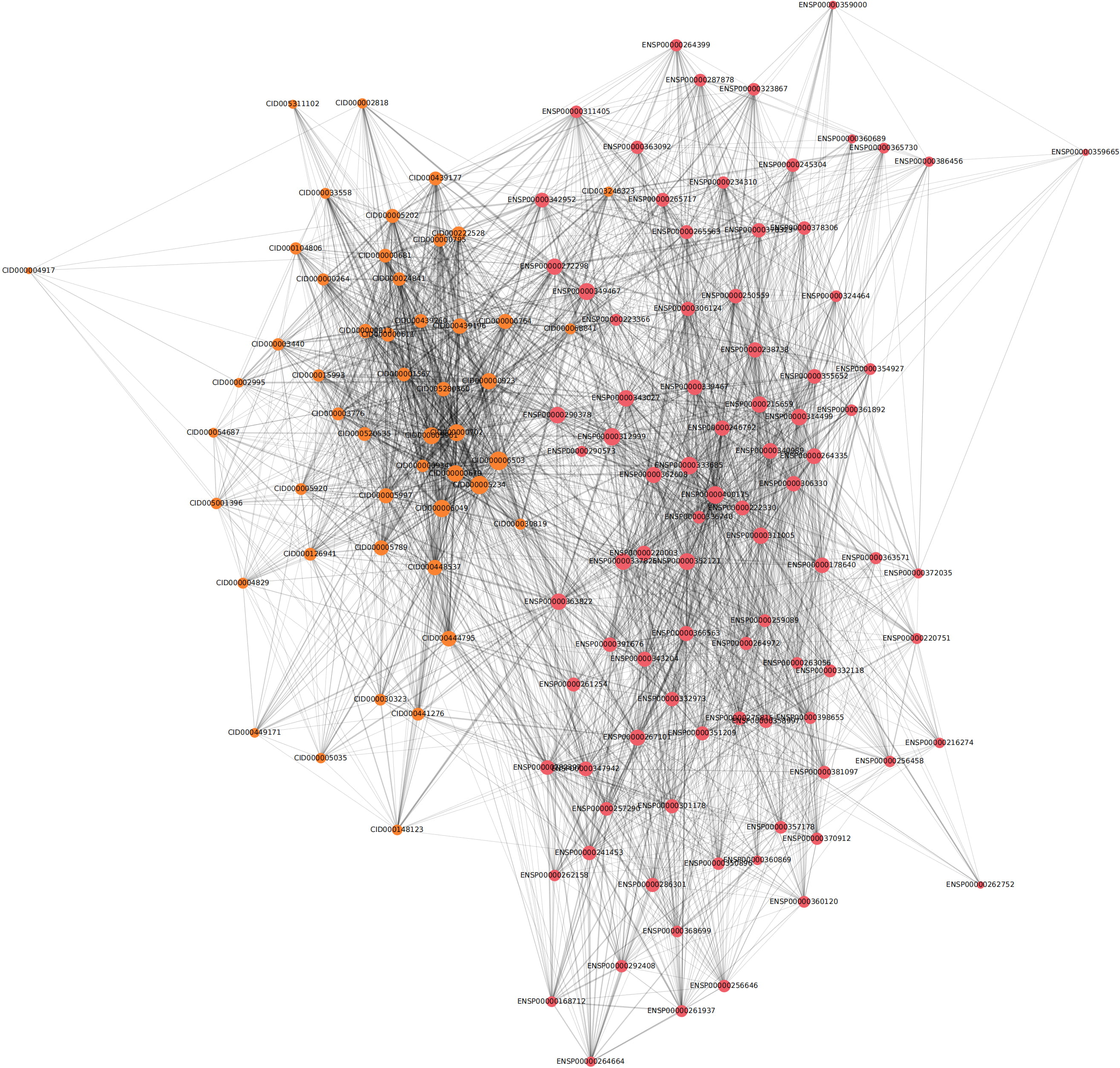
Network diagram of 88 inferred proteins and 50 inferred chemicals. The size of each node in the network corresponds to its degree, with a larger node size indicating a higher degree. The color intensity of edges linking nodes in the diagram amplifies as the combined score between the nodes increases. Several significant nodes demonstrate a higher level of connectivity and a combined score than others.
Prostaglandin E2 (PGE2), also known as dinoprostone, emerged as a potential autophagy modulator through the computational analysis. In this study, PGE2 achieved a significant Z-score of 3.31 and an MIS of 999, interacting with nine validated chemicals. PGE2 is an FDA-approved medication primarily used for labor induction or uterine content evacuation [38]. A growing body of evidence underscores the crucial role of PGE2 in modulating cell death-induced inflammatory responses during pathogenic infection [39, 40]. A recent study elucidated a unique autophagic cell death mechanism triggered by the activation of PGE2 production, corroborating the functional role of PGE2 in autophagy [41]. The relationship between PGE2 and autophagy in hepatocellular carcinoma has been substantiated through in-vitro experiments. Gao et al. [42] discovered that the PDK1 inhibitor, implicated in PGE2-induced pathways, demonstrated autophagy-inducing activity along with reactive oxygen species (ROS) accumulation. Moreover, PGE2 has been found to foster the autophagy of monocytes and neutrophils during Mycobacterium tuberculosis infection, leading to immunosuppressive effects in inflammatory immune responses [43]. These findings indicate the potential role of PGE2 as an autophagy-related modulator and validated the credibility of the results.
Pioglitazone was identified as a potential autophagy-related modulator, achieving a significant Z-score of 7.71 and an MIS of 997, and it interacted with a validated chemical entity. This compound is employed as an antidiabetic drug that aids in the regulation of blood glucose levels in patients with type 2 diabetes. Emerging evidence underscores the considerable effect of pioglitazone on autophagy. The administration of pioglitazone has been shown to exert a protective effect against renal cell hypoxia/reoxygenation by inducing autophagy via the AMPK-mTOR signaling pathway [44]. Pioglitazone has also been found to attenuate hepatic steatosis by augmenting autophagy and cytosolic lipolysis in hepatic cells [45]. Conversely, Lin et al. [46] illustrated that pioglitazone can intervene in the autophagy process triggered by a high fructose diet, thus normalizing autonomic abnormalities. These observations suggest that pioglitazone may exhibit a dual function: it can either promote or inhibit autophagy depending on physiological conditions. Nevertheless, these findings offer substantial evidence to endorse the role of pioglitazone as an autophagy-related modulator.
Cholesterol was found to play a role in autophagy-related activities. It achieved a significant Z-score of 2.0 and an MIS of 996, and it was found to interact with five validated chemical entities. Cholesterol, which is ubiquitously present in all human cells, performs fundamental functions in biological processes. Cellular cholesterol depletion leads to the macroautophagy of human fibroblasts, as evidenced by an increase in LC3-II [47]. A study affirmed the connection between cholesterol homeostasis and autophagy, suggesting that these vital biological processes share mutual regulatory elements [48]. Autophagy is hypothesized to play a protective role in regulating cholesterol levels and sustaining normal lipid metabolism. Meanwhile, excessive cholesterol may activate autophagy as a cellular defense mechanism to mitigate organellar stress [49]. These observations underscore the importance of cholesterol in modulating autophagy and validated the prediction of the present study.
Serotonin, a monoamine neurotransmitter synthesized by nerve cells, plays an
instrumental role in regulating various bodily functions such as sleep,
digestion, and healing. This study posited that serotonin could be a potential
autophagy-related modulator. It showed a significant Z-score of 2.5 and an MIS of
994, engaging in interactions with 16 validated chemical entities. Serotonin
neurons have been reported to initiate autophagic activity in response to the
toxicity of 3,4-methylenedioxymethamphetamine [50]. Besides nerve cells, an
association has been demonstrated between peripheral serotonin and autophagy in
liver cells of a mouse model. Serotonin appears to regulate steatosis in liver
cancer cells, and it may enhance liver tumorigenesis through the induction of
autophagy [51]. Soll et al. [52] documented serotonin’s tumor-promoting
effect in hepatocellular cancer through autophagy regulation. The activation of
serotonin signaling has been shown to induce autophagy in ER
The protein G Protein Subunit Alpha I1 (GNAI1) (ENSP00000343027) was identified
as a strong indicator of autophagy activity. With a significant Z-score of 2.35
and an MIS of 986, GNAI1 demonstrated interactions with 25 validated proteins.
Predominantly expressed in immune cells, GNAI1 acts as a downstream transducer in
numerous signaling cascades for G protein-coupled receptors. A recent study
discovered that the upregulation of GNAI1 and RUNX Family Transcription Factor 2 (RUNX2) coincides with autophagy
activation, which may enhance T cell survival and promote T-cell
leukemia/lymphoma [54]. In the context of breast cancer, GNAI1 plays a
significant role in ether lipid metabolism and influences various cellular
processes, including proliferation and autophagy [55]. A number of studies
implicated G protein-coupled signaling in autophagy regulation. For instance, the
rate of autophagic flux was found to be correlated with G-protein inhibitory
protein in a colon cancer cell line [56]. Other signaling proteins, such as
G
The serine/threonine-protein kinase pim-1 (PIM1, ENSP00000362608), which is associated with cell proliferation, exhibits oncogenic activity in tumorigenesis. It showed a significant Z-score of 8.58 and an MIS of 973 and demonstrated interactions with 11 validated proteins. Accumulating evidence suggests that PIM1 influences cell cycle progression by modulating autophagy. The overexpression of PIM1 can circumvent cell apoptosis by enhancing autophagy via the activation of the AMPK/mTOR pathway in cardiomyocytes in response to oxidative stress [58]. Conversely, PIM1 inhibition disrupts autophagy-related pathways, leading to the apoptosis of glioblastoma cells [59]. Wu et al. [60] highlighted the connection between hispidulin-induced autophagy and PIM1 downregulation in podocytes of patients with nephropathy. These findings reveal a novel role for PIM1 as an autophagy-related modulator.
RIPK2 encodes a receptor-interacting protein kinase (ENSP00000220751),
instrumental in activating the NF-
The protein kinase C (PKC) epsilon type (ENSP00000306124), encoded by the PRKCE gene, emerged as a potential autophagy-related modulator. PRKCE achieved a significant Z-score of 6.3 and an MIS of 964 and demonstrated interactions with 11 validated proteins. PKC plays an integral role in regulating a myriad of biological processes, notably participating in cancer cell proliferation and apoptosis. Toton et al. [65] recently observed PKC’s influence on the autophagy of glioblastoma cells and found that a loss of PKC led to a downregulation in the expression of autophagic signals, thus presenting a potential therapeutic effect for glioblastoma. Additional studies elucidated that the Fas apoptotic inhibitory molecule regulates macroautophagy through the modulation of the mTOR pathway and PKC-mediated phosphorylation in lung adenocarcinoma [66]. In hypoxic conditions, PKC interacts with protein tyrosine phosphatases-PTPN12 and triggers endothelial autophagy via AMPK activation to foster angiogenesis [67]. PRKCE is indeed an autophagy-related modulator.
In this study, the RWR algorithm was used to probe a network comprising PPI, CPI, and CCI by using autophagy-associated proteins and chemicals as seed nodes. The proteins and chemicals underwent further scrutiny via screening tests, resulting in the identification of 50 chemicals and 88 proteins with a pronounced correlation to autophagy. Several representative chemicals and proteins were substantiated through literature review, and compelling evidence was unearthed to endorse the findings. These newly discovered autophagy-related molecules provide a theoretical foundation to advance autophagy research and contribute to the discovery of new therapeutic targets for diseases related to autophagy.
CMA, chaperone-mediated autophagy; ATG, autophagy-related gene; HAMdb, Human Autophagy Modulator Database; ID, identification; STRING, search tool for recurring instances of neighbouring genes; STITCH, search tool for interactions of chemicals; CCI, chemical-chemical interaction; CPI, chemical-protein interaction; PPI, protein-protein interaction; KEGG, Kyoto Encyclopedia of Genes and Genomes; RWR, Random Walk with Restart; MIS, maximum interaction score; GO, Gene Ontology; ES, enrichment score; MES, maximum enrichment score.
The datasets analyzed during the current study are available in the Human Autophagy Modulator Database (http://hamdb.scbdd.com/).
TH and YDC designed the research study. FMH, WG, LC and KYF performed the research. FMH and WG analyzed the data. FMH and WG wrote the manuscript. All authors contributed to editorial changes in the manuscript. All authors read and approved the final manuscript. All authors have participated sufficiently in the work to take public responsibility for appropriate portions of the content and agreed to be accountable for all aspects of the work in ensuring that questions related to its accuracy or integrity.
Not applicable.
Not applicable.
This research was funded by the Strate-gic Priority Research Program of Chinese Academy of Sciences [XDB38050200, XDB38040202], National Key R&D Program of China [2022YFF1203202], the Fund of the Key Laboratory of Tissue Microenvironment and Tumor of Chinese Academy of Sciences [202002], Shandong Provincial Natural Science Foundation [ZR2022MC072].
The authors declare no conflict of interest. Given their role as Guest Editor or Editorial Board Members, Dr. Tao Huang and Dr. Yu-Dong Cai had no involvement in the peer review of this article and had no access to information regarding its peer review. Full responsibility for the editorial process for this article was delegated to Kavindra Kumar Kesari.
Publisher’s Note: IMR Press stays neutral with regard to jurisdictional claims in published maps and institutional affiliations.